Risk assessment of mine water inrush based on Semi-Supervised Deep Learning Model
crossref(2024)
Abstract
Abstract To establish an effective coal mine floor water inrush prediction model, a semi-supervised model based on improved tri-training is presented. By using unlabeled data, the semi supervised model solves the limitation of limited labeled data in the water inrush dataset. Since water inrush characteristics have varying effects on accident occurrence, this paper proposes a mutual information Drop-SAE as the fundamental classifier for the semi-supervised model. The correlation between features and targets is assessed using mutual information, and features with weak correlation have their weights reset to zero to reduce the influence of irrelevant features on prediction accuracy. By contrasting water inrush incidents and model prediction results in typical North Chinese mining areas with real mining areas in Lianghuai, the superiority of this approach was confirmed. The results obtained demonstrate that, in contrast to conventional prediction techniques, the model presented in this article has an accuracy of 91.43%, whereas SAE's accuracy is 82.86%. In comparison to models that have been proposed recently (like IWOA-SVM), this model exhibits a 3% improvement in accuracy. The research results can be used in the prediction of water inrush, combining deep learning with semi-supervised models. The results have theoretical and practical significance.
MoreTranslated text
AI Read Science
Must-Reading Tree
Example
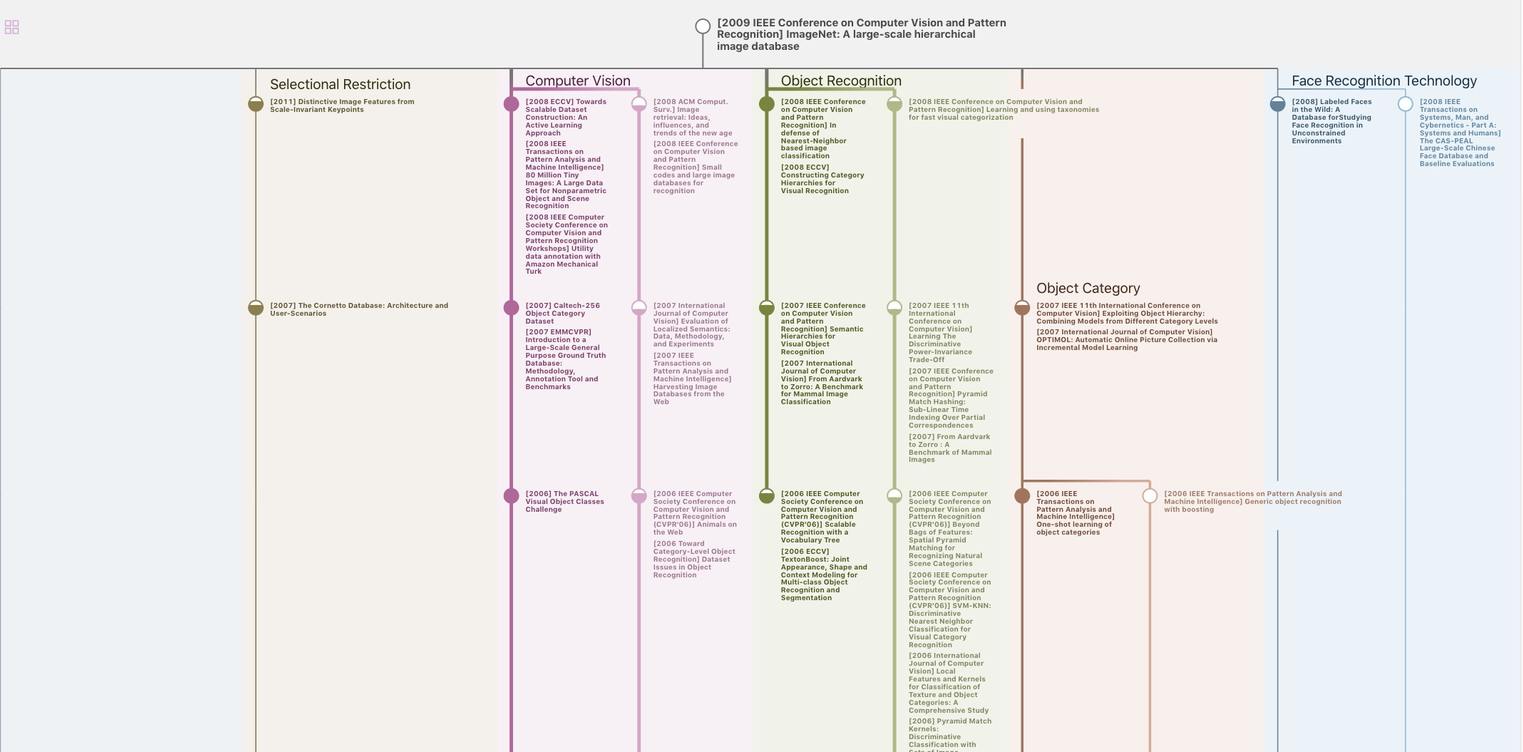
Generate MRT to find the research sequence of this paper
Chat Paper
Summary is being generated by the instructions you defined