Federated Automl Learning for Community Building Energy Prediction
NDE 40, PREDICTIVE MAINTENANCE, COMMUNICATION, AND ENERGY SYSTEMS THE DIGITAL TRANSFORMATION OF NDE II(2024)
摘要
Energy load forecasting across multiple buildings is beneficial for energy saving. Currently, most methodologies are training a single global model for all buildings as the deep learning model relies on large-scale data. However, the energy data distribution may vary a lot across different buildings and enforcing a global model may cause unnecessary computing resource overutilization. Meanwhile, building energy management encounters repeated manual efforts for machine learning model training over the new sensor data. To improve the computing resource utilization of load forecasting model training and automation of building energy management, a new automatic learning framework is proposed to support automatic building energy data analytics. The machine learning model is customized for each building based on an automatic algorithm with efficient model evaluations. The new framework brings comparable performance to federated energy data learning while fewer computing resource is consumed.
更多查看译文
关键词
Power prediction,Automl,Federated learning,Deep learning
AI 理解论文
溯源树
样例
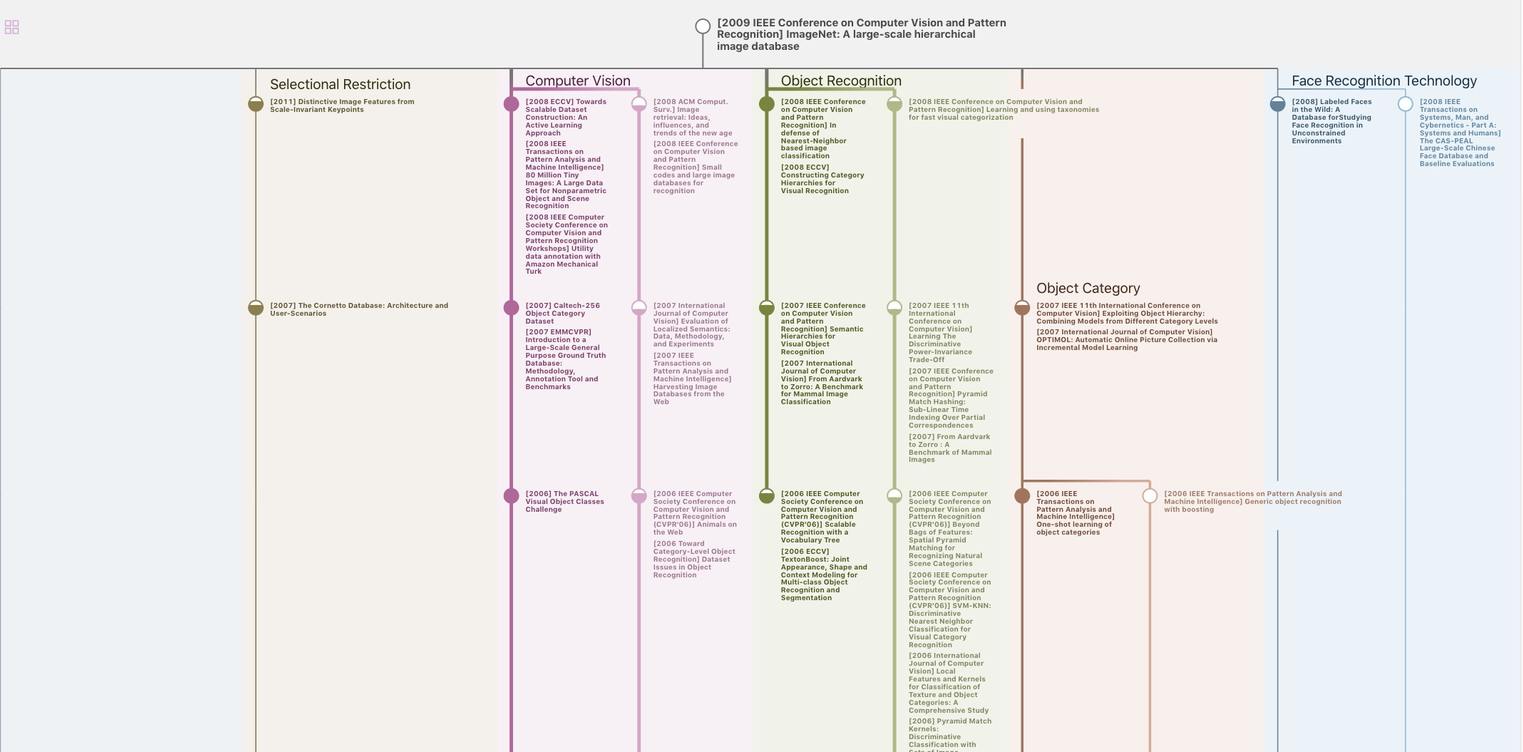
生成溯源树,研究论文发展脉络
Chat Paper
正在生成论文摘要