Generating Realistic Data through Modeling and Parametric Probability for the Numerical Evaluation of Data Processing Algorithms in Two-dimensional Chromatography
Analytica Chimica Acta(2024)
摘要
Background
Comprehensive two-dimensional chromatography generates complex data sets, and numerous baseline correction and noise removal algorithms have been proposed in the past decade to address this challenge. However, evaluating their performance objectively is currently not possible due to a lack of objective data.
Result
To tackle this issue, we introduce a versatile platform that models and reconstructs single-trace two-dimensional chromatography data, preserving peak parameters. This approach balances real experimental data with synthetic data for precise comparisons. We achieve this by employing a Skewed Lorentz-Normal model to represent each peak and creating probability distributions for relevant parameter sampling. The model's performance has been showcased through its application to two-dimensional gas chromatography data where it has created a data set with 458 peaks with an RMSE of 0.0048 or lower and minimal residuals compared to the original data. Additionally, the same process has been shown in liquid chromatography data.
Significance
Data analysis is an integral component of any analytical method. The development of new data processing strategies is of paramount importance to tackle the complex signals generated by state-of-the-art separation technology. Through the use of probability distributions, quantitative assessment of algorithm performance of new algorithms is now possible. Therefore, creating new opportunities for faster, more accurate, and simpler data analysis development.
更多查看译文
关键词
two-dimensional chromatography,peak detection,peak modeling,data synthesis
AI 理解论文
溯源树
样例
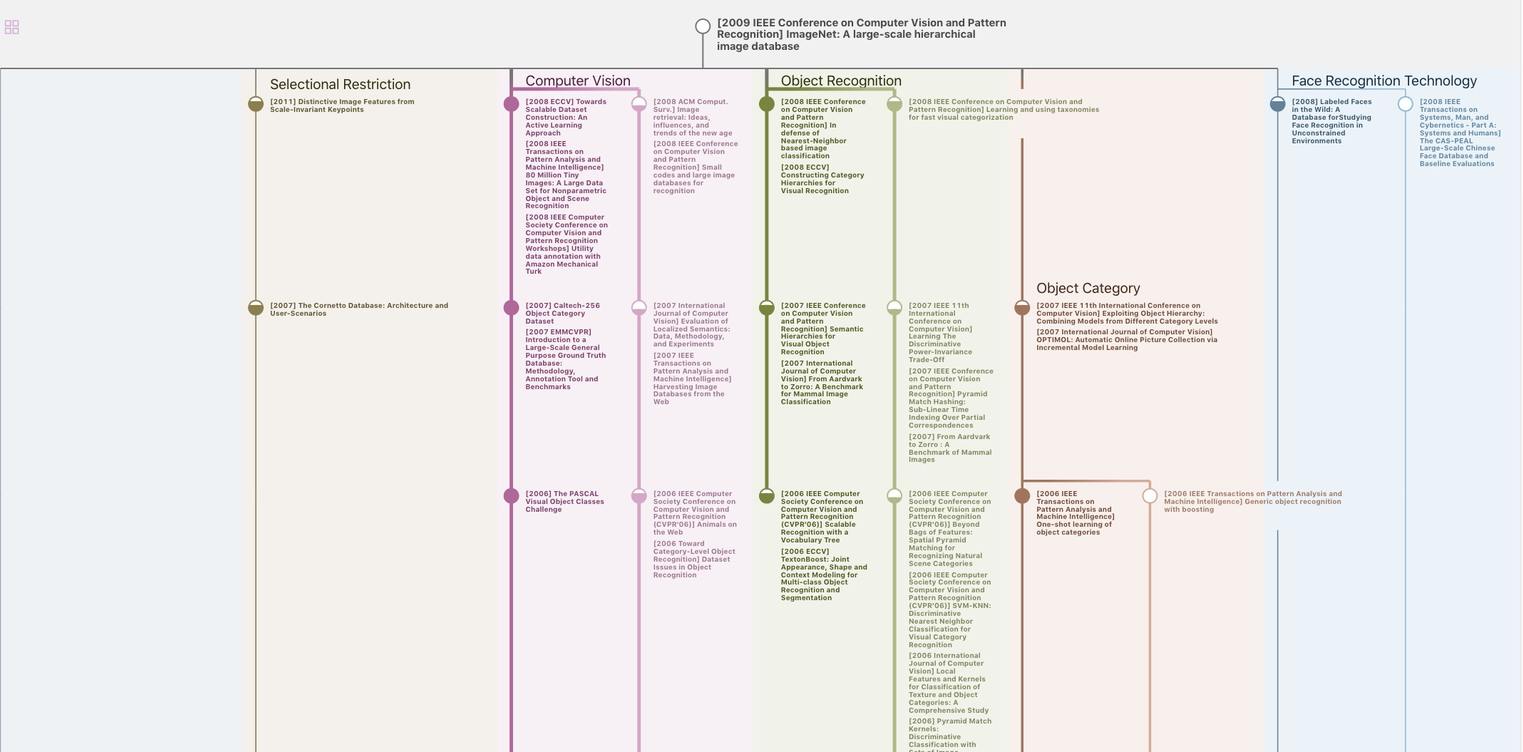
生成溯源树,研究论文发展脉络
Chat Paper
正在生成论文摘要