Natural Local Density-Based Adaptive Oversampling Algorithm for Imbalanced Classification
Knowledge-Based Systems(2024)
摘要
Oversampling-based methods achieve impressive performance for classifying imbalanced data. However, many existing oversampling algorithms are still very sensitive to noise. Besides, these algorithms also require one or more parameters, how to set these parameters is very challenging. Furthermore, the generated samples by these algorithms are meaningless or unsafe. To solve these problems, a novel oversampling algorithm for unbalanced classification is presented, named Natural Local Density-based Adaptive Oversampling algorithm (NLDAO). NLDAO has four main advantages: (a) it does not need any parameter due to the use of natural neighbors to calculate local density; (b) it applies a noisy filter to remove noises, which makes the sample boundary cleaner so that the proposed method is robust to noise; (c) it unevenly distributes the generated samples to the minority class, which maintains the original characteristics and enhances the boundary classification ability; (d) it determines the generated region by tracking the sample proportion drop that declines the randomness of the synthesized and reduces the noisy generation. Finally, in experiments, we apply the NLDAO algorithm to artificial datasets to visually demonstrate its effectiveness. Moreover, intensive experiments on real datasets show that NLDAO can achieve better performance than state-of-the-art methods.
更多查看译文
关键词
Natural neighbor,Instance-based learning,Synthesis strategy,Kernel density estimation,Classification
AI 理解论文
溯源树
样例
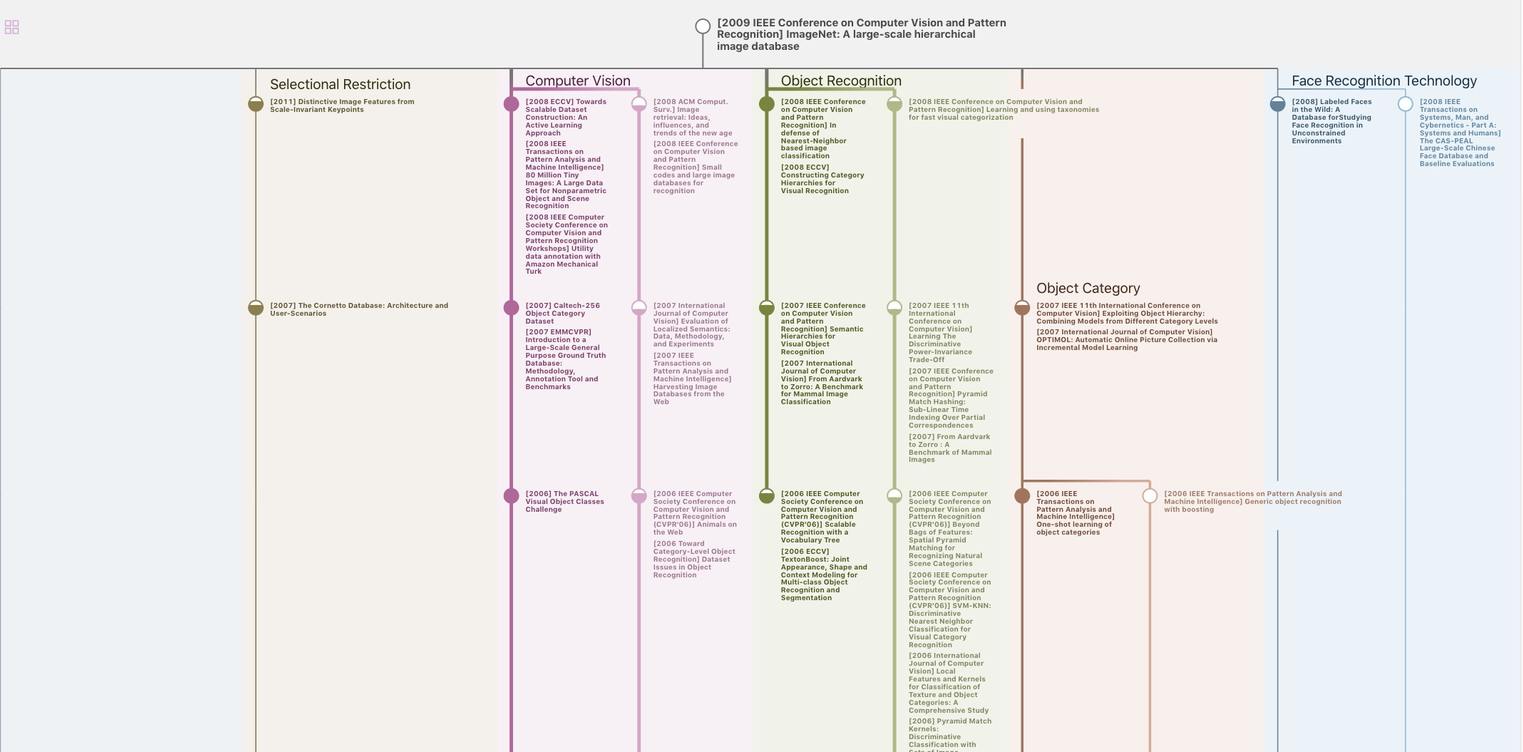
生成溯源树,研究论文发展脉络
Chat Paper
正在生成论文摘要