Enhancing surface drainage mapping in eastern Canada with deep learning applied to LiDAR-derived elevation data.
Scientific reports(2024)
摘要
Agricultural dykelands in Nova Scotia rely heavily on a surface drainage technique called land forming, which is used to alter the topography of fields to improve drainage. The presence of land-formed fields provides useful information to better understand land utilization on these lands vulnerable to rising sea levels. Current field boundaries delineation and classification methods, such as manual digitalization and traditional segmentation techniques, are labour-intensive and often require manual and time-consuming parameter selection. In recent years, deep learning (DL) techniques, including convolutional neural networks and Mask R-CNN, have shown promising results in object recognition, image classification, and segmentation tasks. However, there is a gap in applying these techniques to detecting surface drainage patterns on agricultural fields. This paper develops and tests a Mask R-CNN model for detecting land-formed fields on agricultural dykelands using LiDAR-derived elevation data. Specifically, our approach focuses on identifying groups of pixels as cohesive objects within the imagery, a method that represents a significant advancement over pixel-by-pixel classification techniques. The DL model developed in this study demonstrated a strong overall performance, with a mean Average Precision (mAP) of 0.89 across Intersection over Union (IoU) thresholds from 0.5 to 0.95, indicating its effectiveness in detecting land-formed fields. Results also revealed that 53% of Nova Scotia's dykelands are being used for agricultural purposes and approximately 75% (6924 hectares) of these fields were land-formed. By applying deep learning techniques to LiDAR-derived elevation data, this study offers novel insights into surface drainage mapping, enhancing the capability for precise and efficient agricultural land management in regions vulnerable to environmental changes.
更多查看译文
关键词
Mask RCNN,Deep learning,Dykelands,Remote sensing,Precision agriculture,NOVA Scotia
AI 理解论文
溯源树
样例
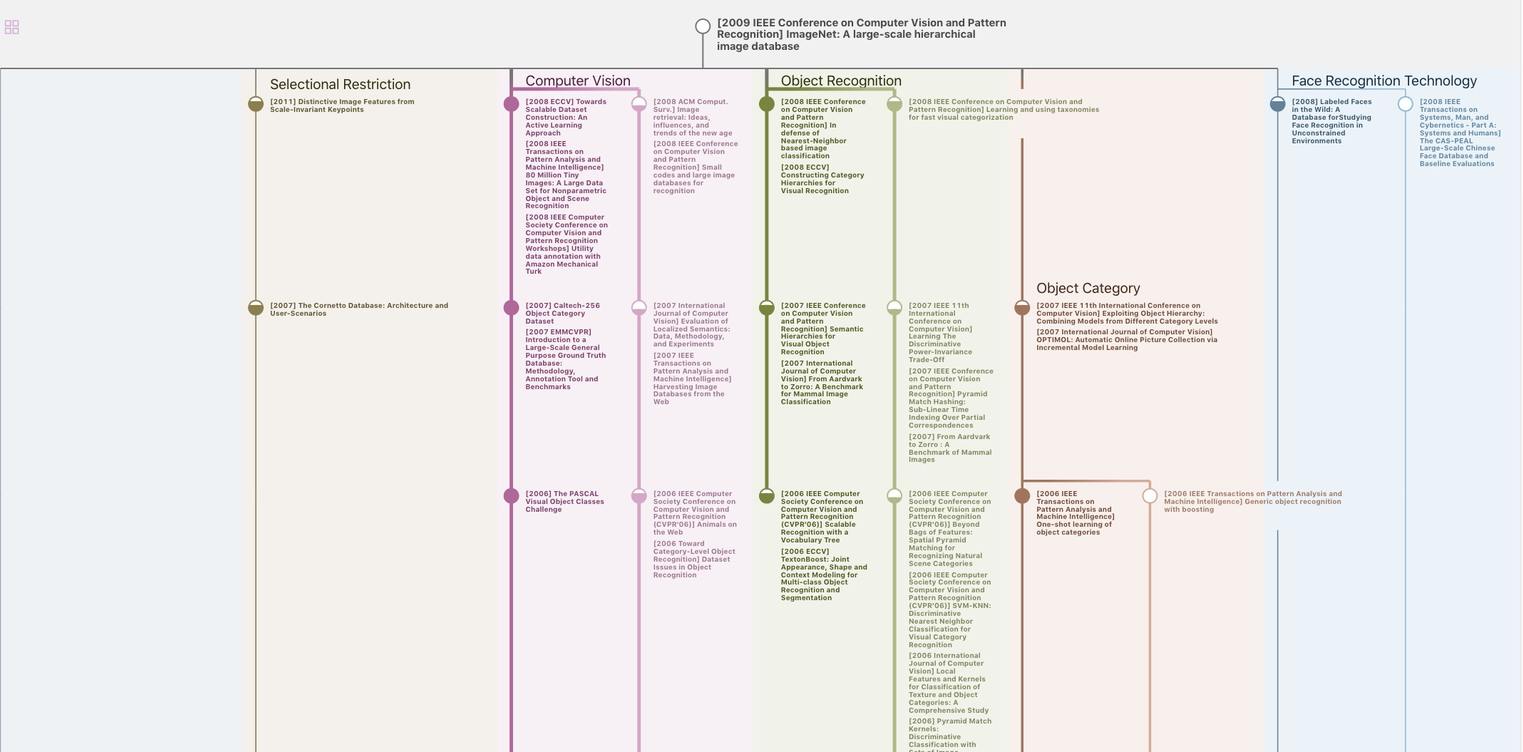
生成溯源树,研究论文发展脉络
Chat Paper
正在生成论文摘要