MF-Net: multi-scale feature extraction-integration network for unsupervised deformable registration
FRONTIERS IN NEUROSCIENCE(2024)
Abstract
Deformable registration plays a fundamental and crucial role in scenarios such as surgical navigation and image-assisted analysis. While deformable registration methods based on unsupervised learning have shown remarkable success in predicting displacement fields with high accuracy, many existing registration networks are limited by the lack of multi-scale analysis, restricting comprehensive utilization of global and local features in the images. To address this limitation, we propose a novel registration network called multi-scale feature extraction-integration network (MF-Net). First, we propose a multiscale analysis strategy that enables the model to capture global and local semantic information in the image, thus facilitating accurate texture and detail registration. Additionally, we introduce grouped gated inception block (GI-Block) as the basic unit of the feature extractor, enabling the feature extractor to selectively extract quantitative features from images at various resolutions. Comparative experiments demonstrate the superior accuracy of our approach over existing methods.
MoreTranslated text
Key words
deformable image registration,unsupervised learning,convolutional neural network,multi-scale,gating mechanism
AI Read Science
Must-Reading Tree
Example
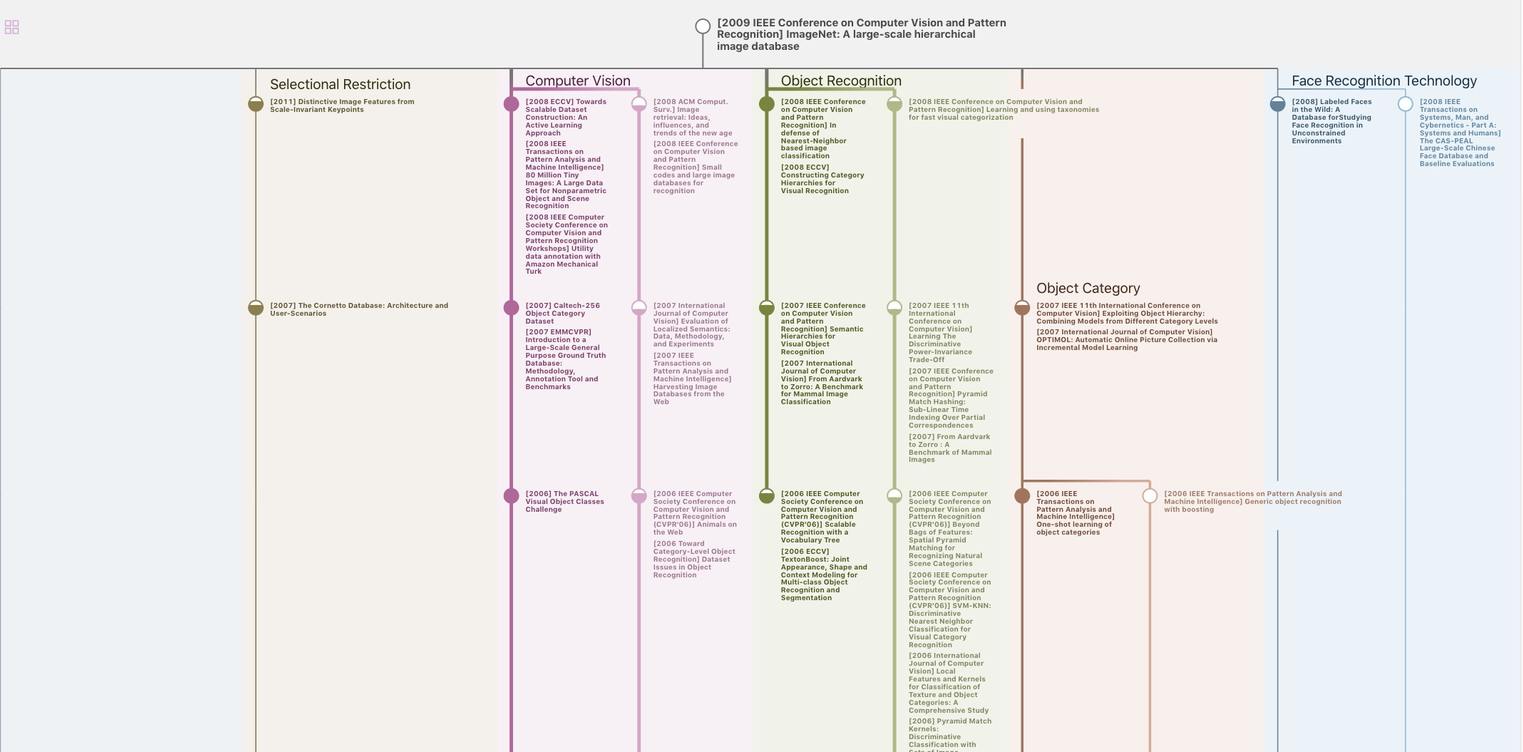
Generate MRT to find the research sequence of this paper
Chat Paper
Summary is being generated by the instructions you defined