Optimising Building Energy and Comfort Predictions with Intelligent Computational Model
Sustainability(2024)
摘要
Building performance prediction is a significant area of research, due to its potential to enhance the efficiency of building energy management systems. Its importance is particularly evident when such predictions are validated against field data. This paper presents an intelligent computational model combining Monte Carlo analysis, Energy Plus, and an artificial neural network (ANN) to refine energy consumption and thermal comfort predictions. This model addresses various combinations of architectural building design parameters and their distributions, effectively managing the complex non-linear relationships between the response variables and predictors. The model’s strength is demonstrated through its alignment with R2 values exceeding 0.97 for both thermal discomfort hours and energy consumption during the training and testing phases. Validation with field investigation data further confirms its accuracy, demonstrating average relative errors below 2.0% for total energy consumption and below 1.0% for average thermal discomfort hours. In particular, an average underestimation of −12.5% in performance discrepancies is observed when comparing the building energy simulation model with field data, while the intelligent computational model presented a smaller overestimation error (of +8.65%) when validated against the field data. This discrepancy highlights the model’s potential and reliability for the simulation of real-world building performance metrics, marking it as a valuable tool for practitioners and researchers in the field of building sustainability.
更多查看译文
关键词
artificial neural networks,architectural building design parameters,energy consumption,educational buildings,thermal comfort
AI 理解论文
溯源树
样例
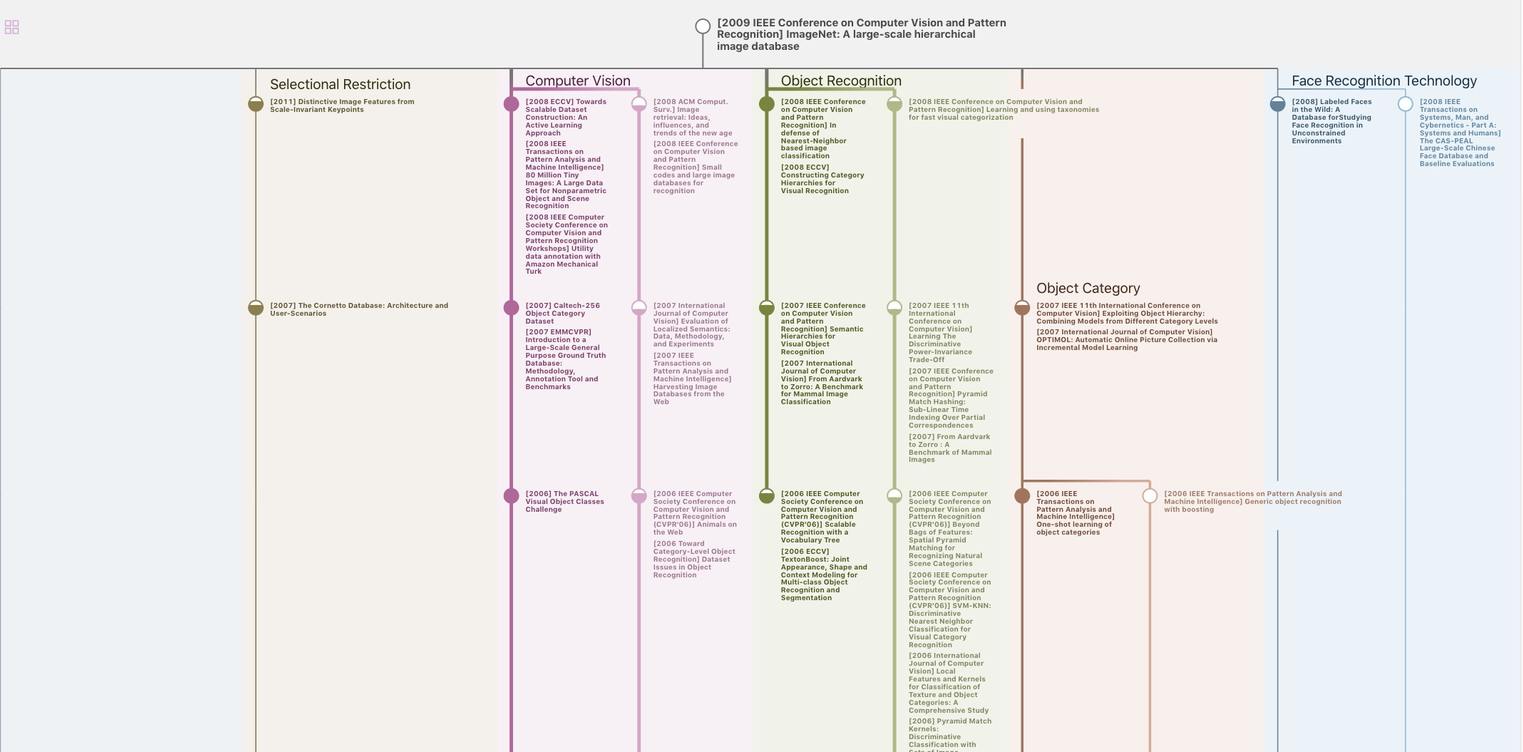
生成溯源树,研究论文发展脉络
Chat Paper
正在生成论文摘要