Applying Transformer-Based Neural Networks to Corpus Linguistic Data for Predictive Text Generation in Multilingual Environments
2024 International Conference on Emerging Smart Computing and Informatics (ESCI)(2024)
摘要
In the subject of corpus linguistics, this study explores the use of transformer-based neural networks to the problems of predictive text production in multilingual contexts. The Transformer architecture presents a viable framework for natural language processing problems because of its reputation for capturing complex patterns and long-range dependencies in sequential data. The research employs a two-phase approach, whereby the Transformer is first pre-trained using a variety of multilingual corpora. This helps the model learn representations that are independent of language, which makes it easier for it to adapt to various linguistic circumstances. The model is then fine-tuned using language-specific datasets to improve its ability to produce linguistically nuanced and contextually appropriate text. The study looks into how different model architectures, training methods, and hyperparameters affect how well the suggested multilingual predictive text generation system performs. Evaluation measures are used to evaluate the model's performance in several languages, including linguistic diversity measurements, BLEU scores, and perplexity. The overall accuracy, calculated across all languages, stands at 80.3%, indicating the model's robust cross-lingual generalization and its effectiveness in capturing diverse linguistic nuances The efficacy and generalization of Transformer-based models are improved across a wide range of languages to handle linguistic diversity.
更多查看译文
关键词
Multilingual Text Generation,Neural Language Models,Cultural Context Integration,Corpus Linguistics,Transformer-Based Neural Networks
AI 理解论文
溯源树
样例
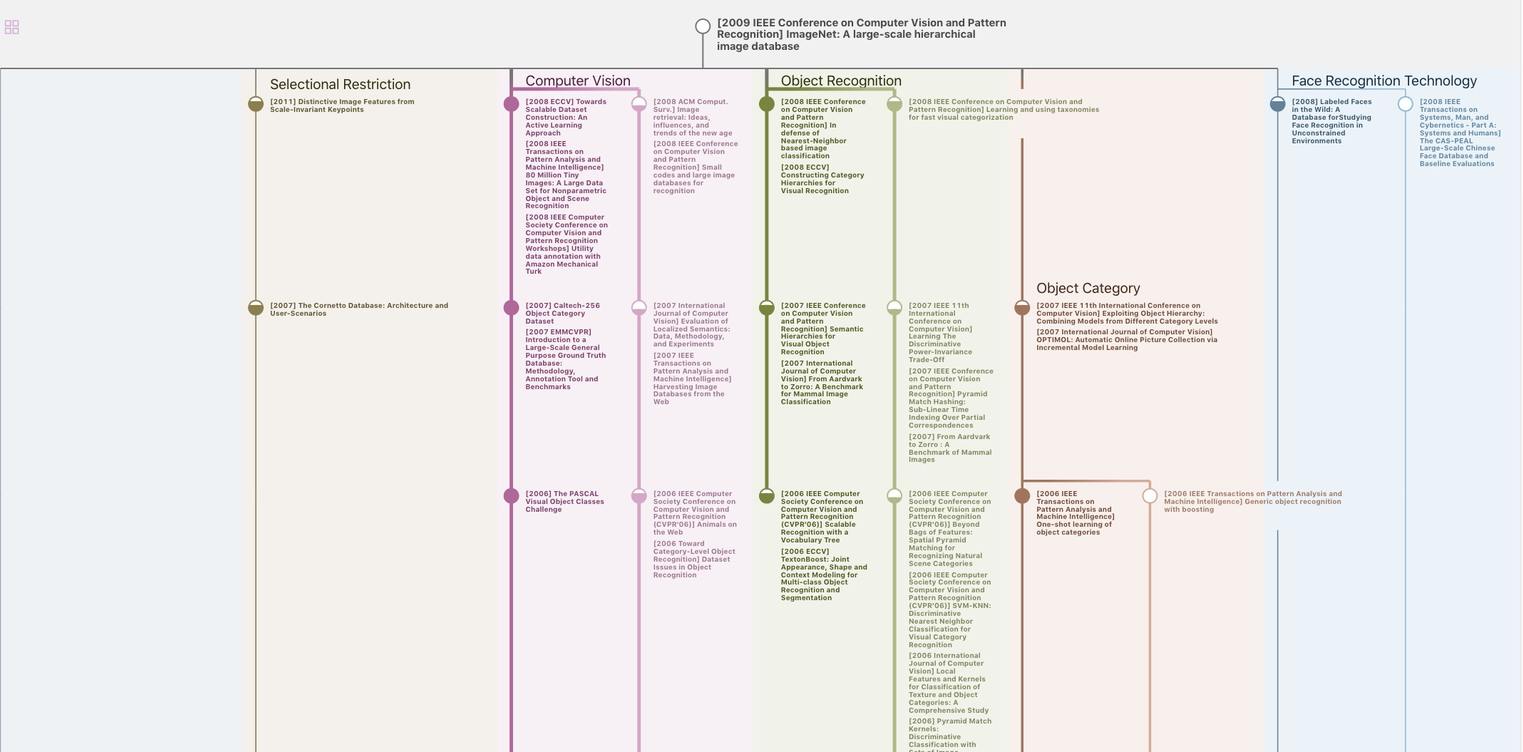
生成溯源树,研究论文发展脉络
Chat Paper
正在生成论文摘要