Few-Shot Incremental Object Detection in Aerial Imagery via Dual-Frequency Prompt
IEEE TRANSACTIONS ON GEOSCIENCE AND REMOTE SENSING(2024)
摘要
Recently, there has been a growing interest in few-shot incremental object detection (FSIOD). It learns new tasks with limited data while mitigating catastrophic forgetting on previous tasks. However, existing FSIOD methods experience parameter changes after training on new tasks, causing a parameter competition issue among tasks. In addition, the background information differs among various tasks, and using a common background weight for all tasks results in the background shift. Constrained by these two issues, existing methods only alleviate catastrophic forgetting and cannot wholly prevent the performance decline on previous tasks. Especially for complex remote sensing images with messy background, the models trained on new tasks exhibit noticeable performance drops on previous tasks. In this article, we propose a novel FSIOD method via dual-frequency prompt to address these challenges, named FSIOD-DFP. It can completely eliminate catastrophic forgetting while mitigating overfitting. Specifically, a dual-frequency prompt generator is designed to tackle the parameter competition issue. It decouples the frequency components of images to produce prompts that modify the images to adapt to the base model trained on the previous tasks. Compared to traditional prompts, our generator introduces fewer parameters to address overfitting for limited data and allows freezing the base model to maintain the performance of previous data. Besides, a self-regularization loss is introduced to guide the prompt-modified images to leverage the knowledge of the base model effectively. Furthermore, we propose a task-decoupled detection head to address the background shift problem. It separates the detection heads for new and previous tasks to resolve the conflict in the background between different tasks. In FSIOD-DFP, only a prompt generator and a novel detection head are added and fine-tuned when learning a new task. Experiments on three remote sensing object detection datasets demonstrate that our method achieves state-of-the-art performance on both new and previous tasks in all few-shot incremental settings.
更多查看译文
关键词
Task analysis,Data models,Remote sensing,Training,Head,Object detection,Adaptation models,Dual-frequency prompt,few-shot incremental learning (FSIL),object detection,remote sensing images
AI 理解论文
溯源树
样例
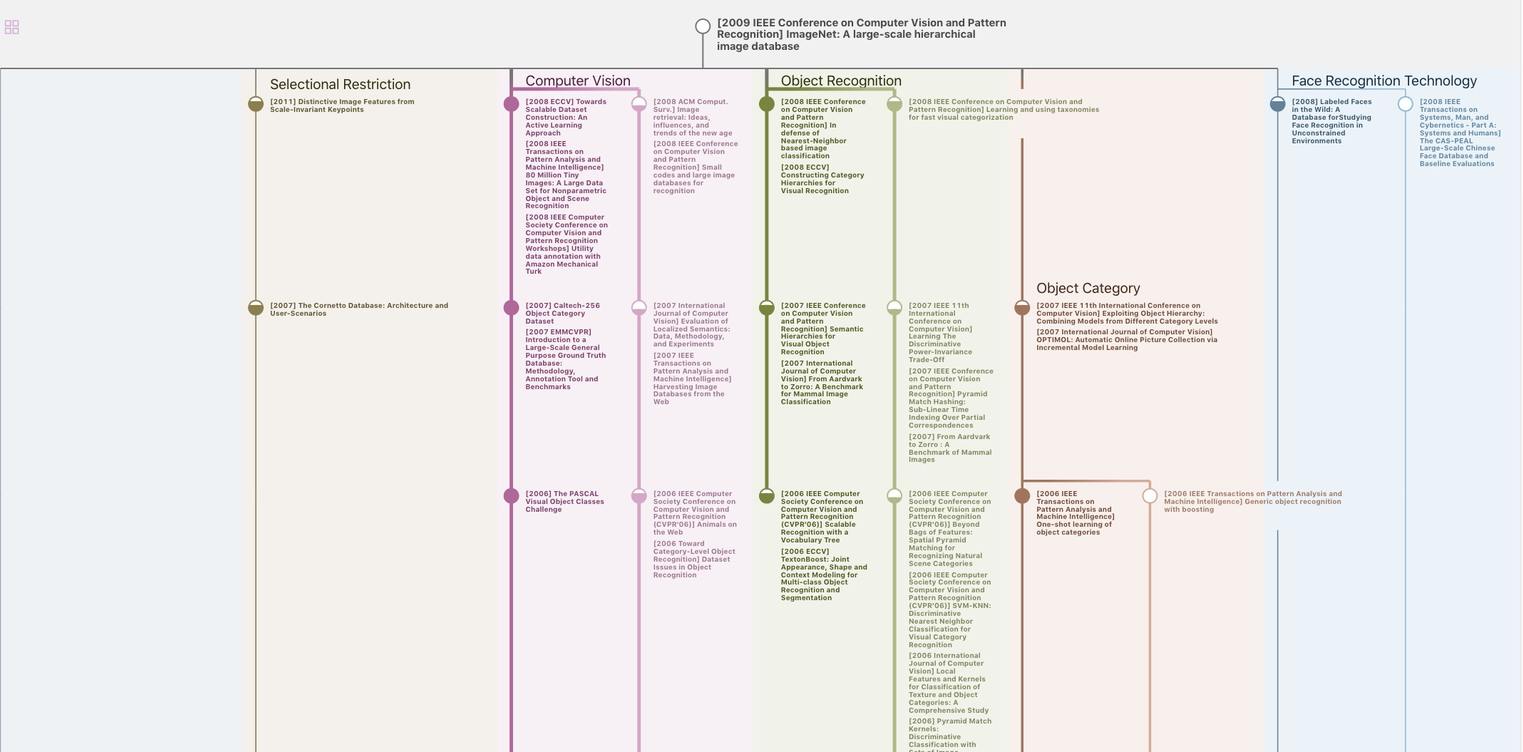
生成溯源树,研究论文发展脉络
Chat Paper
正在生成论文摘要