Distributions and bootstrap for data-based stochastic programming
Computational Management Science(2024)
Abstract
In the context of optimization under uncertainty, we consider various combinations of distribution estimation and resampling (bootstrap and bagging) for obtaining samples used to estimate a confidence interval for an optimality gap. This paper makes three experimental contributions to on-going research in data driven stochastic programming: (a) most of the combinations of distribution estimation and resampling result in algorithms that have not been published before, (b) within the algorithms, we describe innovations that improve performance, and (c) we provide open-source software implementations of the algorithms. Among others, three important conclusions can be drawn: using a smoothed point estimate for the optimality gap for the center of the confidence interval is preferable to a purely empirical estimate, bagging often performs better than bootstrap, and smoothed bagging sometimes performs better than bagging based directly on the data.
MoreTranslated text
Key words
Optimization under uncertainty,Smoothed bootstrap,Bagging,Stochastic programming,Optimality gap
AI Read Science
Must-Reading Tree
Example
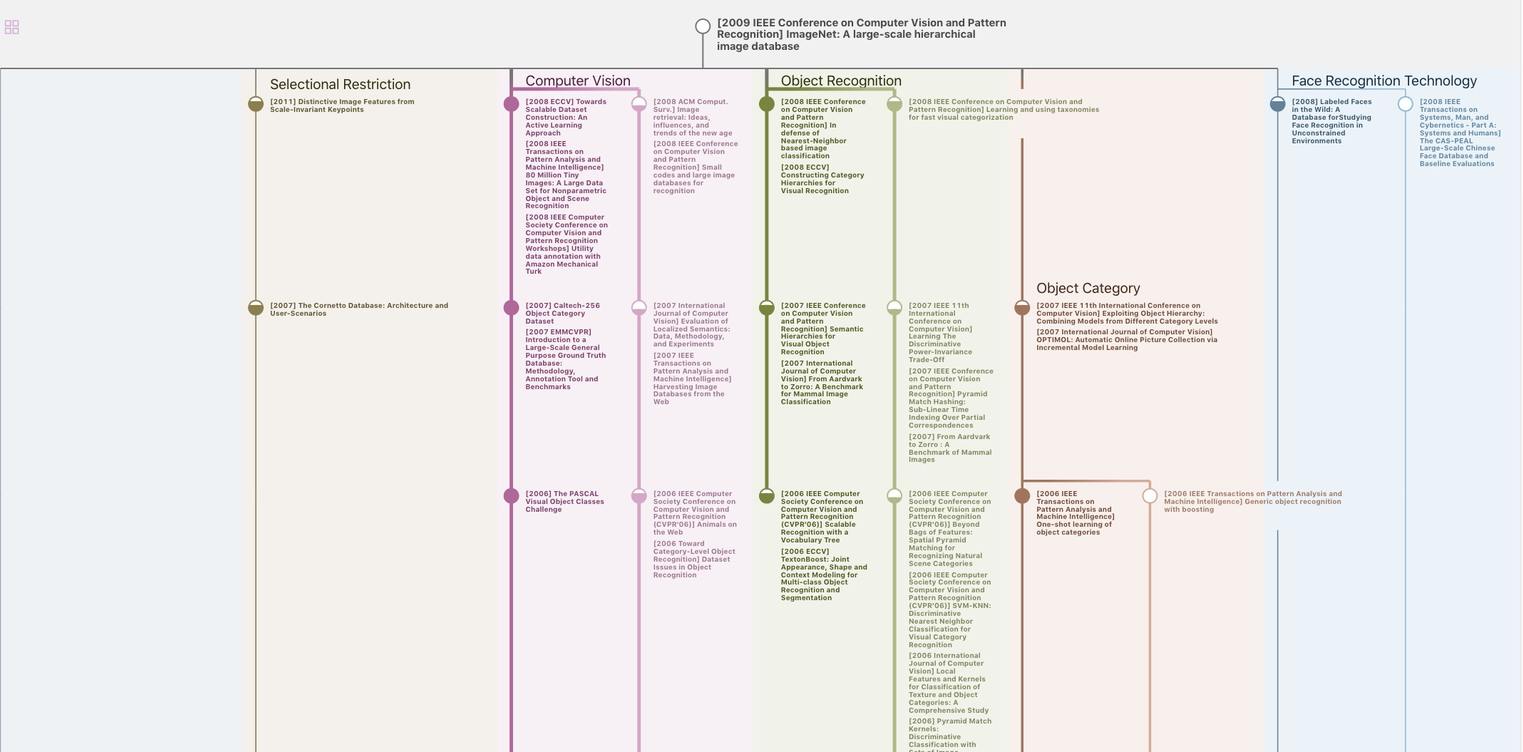
Generate MRT to find the research sequence of this paper
Chat Paper
Summary is being generated by the instructions you defined