CT Image-Based Radiomic Analysis for Detecting PD-L1 Expression Status in Bladder Cancer Patients
Academic Radiology(2024)
摘要
Rationale and Objectives
The role of Programmed death-ligand 1 (PD-L1) expression is crucial in guiding immunotherapy selection. This study aims to develop and evaluate a radiomic model, leveraging Computed Tomography (CT) imaging, with the objective of predicting PD-L1 expression status in patients afflicted with bladder cancer.
Materials and Methods
The study encompassed 183 subjects diagnosed with histologically confirmed bladder cancer, among which the PD-L1(+) cohort constituted 60.1% of the total population. Stratified random sampling was utilized at a 7:3 ratio. We employed five diverse machine learning algorithms—Decision Tree, Random Forest, Linear Support Vector Classification, Support Vector Machine, and Logistic Regression—to establish radiomic models on the training dataset. These models endeavored to predict PD-L1 expression status premised on radiomic features derived from region-of-interest segmentation. Subsequent to this, the predictive performance of these models was examined on a validation set employing the receiver operating characteristic (ROC) curve. The DeLong test was utilized to contrast ROC curves, thereby pinpointing the model with superior predictive accuracy.
Results
16 features were chosen for the model construction. All five models revealed strong performance in the training set (AUC, 0.920–1) and commendable predictive ability in the validation set (AUC, 0.753–0.766). As per the DeLong test, no statistically significant disparities were observed among any of the models (P > 0.05) in the validation set. Additional verification through the calibration curve and decision curve analysis indicated that the Logistic Regression model exhibited extraordinary precision and practicality.
Conclusion
Our machine learning model, grounded on radiomic features, demonstrated its proficiency in accurately distinguishing bladder cancer patients with high PD-L1 expression. Future research, incorporating more exhaustive datasets, could potentially augment the predictive efficiency of radiomic algorithms, thereby advancing their clinical utility.
更多查看译文
关键词
Radiomics,PD-L1,Bladder Cancer,CT Imaging,Prediction Model
AI 理解论文
溯源树
样例
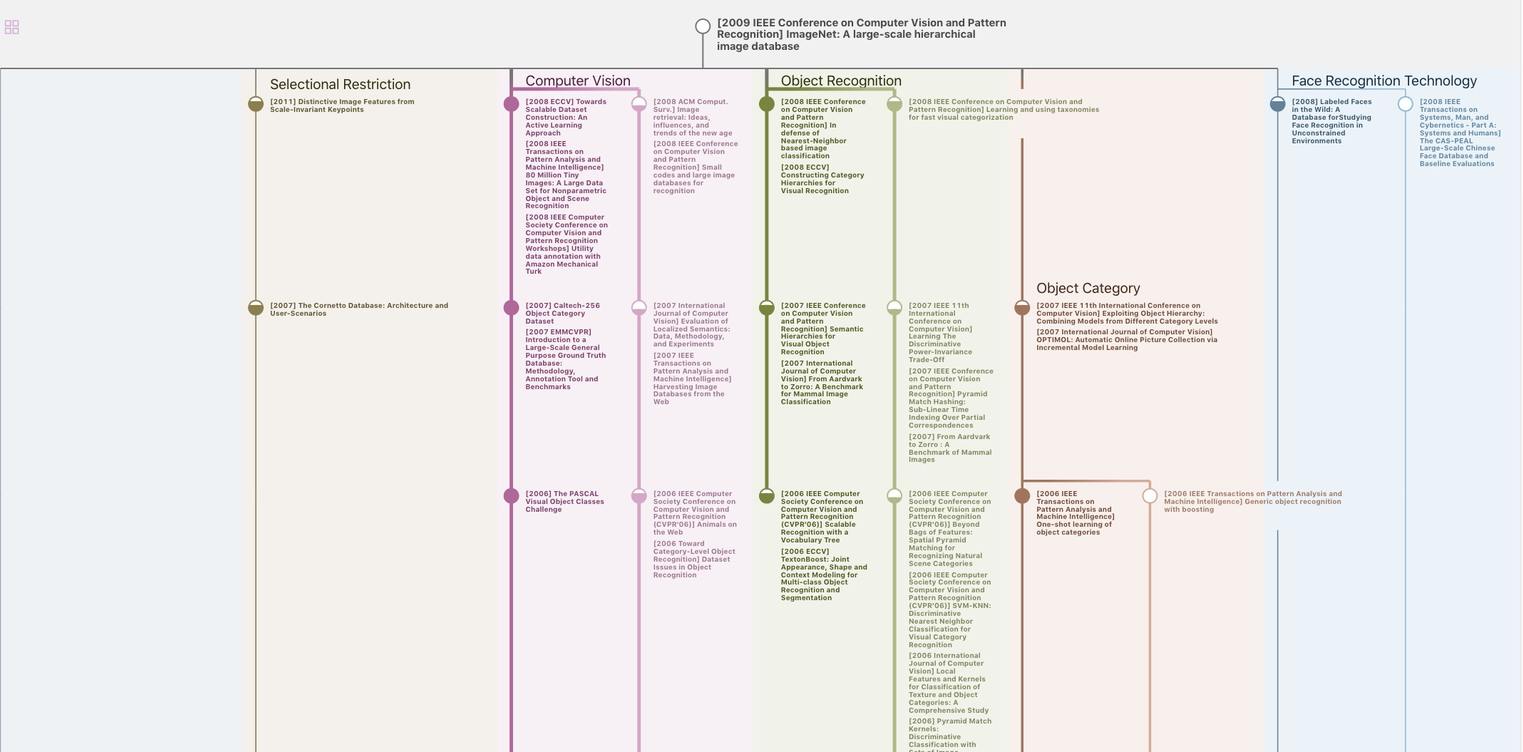
生成溯源树,研究论文发展脉络
Chat Paper
正在生成论文摘要