Symptom-BERT: Enhancing Cancer Symptom Detection in EHR Clinical Notes
Journal of Pain and Symptom Management(2024)
摘要
Context
Extracting cancer symptom documentation allows clinicians to develop highly individualized symptom prediction algorithms to deliver symptom management care. Leveraging advanced language models to detect symptom data in clinical narratives can significantly enhance this process.
Objective
This study uses a pre-trained large language model to detect and extract cancer symptoms in clinical notes.
Methods
We developed a pre-trained language model to identify cancer symptoms in clinical notes based on a clinical corpus from the Enterprise Data Warehouse for Research at a healthcare system in the Midwestern United States. This study was conducted in 4 phases:1 pre-training a Bio-Clinical BERT model on 1 million unlabeled clinical documents,2 fine-tuning Symptom-BERT for detecting 13 cancer symptom groups within 1112 annotated clinical notes,3 generating 180 synthetic clinical notes using ChatGPT-4 for external validation, and4 comparing the internal and external performance of Symptom-BERT against a non-pre-trained version and six other BERT implementations.
Results
The Symptom-BERT model effectively detected cancer symptoms in clinical notes. It achieved results with a micro-averaged F1-score of 0.933, an AUC of 0.929 internally, and 0.831 and 0.834 externally. Our analysis shows that physical symptoms, like Pruritus, are typically identified with higher performance than psychological symptoms, such as Anxiety.
Conclusion
This study underscores the transformative potential of specialized pre-training on domain-specific data in boosting the performance of language models for medical applications. The Symptom-BERT model's exceptional efficacy in detecting cancer symptoms heralds a groundbreaking stride in patient-centered AI technologies, offering a promising path to elevate symptom management and cultivate superior patient self-care outcomes.
更多查看译文
关键词
Natural language processing,large language Model,Multiclassification,Cancer symptoms
AI 理解论文
溯源树
样例
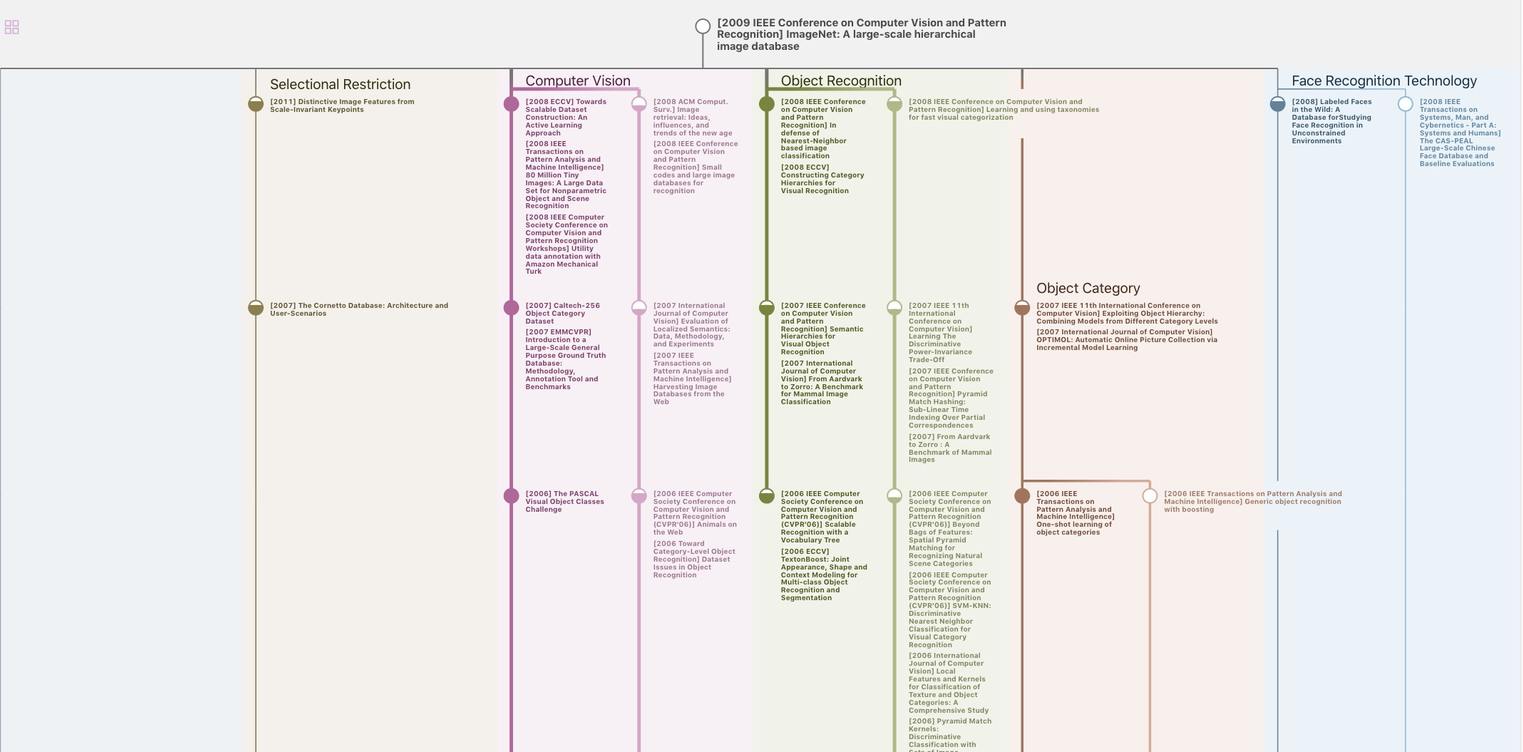
生成溯源树,研究论文发展脉络
Chat Paper
正在生成论文摘要