A Deep Dive into Electra: Transfer Learning for Fine-Grained Text Classification on SST-2
2023 6th International Seminar on Research of Information Technology and Intelligent Systems (ISRITI)(2023)
摘要
In the dynamic landscape of Natural Language Processing (NLP), a transformative revolution is underway, marked by the rapid evolution and proliferation of pre-trained language models that have irrevocably reshaped the boundaries of text understanding and classification. Electra, a language model introduced by Clark et al. in 2020, stands out as an innovation with a distinctive pre-training approach, particularly in fine-grained text classification tasks. This research aims to rigorously evaluate Electra's performance in fine-grained text classification, primarily focusing on sentiment analysis tasks within the SST-2 dataset. Additionally, the study seeks to provide invaluable guidance to researchers and practitioners by elucidating the most effective fine-tuning strategies and configuration settings. The results highlight the significance of gradual fine-tuning, with increased layer unfreezing positively impacting model accuracy. This underscores Electra's vast potential for NLP tasks and the importance of thoughtful fine-tuning processes.
更多查看译文
关键词
Natural Language Processing (NLP),Electra,Fine-tuning,Transfer Learning,Model Layer Unfreezing,Text Classification
AI 理解论文
溯源树
样例
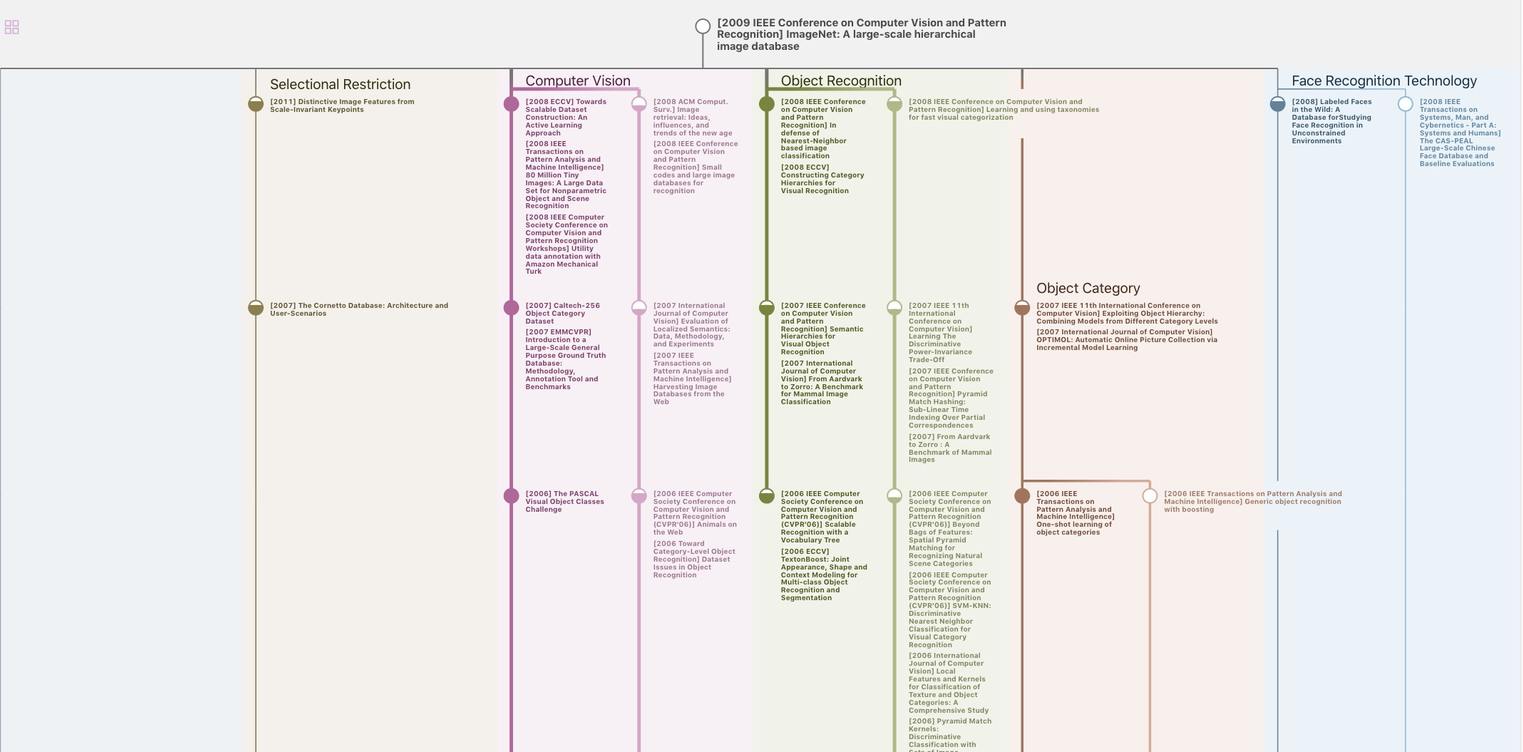
生成溯源树,研究论文发展脉络
Chat Paper
正在生成论文摘要