High-throughput Discovery of Metal Oxides with High Thermoelectric Performance Via Interpretable Feature Engineering on Small Data
Materials today physics(2024)
摘要
In this work, we have proposed a data-driven screening framework combiningthe interpretable machine learning with high-throughput calculations toidentify a series of metal oxides that exhibit both high-temperature toleranceand high power factors. Aiming at the problem of weak generalization ability ofsmall data with power factors at high temperatures, we employ symbolicregression for feature creation which enhances the robustness of the modelwhile preserving the physical meaning of features. 33 candidate metal oxidesare finally targeted for high-temperature thermoelectric applications from apool of 48,694 compounds in the Materials Project database. The Boltzmanntransport theory is utilized to perform electrical transport propertiescalculations at 1,000 K. The relaxation time is approximated by employingconstant electron-phonon coupling based on the deformation potential theory.Considering band degeneracy, the electron group velocity is obtained using themomentum matrix element method, yielding 28 materials with power factorsgreater than 50 μW cm^-1 K^-2. The high-throughput framework weproposed is instrumental in the selection of metal oxides for high-temperaturethermoelectric applications. Furthermore, our data-driven analysis andtransport calculation suggest that metal oxides rich in elements such as cerium(Ce), tin (Sn), and lead (Pb) tend to exhibit high power factors at hightemperatures.
更多查看译文
AI 理解论文
溯源树
样例
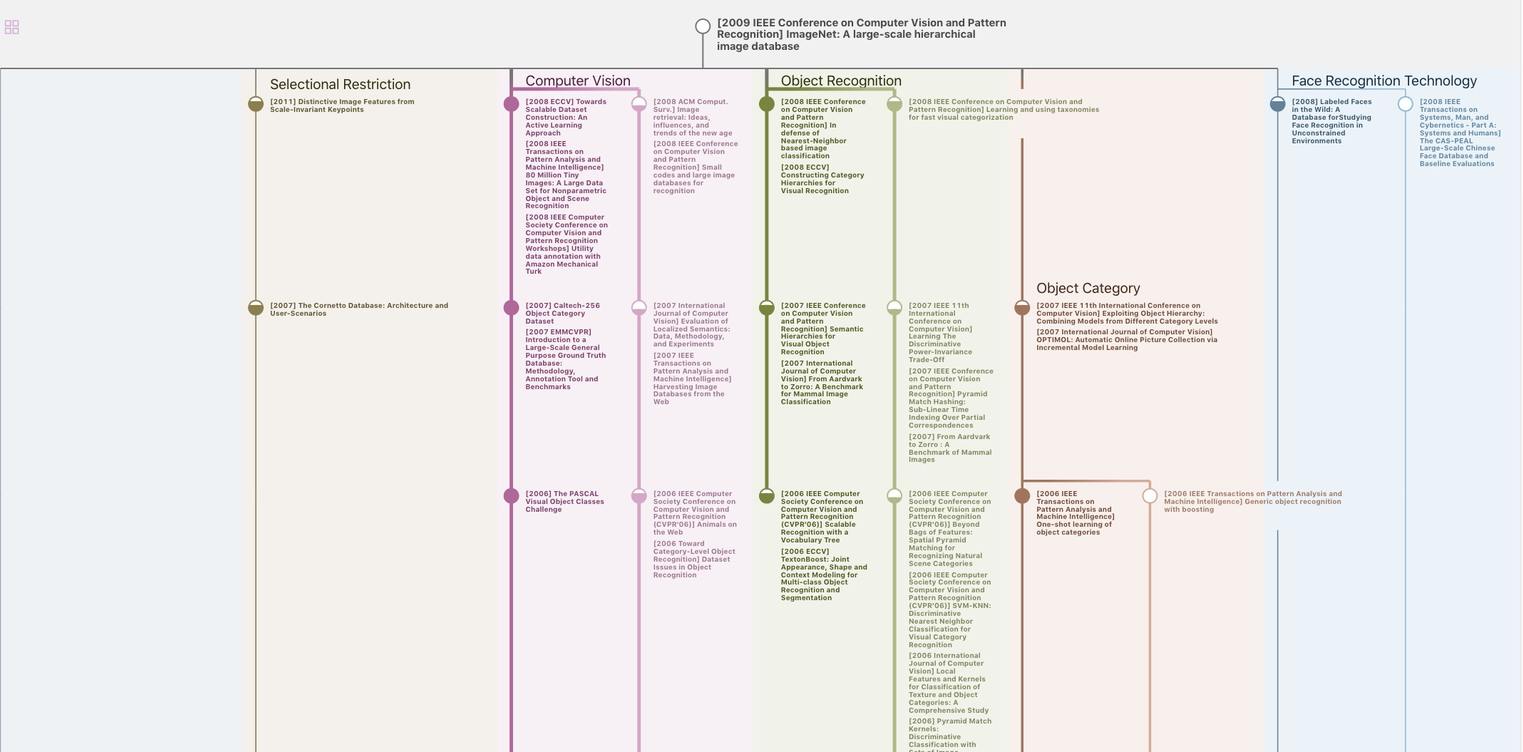
生成溯源树,研究论文发展脉络
Chat Paper
正在生成论文摘要