PDAS: a Newton-type method for L_0 regularized accelerated failure time model
Computational Statistics(2024)
Abstract
Regularization methods are commonly utilized in survival analysis to address variable selection and estimation problems. Although most of the penalties can be regarded as variations of L_0 regularization to handle computational challenges, they may not always be efficient or effective in sparse recovery scenarios with massive amounts of data. To address this concern, this paper proposes a method for L_0 regularized estimation in the high-dimensional accelerated failure time (AFT) model, called the Primal Dual Active Set (PDAS) algorithm. Our approach introduces a tuning parameter to select active sets based on primal and dual information and performs root finding using the Karush-Kuhn-Tucker (KKT) conditions. To generate a sequence of solutions iteratively, this work also presents a sequential Primal Dual Active Set (SPDAS) algorithm that incorporates the PDAS algorithm in each iteration. Our approach can be classified as a Newton-type method to address the L_0 regularization problem directly. Extensive analysis, including simulations and real data studies, demonstrates that our approach provides competitive performance in terms of computational efficiency and predictive accuracy compared with existing methods for sparse recovery.
MoreTranslated text
Key words
regularization,KKT conditions,Sparse recovery,High dimension,AFT model
AI Read Science
Must-Reading Tree
Example
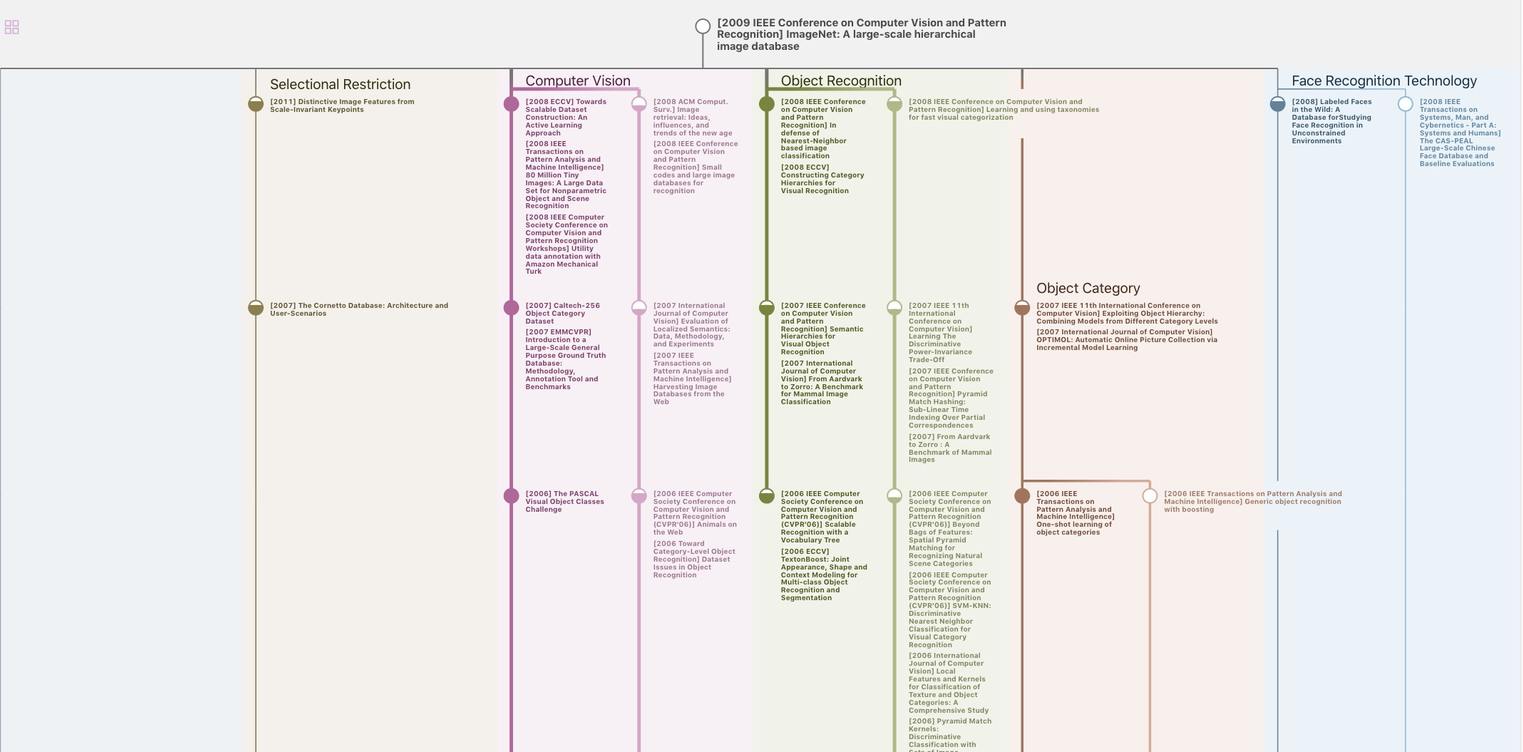
Generate MRT to find the research sequence of this paper
Chat Paper
Summary is being generated by the instructions you defined