View-interactive attention information alignment-guided fusion for incomplete multi-view clustering
Expert Systems with Applications(2024)
Abstract
Multi-view data from real applications is usually incomplete, leading to unsatisfactory performance in clustering tasks. Existing incomplete multi-view clustering (IMVC) methods still encounter the following issues: (1) They focus on learning consistency features between different views but often struggle to recover missing data based on the complementary features between views. (2) Differing from complete multi-view data, the misaligned characteristics of incomplete multi-view data may introduce noises in feature learning, thereby affecting the performance of the IMVC models. To address these issues, we propose a novel view-interactive attention information alignment-guided fusion (VAIAF) method for clustering incomplete multi-view data. Firstly, it utilizes an autoencoder to extract feature information from each view. Then we construct an information interactive attention (IIA) module to learn multi-view information by introducing the self-attention mechanism. This module not only extracts consistent features from different views but also utilizes the feature complementary relationships between views to recover missing data to a certain extent. Therefore, it can enhance the capability of the model to learn the consistency and complementarity of incomplete multi-view data. The proposed VAIAF method also constructs a discriminator for each view to facilitate missing data recovery. In the feature fusion stage, we implement an alignment-guided strategy to prevent noise caused by misaligned feature fusion. Extensive experiments on four incomplete multi-view datasets demonstrate the effectiveness of the proposed VAIAF method in clustering tasks. The source code of this work will be released later.
MoreTranslated text
Key words
Incomplete multi-view clustering,Consistency,Complementarity,Information interaction attention,Adversarial recover,Alignment-guided fusion
AI Read Science
Must-Reading Tree
Example
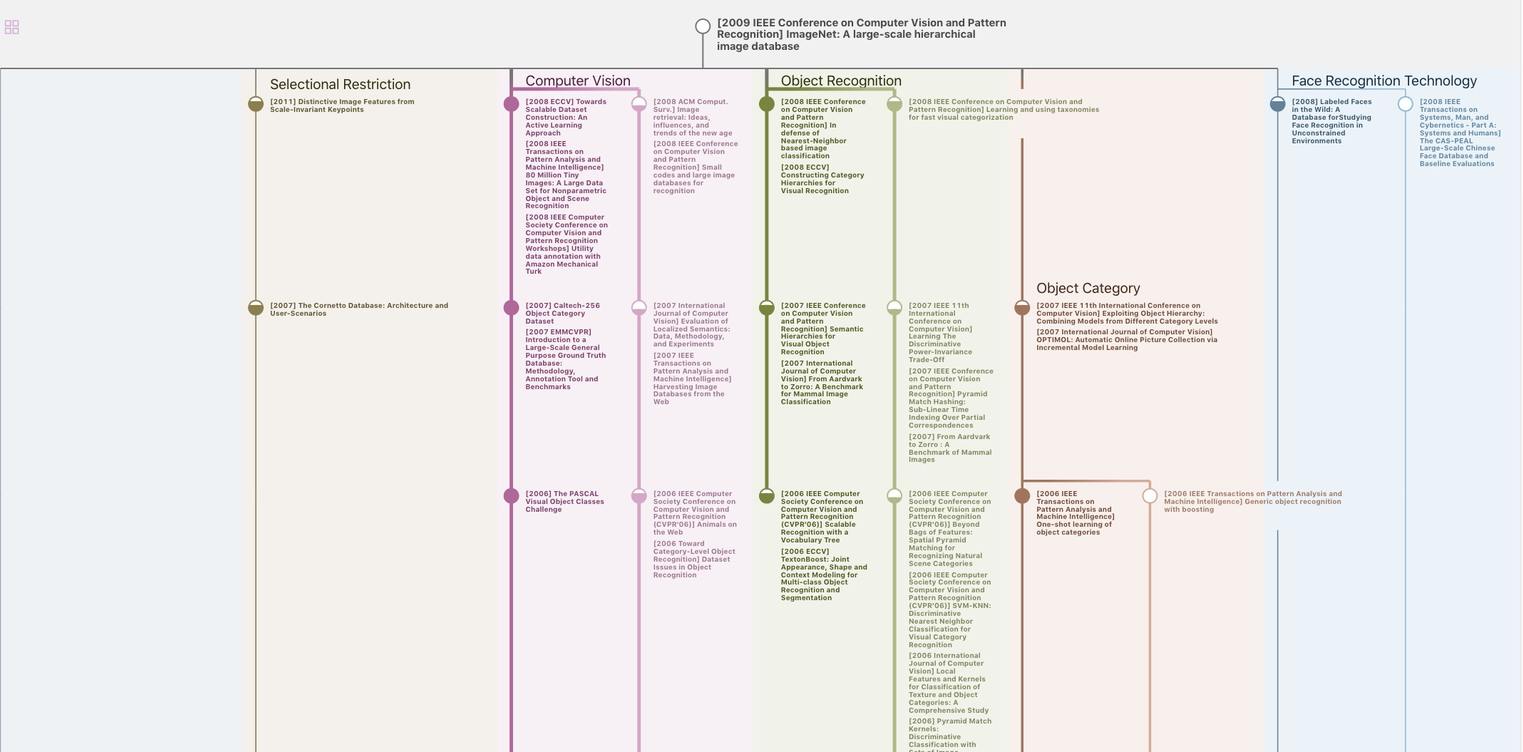
Generate MRT to find the research sequence of this paper
Chat Paper
Summary is being generated by the instructions you defined