Zanthoxylum Infructescence Detection Based on Adaptive Density Clustering
Journal of agricultural engineering(2024)
Abstract
Infructescence detection during the early fruiting stage is a necessary preliminary work to estimate the yield of Zanthoxylum. The purpose of this research is to detect and quantify the infructescences on the images of early fruit-bearing branches of Zanthoxylum which are collected in the natural environment. Thus, a machine vision-based algorithm for detecting Zanthoxylum infructescences is proposed, which contains of two phases. The first is to segment fruit-like and non-fruit region from Zanthoxylum branch image by extracting the Histogram of Oriented Gradient (HOG) feature map and Excess Green minus Excess Red (ExGR) index to obtain the fruits of Zanthoxylum infructescences. The second is to identify fruits adaptively and roughly in accordance with the density of their position distribution, then an optimization model is established to merge rough clusters and the optimal clustering result is obtained. Experiments with normal samples demonstrate that the proposed approach receives a Precision of 96.67%, a Recall of 91.07%, and an F1-score of 0.93. Compared with ADPC-kNN, DBSCAN and OPTICS, the results reveal that the proposed algorithm has an improved robustness and achieves higher Recall and F1-score. Meanwhile, its competitiveness is displayed in the experiments with deep learning-based methods. It can adaptively detect the infructescences of Zanthoxylum branch images, and the experiments prove that it is effective.
MoreTranslated text
Key words
Adaptive density clustering,infructescence detection,yield estimation,Zanthoxylum
AI Read Science
Must-Reading Tree
Example
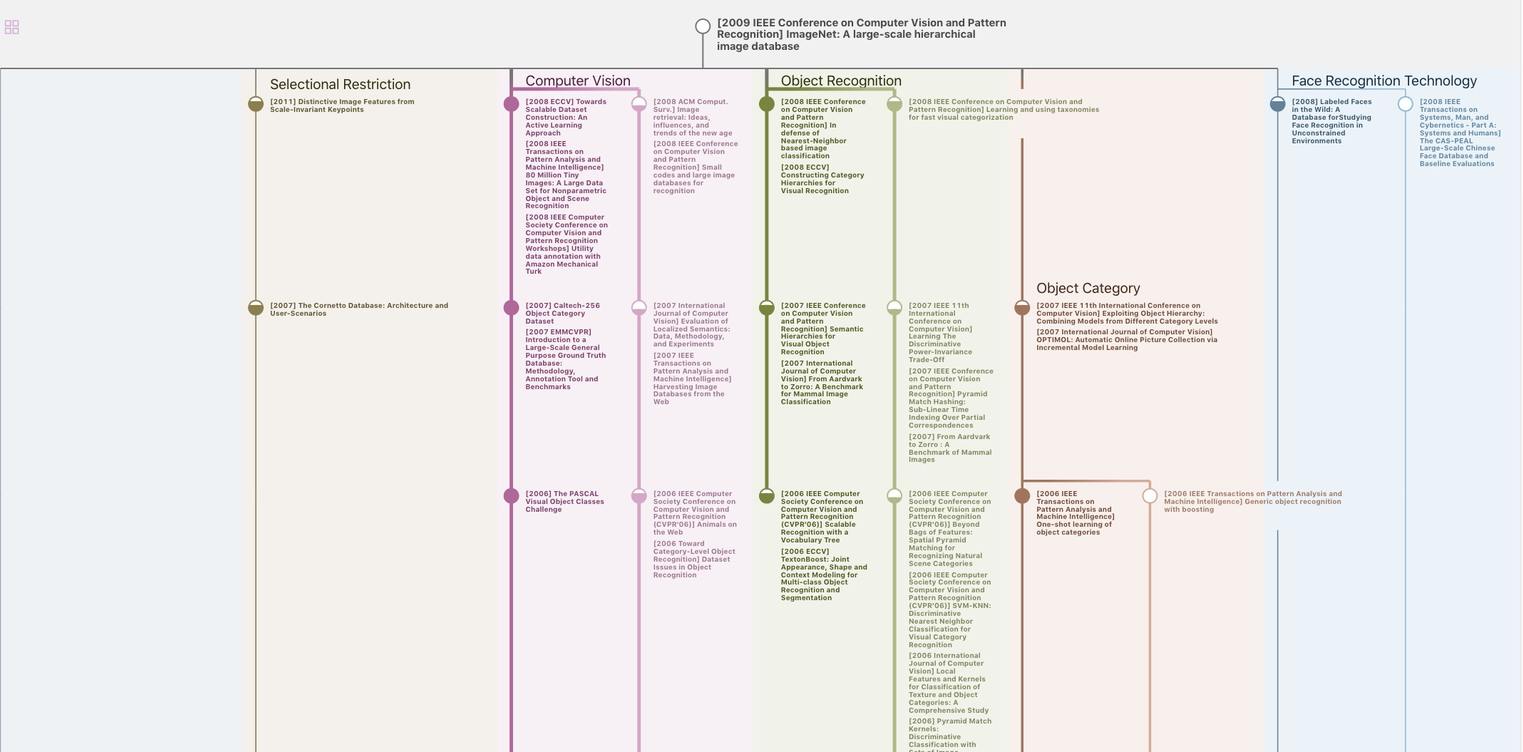
Generate MRT to find the research sequence of this paper
Chat Paper
Summary is being generated by the instructions you defined