A block-randomized stochastic method with importance sampling for CP tensor decomposition
Advances in Computational Mathematics(2024)
摘要
One popular way to compute the CANDECOMP/PARAFAC (CP) decomposition of a tensor is to transform the problem into a sequence of overdetermined least squares subproblems with Khatri-Rao product (KRP) structure involving factor matrices. In this work, based on choosing the factor matrix randomly, we propose a mini-batch stochastic gradient descent method with importance sampling for those special least squares subproblems. Two different sampling strategies are provided. They can avoid forming the full KRP explicitly and computing the corresponding probabilities directly. The adaptive step size version of the method is also given. For the proposed method, we present its theoretical properties and comprehensive numerical performance. The results on synthetic and real data show that our method is effective and efficient, and for unevenly distributed data, it performs better than the corresponding one in the literature.
更多查看译文
关键词
CP decomposition,Importance sampling,Stochastic gradient descent,Khatri-Rao product,Randomized algorithm,Adaptive algorithm,15A69,68W20,90C52
AI 理解论文
溯源树
样例
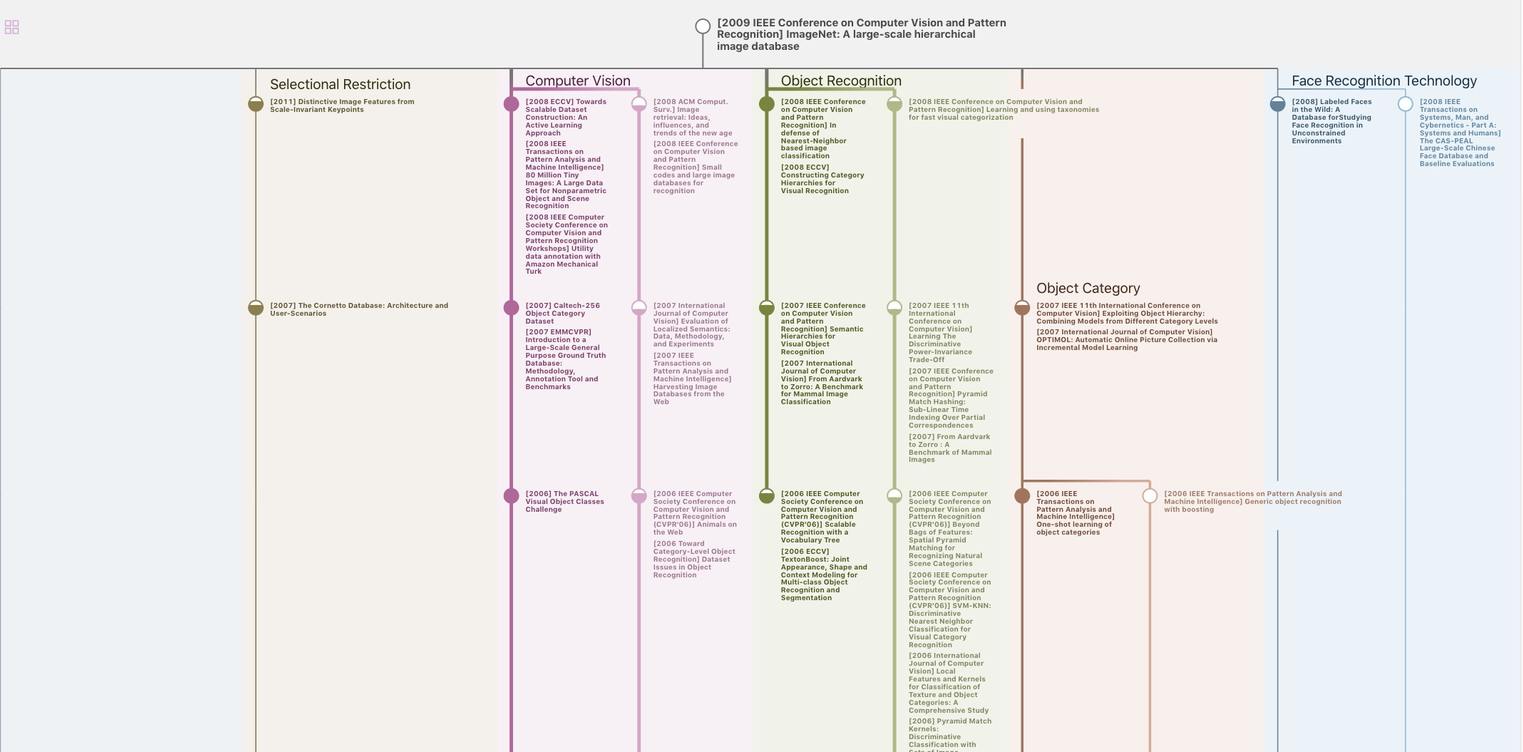
生成溯源树,研究论文发展脉络
Chat Paper
正在生成论文摘要