Addressing the Band Gap Problem with a Machine-Learned Exchange Functional
arxiv(2024)
摘要
The systematic underestimation of band gaps is one of the most fundamental
challenges in semilocal density functional theory (DFT). In addition to
hindering the application of DFT to predicting electronic properties, the band
gap problem is intimately related to self-interaction and delocalization
errors, which make the study of charge transfer mechanisms with DFT difficult.
In this work, we present two key innovations to address the band gap problem.
First, we design an approach for machine learning density functionals based on
Gaussian processes to explicitly fit single-particle energy levels. Second, we
introduce novel nonlocal features of the density matrix that are expressive
enough to fit these single-particle levels. Combining these developments, we
train a machine-learned functional for the exact exchange energy that predicts
molecular energy gaps and reaction energies of a wide range of molecules in
excellent agreement with reference hybrid DFT calculations. In addition, while
being trained solely on molecular data, our model predicts reasonable formation
energies of polarons in solids, showcasing its transferability and robustness.
Our approach generalizes straightforwardly to full exchange-correlation
functionals, thus paving the way to the design of novel state-of-the-art
functionals for the prediction of electronic properties of molecules and
materials.
更多查看译文
AI 理解论文
溯源树
样例
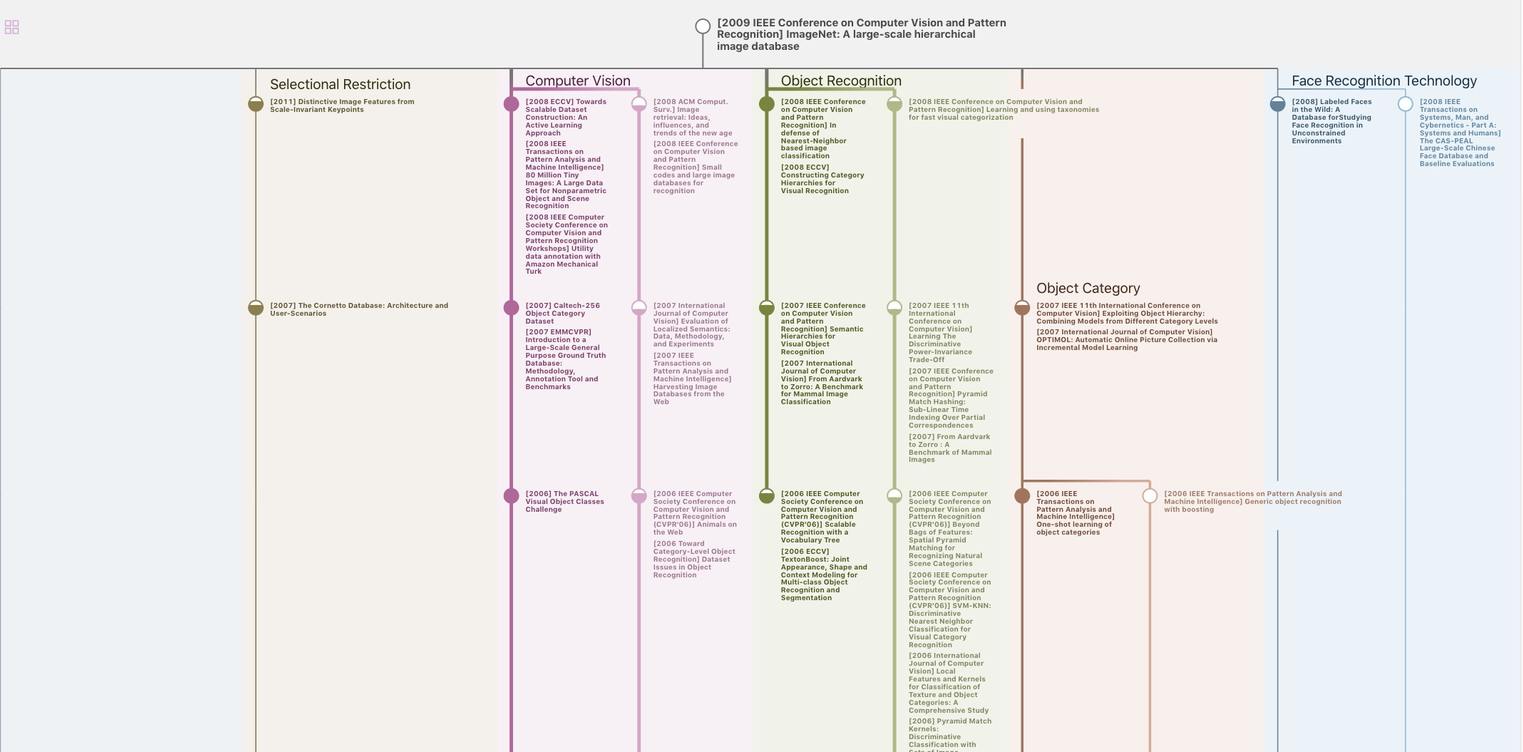
生成溯源树,研究论文发展脉络
Chat Paper
正在生成论文摘要