Exploring the Use of Long Short-Term Memory for Time Series Analysis
2024 International Conference on Optimization Computing and Wireless Communication (ICOCWC)(2024)
摘要
This abstract explores the applications of long short-time period reminiscence (LSTM) for time collection evaluation. Time series analysis is essential in many fields to explore past activities and predict future trends. LSTM networks are artificial neural networks that approach records in temporal order. They can gain knowledge of lengthy-time period dependencies and may be used for sequence prediction and forecasting obligations. This abstract discusses how LSTM networks can be carried out for time series evaluation, the benefits and challenges of such networks, and potential programs for using such networks for time series evaluation. The abstract concludes with a quick outline of continuing research in this area. This paper examines the usage of long short-time period reminiscence (LSTM) for time collection analysis. We examine using LSTMs for time collection evaluation and how they may be used to become aware of and distinguish between developments and styles in time series datasets. We gift a top-level view of LSTM architectures and discuss the effectiveness of their use of them for time collection analysis. We additionally speak about the ability of programs using LSTMs for time series analysis, including predicting stock market movement and personal conduct. ultimately, we talk about the demanding situations that want to be addressed to make LSTM fashions extra effective for time series analysis..
更多查看译文
AI 理解论文
溯源树
样例
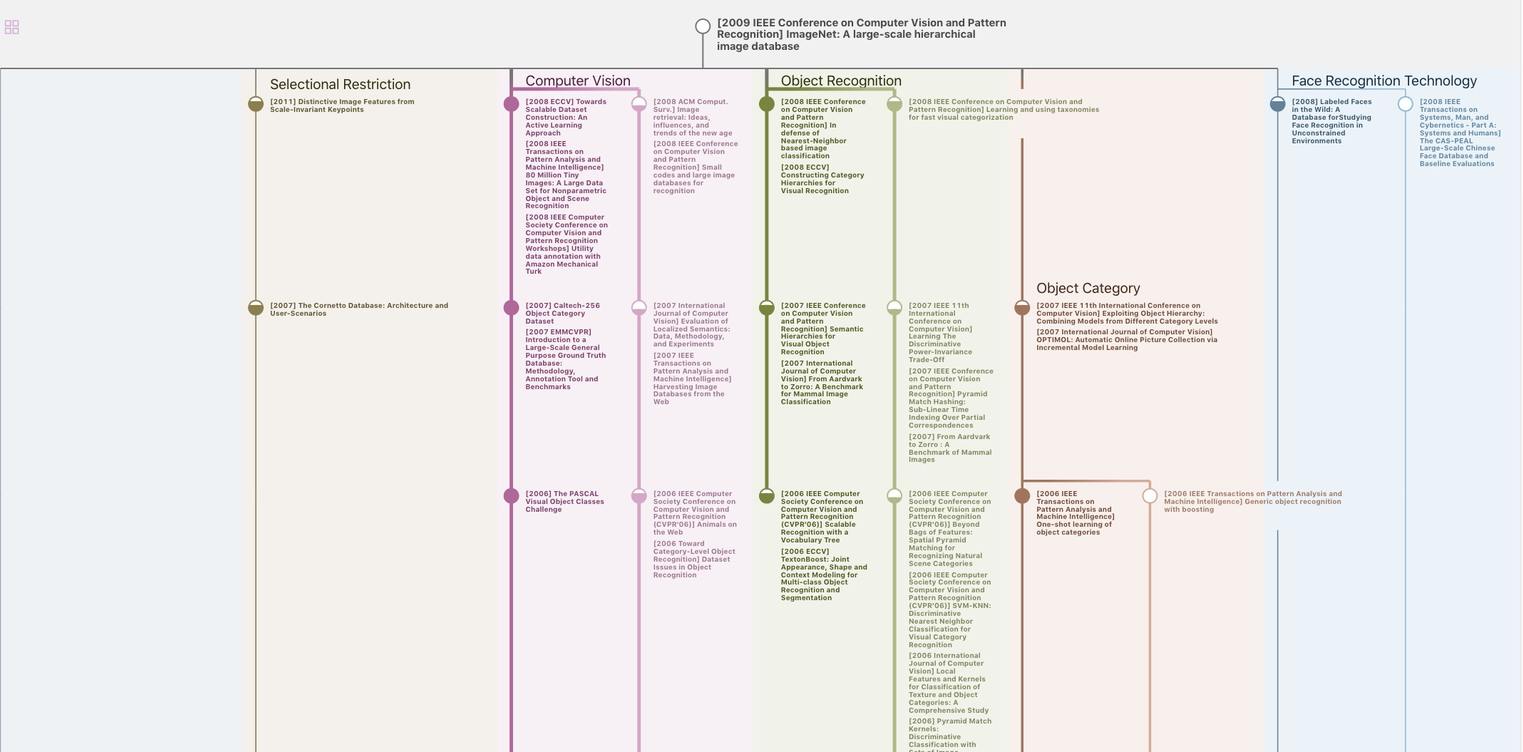
生成溯源树,研究论文发展脉络
Chat Paper
正在生成论文摘要