Machine learning allows for large-scale habitat prediction of a wide-ranging carnivore across diverse ecoregions
Landscape Ecology(2024)
Abstract
Resource selection functions are powerful tools for predicting habitat selection of animals. Recently, machine-learning methods such as random forest have gained popularity for predicting habitat selection due to their flexibility and strong predictive performance. We tested two methods for predicting continental-scale, second-order habitat selection of a wide-ranging large carnivore, the mountain lion (Puma concolor), to support continent-wide conservation management, including estimating abundance, and to predict habitat suitability for recolonizing or reintroduced animals. We compared a generalized linear model (GLM) and a random forest model using GPS location data from 476 individuals across 20 study sites in the western USA and Canada and remotely-sensed landscape data. We internally validated models and examined their ability to correctly classify used and available points by calculating area under the receiver operating characteristics (AUC). We performed leave-one-out (LOO) out-of-sample tests of predictive strength on both models. Both models suggested that mountain lions select for steeper slopes, areas closer to water, and with higher normalized difference vegetation index (NDVI), and against variables associated with human impact. The random forest model (AUC = 0.94) demonstrated that mountain lion habitat can be accurately predicted at continental scales, outperforming the traditional GLM model (AUC = 0.68). Our LOO validation provided similar results (x̄ = 0.93 for the random forest and x̄ = 0.65 for the GLM). We found that the added flexibility of the random forest model provided deeper insights into how individual covariates impacted habitat selection across diverse ecosystems. Our LOO analyses suggested that our model can predict mountain lion habitat selection in unoccupied areas or where local data are unavailable. Our model thus provides a tool to support discussions and analyses relevant to continent-wide mountain lion conservation and management including estimating metapopulation abundance.
MoreTranslated text
Key words
Conservation,Habitat suitability,Puma concolor,Random forest,Machine learning,Resource selection function
AI Read Science
Must-Reading Tree
Example
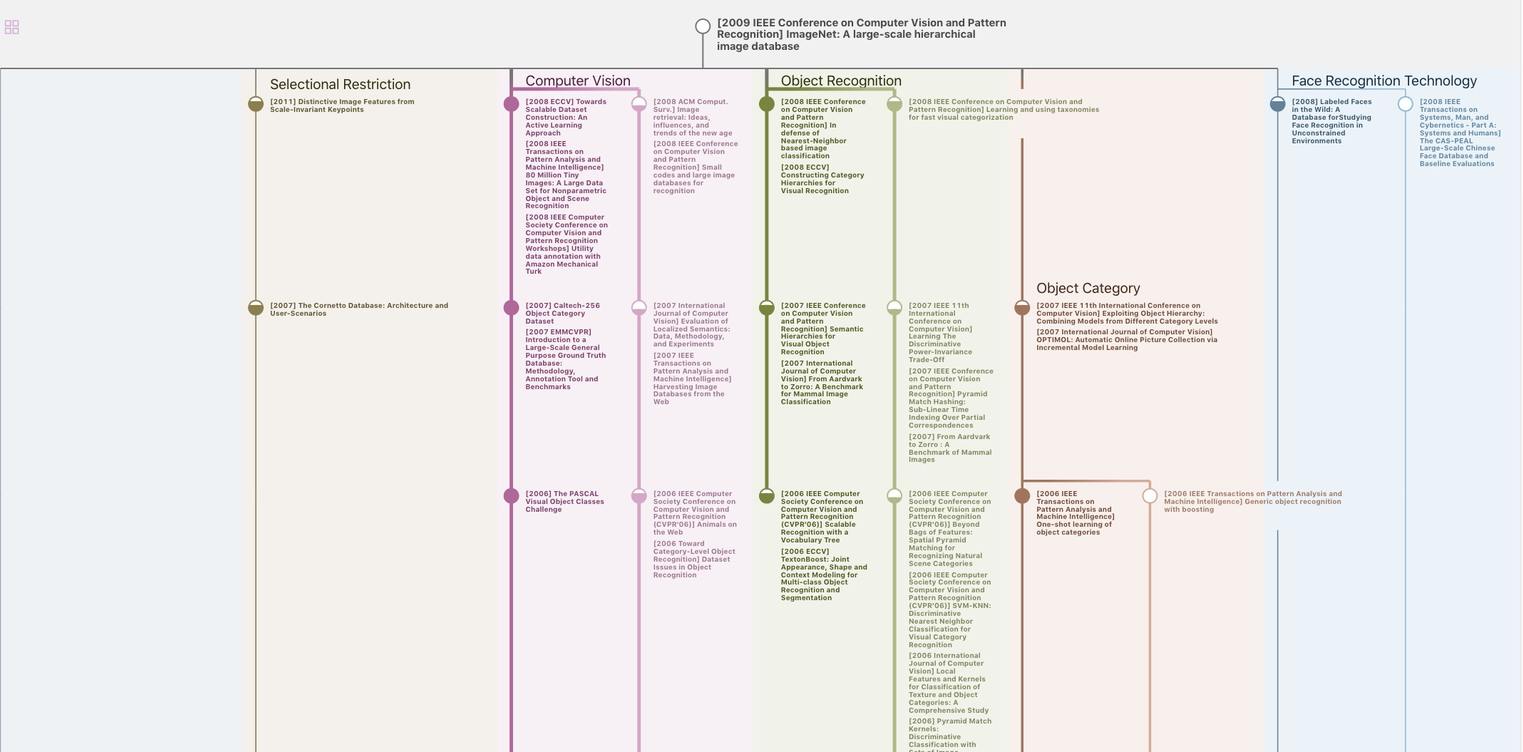
Generate MRT to find the research sequence of this paper
Chat Paper
Summary is being generated by the instructions you defined