A novel MO-YOLOv4 for segmentation of multi-class bridge damages
ADVANCED ENGINEERING INFORMATICS(2024)
摘要
Most studies of multi-class damage segmentation overlook two prominent and critical challenges: the substantial variations in damage scale and the susceptibility of network training to background interferences. As a consequence, achieving the desired performance in multi-class damage segmentation becomes a difficult task. To tackle these challenges, a novel multi-class damage segmentation method, MO-YOLOv4, is proposed based on the widely used YOLOv4 structure. MO-YOLOv4 incorporates the Multi-Scale Attention (MSA) module to greatly enhance the feature extraction ability for multi-class damages, and employs the Oriented Bounding Box (OBB)based segmentation strategy for effectively reducing the influence of background interferences during the network training phase. The effectiveness of the MSA module and OBB-based segmentation strategy is validated through verification and ablation experiments. In the comparative study, the Mean Precision rate (MP), Mean Recall rate (MR), Mean F1-score (MF) and Mean Intersection over Union rate (MIoU) of the proposed MOYOLOv4 reach up to 82.49%, 72.73%, 77.04% and 64.28%, respectively, indicating the superior level of segmentation performance offered by MO-YOLOv4 in comparison to the mainstream semantic segmentation methods for multi-class damages.
更多查看译文
关键词
Multi-class damage segmentation,Convolutional neural network,YOLOv4,Multi-scale attention,Oriented bounding box
AI 理解论文
溯源树
样例
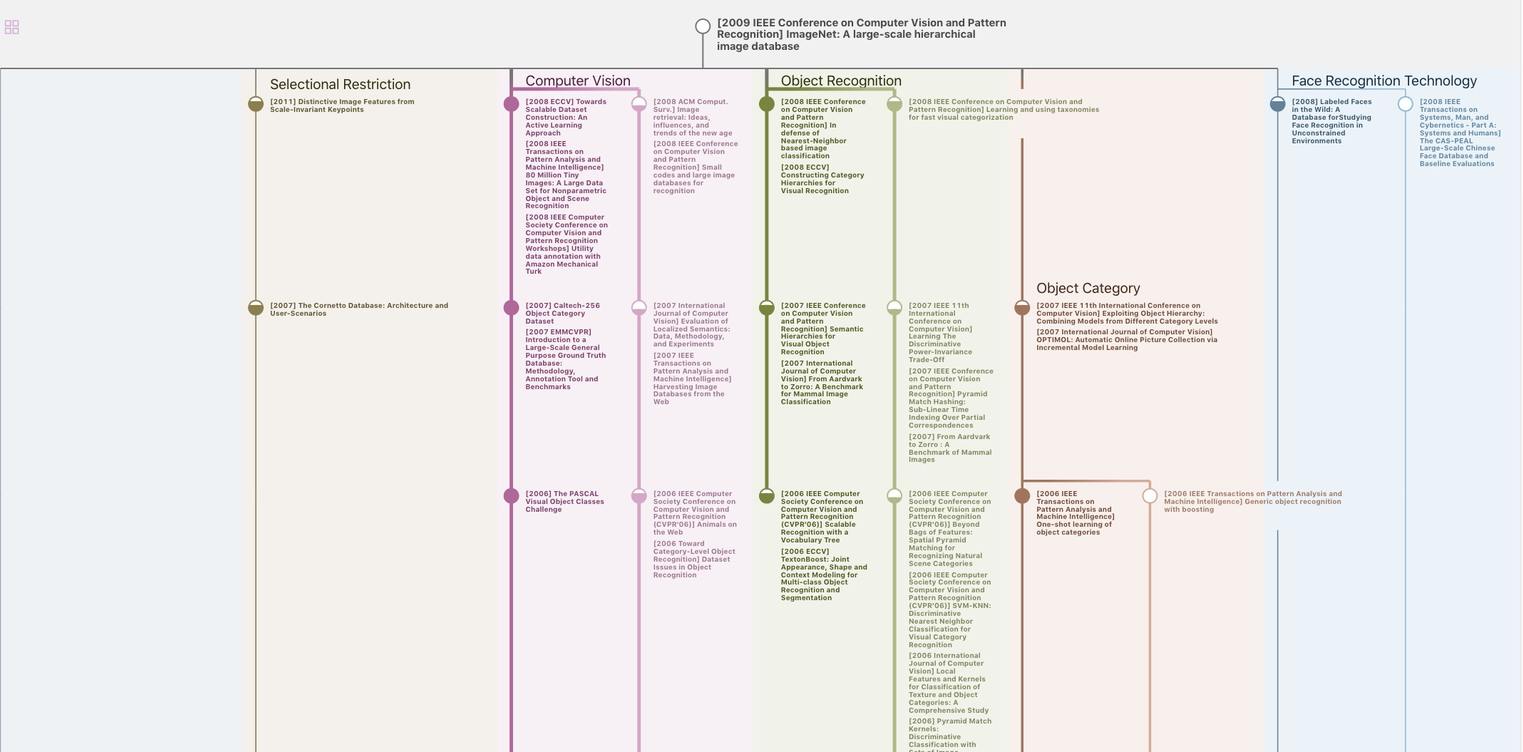
生成溯源树,研究论文发展脉络
Chat Paper
正在生成论文摘要