Application of Machine Learning for Productivity Prediction in Tight Gas Reservoirs
Energies(2024)
摘要
Accurate well productivity prediction plays a significant role in formulating reservoir development plans. However, traditional well productivity prediction methods lack accuracy in tight gas reservoirs; therefore, this paper quantitatively evaluates the correlations between absolute open flow and the critical parameters for Linxing tight gas reservoirs through statistical analysis. Dominant control factors are obtained by considering reservoir engineering theories, and a novel machine learning-based well productivity prediction method is proposed for tight gas reservoirs. The adaptability of the productivity prediction model is assessed through machine learning and field data analysis. Combined with the typical decline curve analysis, the estimated ultimate recovery (EUR) of a single well in the tight gas reservoir is forecasted in an appropriate range. The results of the study include 10 parameters (such as gas saturation) identified as the dominant controlling factors for well productivity and geological factors that impact the productivity in this area compared to fracturing parameters. According to the prediction results of the three models, the R2 of Support Vector Regression (SVR), Back Propagation (BP), and Random Forest (RF) models are 0.72, 0.87, and 0.91, respectively. The results indicate that RF has a more accurate prediction. In addition, the RF model is more suitable for medium and high-production wells based on the actual field data. Based on this model, it is verified that the productivity of low-producing wells is affected by water production. This study confirms the model’s reliability and application value by predicting recoverable reserves for a single well.
更多查看译文
关键词
tight gas reservoirs,machine learning (ML),well productivity prediction,dominant controlling factors,recoverable reserves
AI 理解论文
溯源树
样例
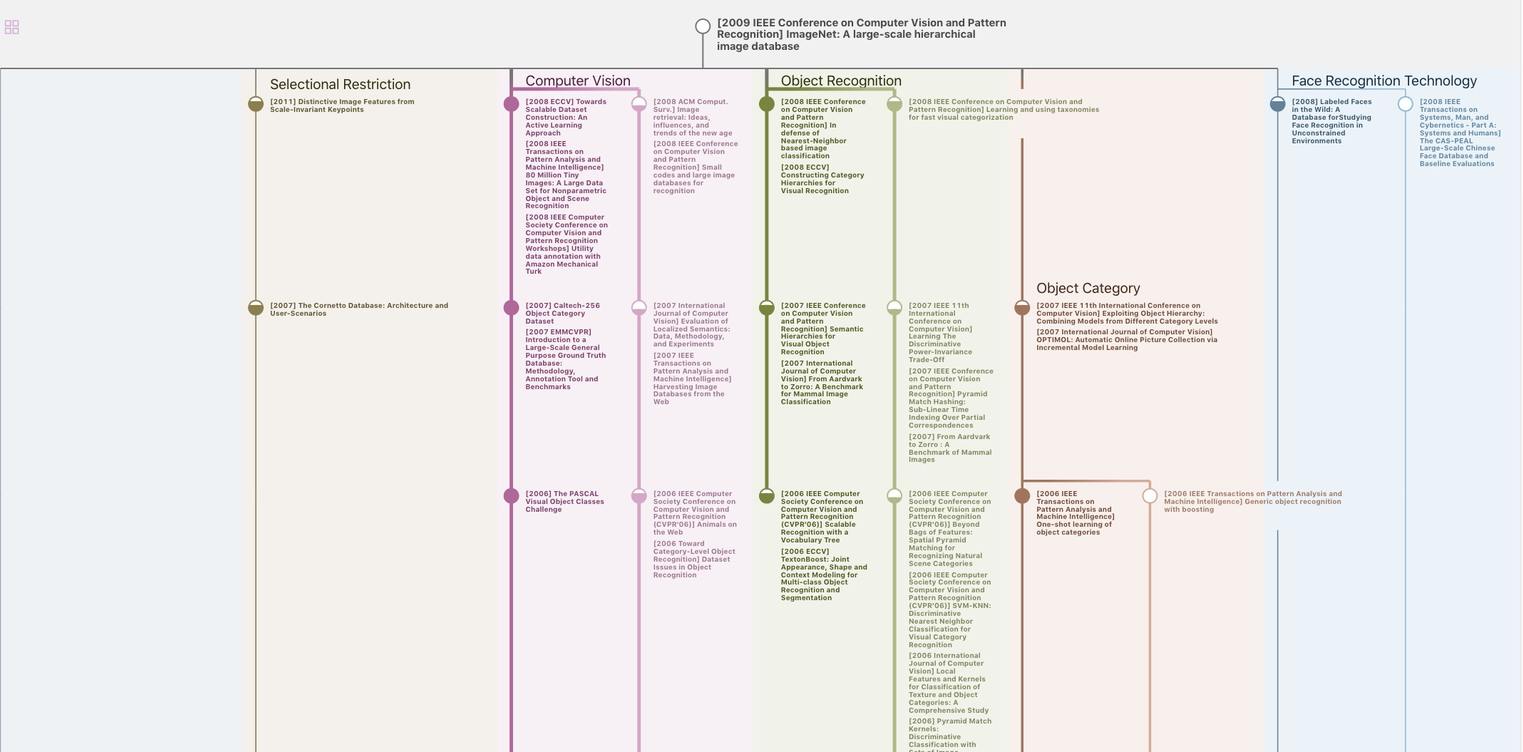
生成溯源树,研究论文发展脉络
Chat Paper
正在生成论文摘要