The influence of cartographic representation on landslide susceptibility models: empirical evidence from a Brazilian UNESCO world heritage site
Natural Hazards(2024)
摘要
The influence of cartographic representation of landslides on susceptibility models is well-known but often neglected by academic studies, which rarely explore its practical implications. This study aims to address this knowledge gap by evaluating the impact of different landslide sampling strategies on statistical models of landslide susceptibility in Ouro Preto, a UNESCO World Heritage Site located in South-eastern Brazil. The study’s main objective was to assess how different landslide sampling strategies affected statistical susceptibility models. It adopted an innovative methodological approach that categorized dependent variables between training and test subgroups, adopting both balanced and unbalanced divisions of dependent variables, and focusing on shallow and deep landslides. In addition, the study introduced a systematic and critical approach to cartographic representation, providing valuable insights for future research and practice on landslide susceptibility mapping. Eighteen models were produced using an inventory with 57 historical landslides mapped with an Unmanned Aerial Vehicle. The area and volume of these landslides were determined. Three divisions of dependent variables were adopted between training and test subgroups: one balanced division, with large and deep landslides in both subgroups, and two unbalanced divisions, with a predominance of large and deep landslides in the training subgroup or test subgroup. The construction of landslide susceptibility models employed the information value method, validated through success and prediction curves. The results show the significant influence of cartographic representation (point or polygon) on the quality of statistical models and the spatial distribution of susceptibility classes. The polygonal cartographic representation and balanced partition of dependent variables produced the best results. However, it is emphasized that this cartographic representation is not universally optimal in other contexts. The worst result was obtained using a point and random cartographic representation. Overall, findings indicate the need for more accurate landslide inventories and databases, possibly through standards and regulations.
更多查看译文
AI 理解论文
溯源树
样例
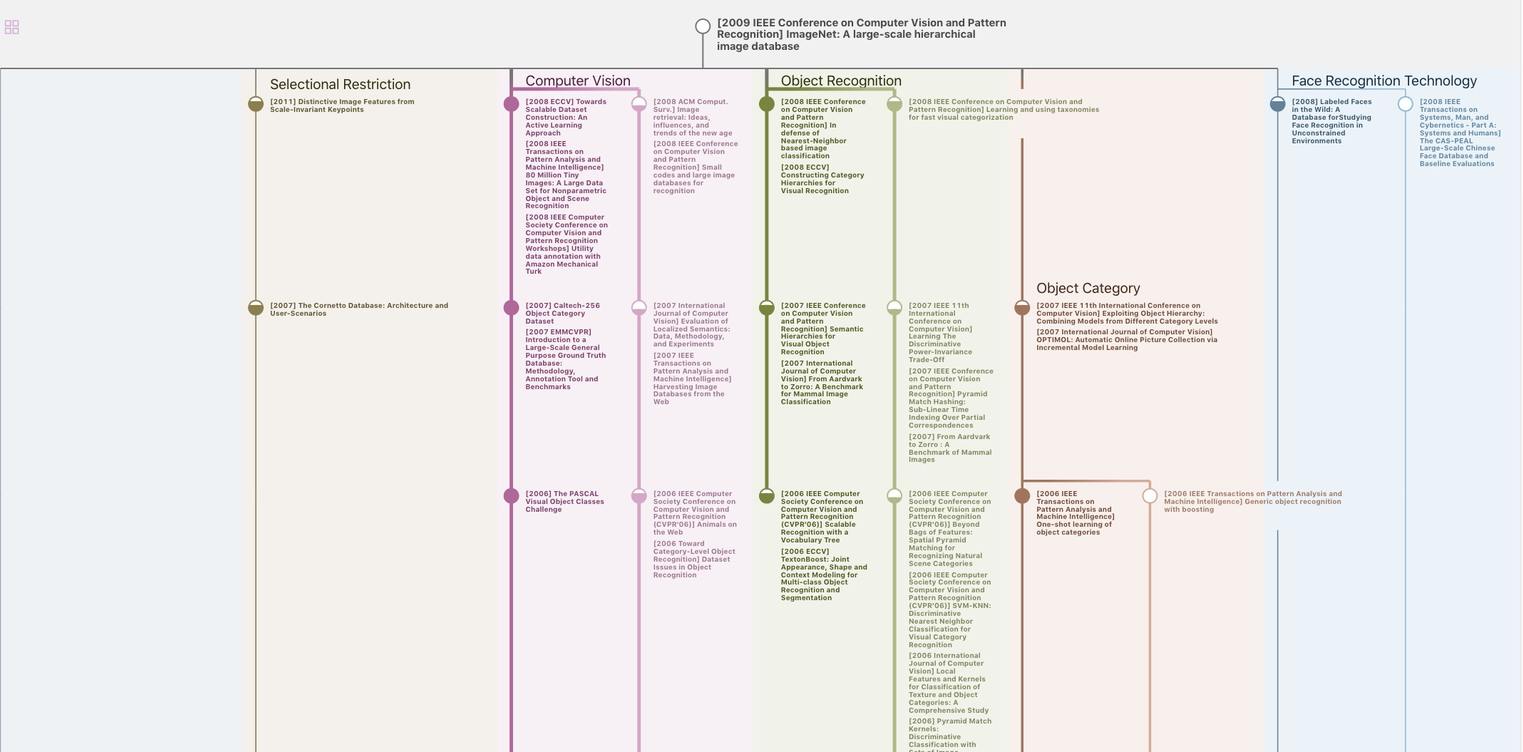
生成溯源树,研究论文发展脉络
Chat Paper
正在生成论文摘要