A Neural Multigrid Solver for Helmholtz Equations with High Wavenumber and Heterogeneous Media
CoRR(2024)
Abstract
Solving high-wavenumber and heterogeneous Helmholtz equations presents a
long-standing challenge in scientific computing. In this paper, we introduce a
deep learning-enhanced multigrid solver to address this issue. By conducting
error analysis on standard multigrid applied to a discrete Helmholtz equation,
we devise a strategy to handle errors with different frequencies separately.
For error components with frequencies distant from the wavenumber, we perform
simple smoothing based on local operations at different levels to eliminate
them.
On the other hand, to address error components with frequencies near the
wavenumber, we utilize another multigrid V-cycle to solve an
advection-diffusion-reaction (ADR) equation at a coarse scale.
The resulting solver, named Wave-ADR-NS, involves parameters learned through
unsupervised training.
Numerical results demonstrate that Wave-ADR-NS effectively resolves
heterogeneous 2D Helmholtz equation with wavenumber up to 2000. Comparative
experiments against classical multigrid preconditioners and existing deep
learning-based multigrid preconditioners reveals the superior performance of
Wave-ADR-NS.
MoreTranslated text
AI Read Science
Must-Reading Tree
Example
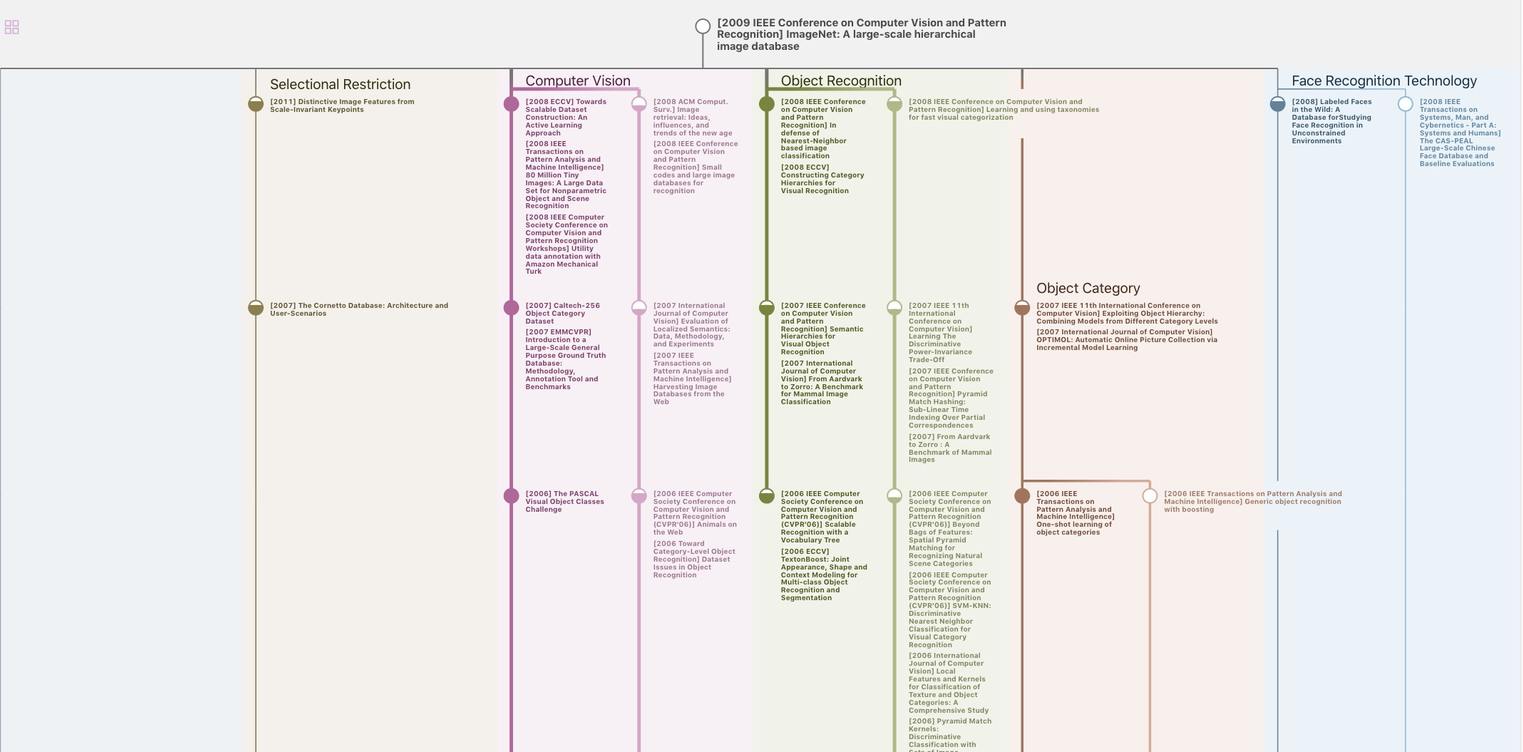
Generate MRT to find the research sequence of this paper
Chat Paper
Summary is being generated by the instructions you defined