EVE: Efficient Vision-Language Pre-training with Masked Prediction and Modality-Aware MoE
Proceedings of the AAAI Conference on Artificial Intelligence(2024)
Abstract
Building scalable vision-language models to learn from diverse, multimodal data remains an open challenge. In this paper, we introduce an Efficient Vision-languagE foundation model, namely EVE, which is one unified multimodal Transformer pre-trained solely by one unified pre-training task. Specifically, EVE encodes both vision and language within a shared Transformer network integrated with modality-aware sparse Mixture-of-Experts (MoE) modules, which capture modality-specific information by selectively switching to different experts. To unify pre-training tasks of vision and language, EVE performs masked signal modeling on image-text pairs to reconstruct masked signals, i.e., image pixels and text tokens, given visible signals. This simple yet effective pre-training objective accelerates training by 4x compared to the model pre-trained with Image-Text Contrastive and Image-Text Matching losses. Owing to the combination of the unified architecture and pre-training task, EVE is easy to scale up, enabling better downstream performance with fewer resources and faster training speed. Despite its simplicity, EVE achieves state-of-the-art performance on various vision-language downstream tasks, including visual question answering, visual reasoning, and image-text retrieval.
MoreTranslated text
AI Read Science
Must-Reading Tree
Example
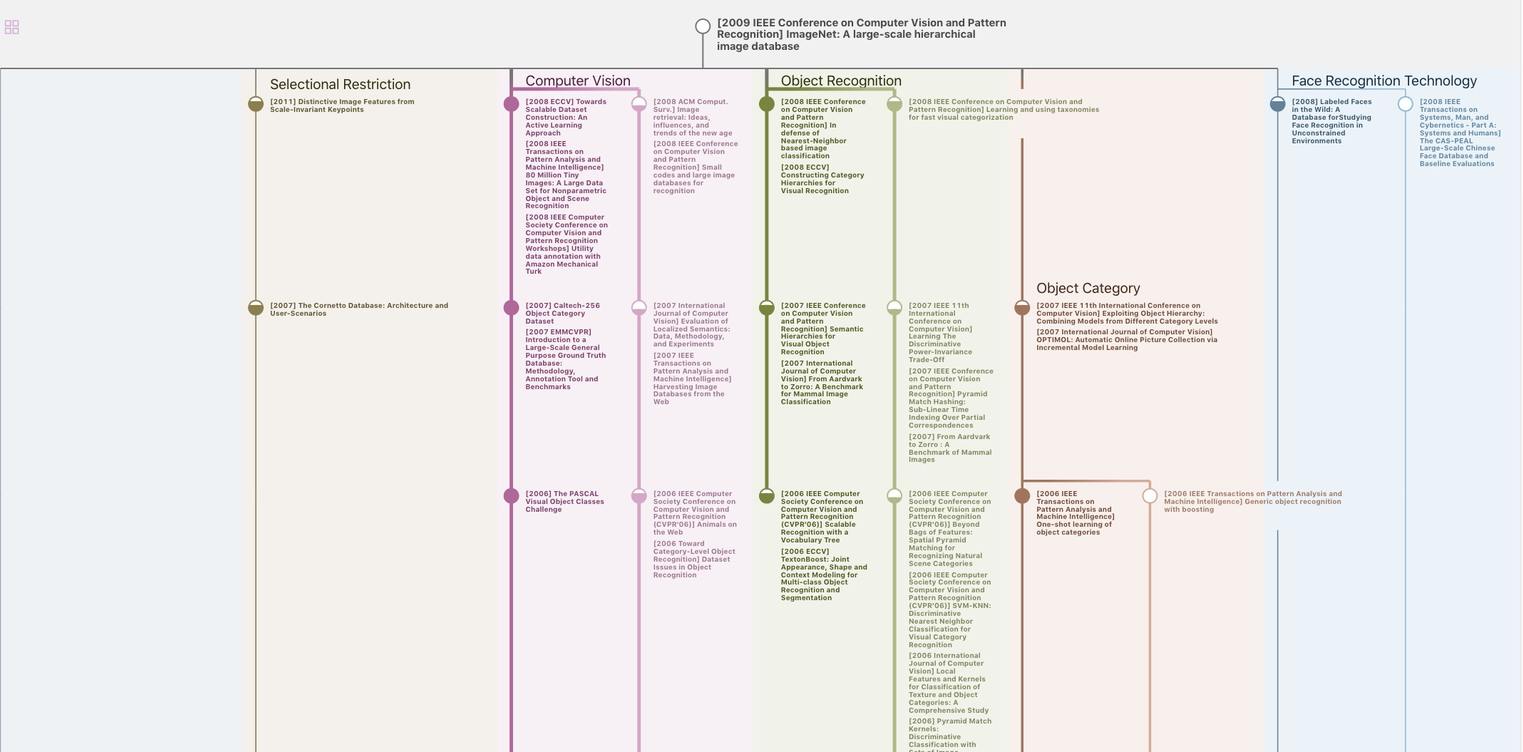
Generate MRT to find the research sequence of this paper
Chat Paper
Summary is being generated by the instructions you defined