Machine learning techniques for reinforced concrete's tensile strength assessment under different wetting and drying cycles
STEEL AND COMPOSITE STRUCTURES(2023)
摘要
Successive wetting and drying cycles of concrete due to weather changes can endanger the safety of engineering structures over time. Considering wetting and drying cycles in concrete tests can lead to a more correct and reliable design of engineering structures. This study aims to provide a model that can be used to estimate the resistance properties of concrete under different wetting and drying cycles. Complex sample preparation methods, the necessity for highly accurate and sensitive instruments, early sample failure, and brittle samples all contribute to the difficulty of measuring the strength of concrete in the laboratory. To address these problems, in this study, the potential ability of six machine learning techniques, including ANN, SVM, RF, KNN, XGBoost, and NB, to predict the concrete's tensile strength was investigated by applying 240 datasets obtained using the Brazilian test (80% for training and 20% for test). In conducting the test, the effect of additives such as glass and polypropylene, as well as the effect of wetting and drying cycles on the tensile strength of concrete, was investigated. Finally, the statistical analysis results revealed that the XGBoost model was the most robust one with R2 = 0.9155, mean absolute error (MAE) = 0.1080 Mpa, and variance accounted for (VAF) = 91.54% to predict the concrete tensile strength. This work's significance is that it allows civil engineers to accurately estimate the tensile strength of different types of concrete. In this way, the high time and cost required for the laboratory tests can be eliminated.
更多查看译文
关键词
machine learning,reinforced concrete,steel-concrete composite,tensile strength,wetting-drying cycles
AI 理解论文
溯源树
样例
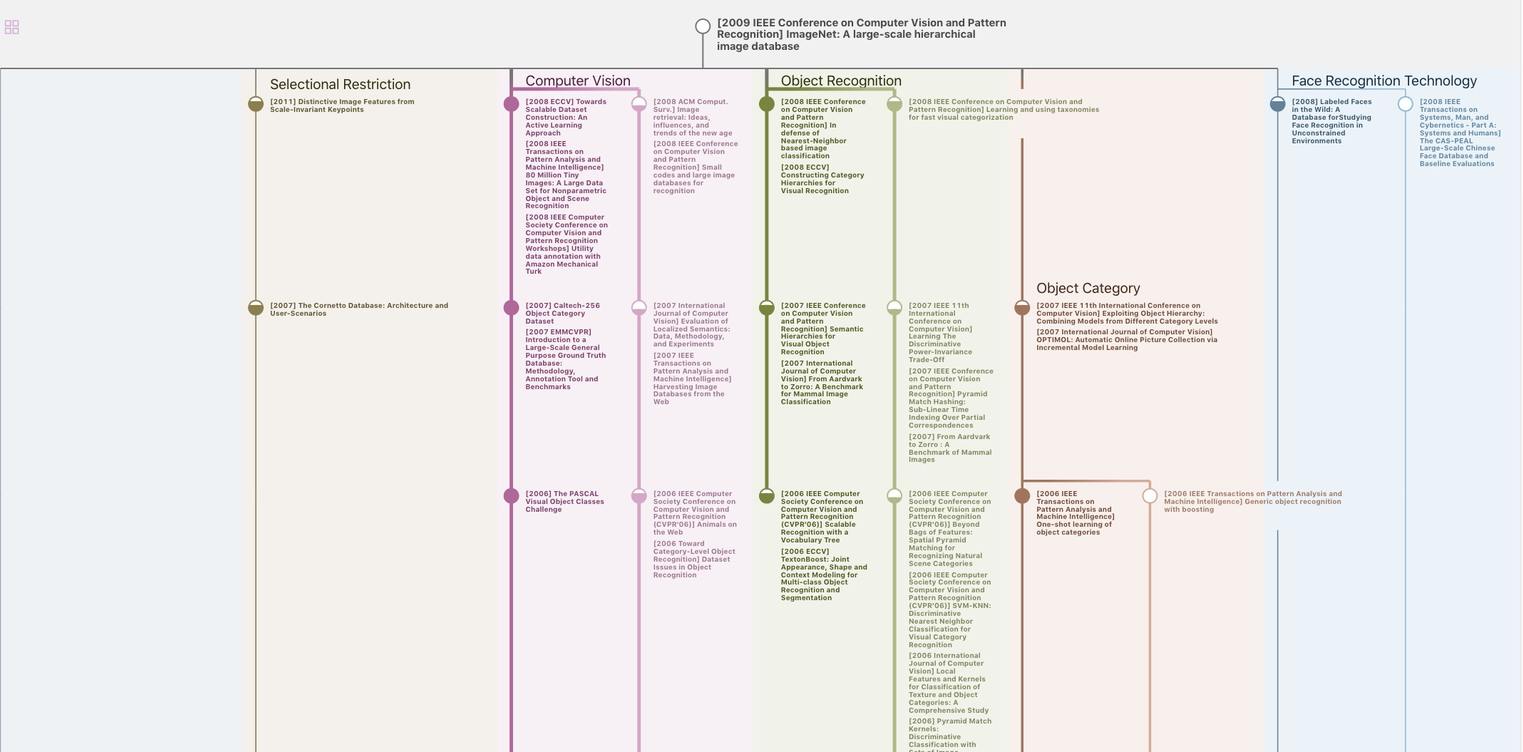
生成溯源树,研究论文发展脉络
Chat Paper
正在生成论文摘要