Prediction of Interstitial Glucose Levels Through Wearable Sensors Using Machine Learning
2023 IEEE Asia-Pacific Conference on Computer Science and Data Engineering (CSDE)(2023)
摘要
The incidence of diabetes has been increasing, resulting in an increasing cost of managing and tracking the correlates for blood glucose levels. Just for New Zealand alone, 2.1 billion dollars are spent annually on diabetes management. This is 4 times the current tertiary education budget in the same country. Compared to the traditional cumulative HbA1c marker of glucose used to monitor the health of diabetics, continuous glucose monitoring allows for tracking the trends in glucose value in real time. It also helps in gauging important statistics such as time in range of glucose values. For healthy individuals, glucose tracking can inform the user of the effect various activities and foods have on an individual's level. This paper aims to present a method of predicting the level of glucose through wearable continuous glucose monitors (Empatica E4 and Dexcom) and machine learning. The data from these wearable sensors comprises of inter beat interval (IBI), electrodermal activity (EDA), tri-axial accelerometry (ACC), heart rate (HR), skin temperature, food log, and blood volume pulse (BVP). It was collected over 8-10 days for 19 individuals and used to calculate 73 statistical features. 69 features were used from previous literature and 4 novel features proposed in this research were used. These features were in turn used to train support vector machine (SVM) and decision tree model (DTM) with stratified K-fold validation which yield accuracies of 69.10 ± 14.2 % and 72.38 ± 2.4 %. The combined feature importance of the 4 novel features was calculated to be 5%, highlighting their significance in the model.
更多查看译文
AI 理解论文
溯源树
样例
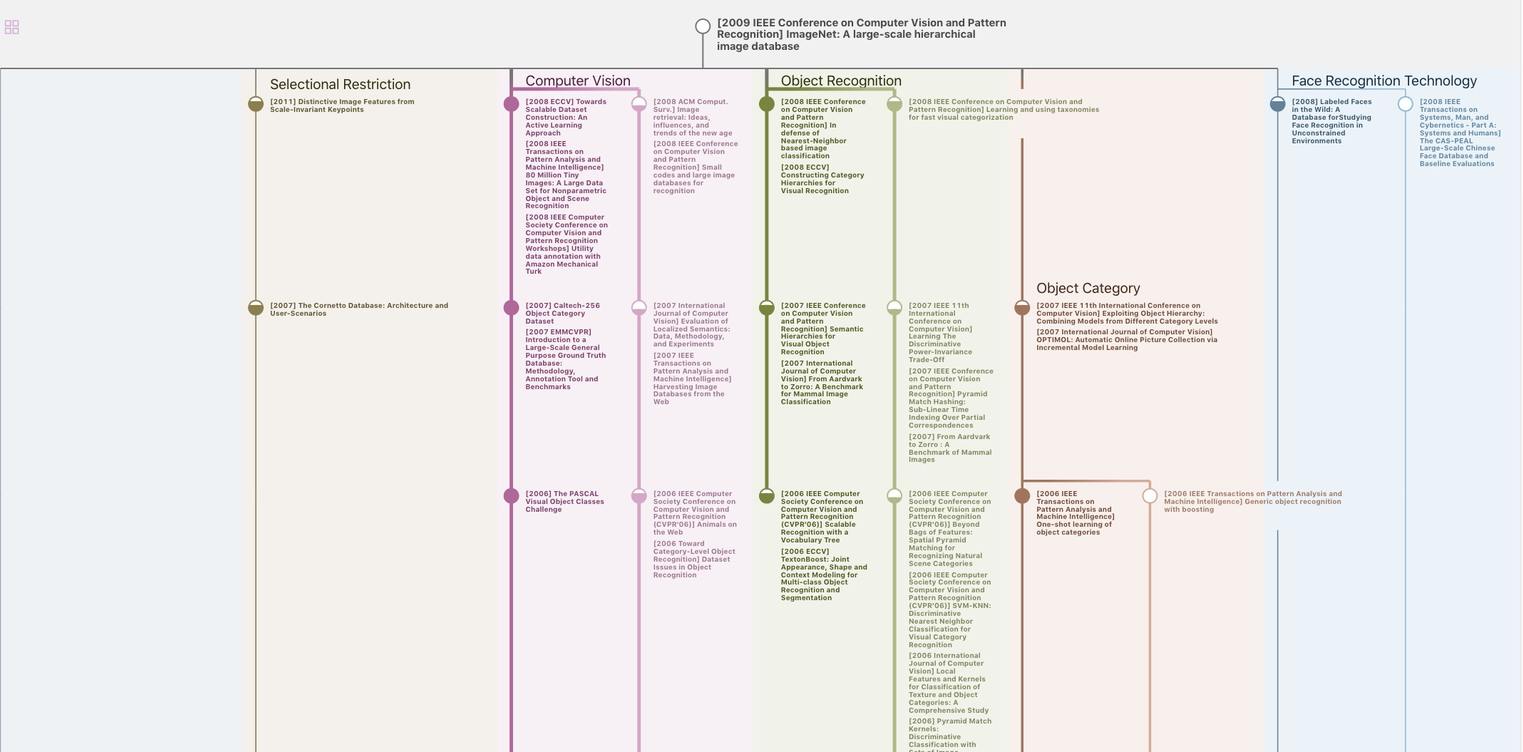
生成溯源树,研究论文发展脉络
Chat Paper
正在生成论文摘要