Chernoff Information As a Privacy Constraint for Adversarial Classification
arXiv (Cornell University)(2024)
摘要
This work studies a privacy metric based on Chernoff information,Chernoff differential privacy, due to its significance incharacterization of classifier performance. Adversarial classification, as anyother classification problem is built around minimization of the (average orcorrect detection) probability of error in deciding on either of the classes inthe case of binary classification. Unlike the classical hypothesis testingproblem, where the false alarm and mis-detection probabilities are handledseparately resulting in an asymmetric behavior of the best error exponent, inthis work, we focus on the Bayesian setting and characterize the relationshipbetween the best error exponent of the average error probability andε-differential privacy. Accordingly, we re-derive Chernoffdifferential privacy in terms of ε-differential privacy using theRadon-Nikodym derivative and show that it satisfies the composition property.Subsequently, we present numerical evaluation results, which demonstrates thatChernoff information outperforms Kullback-Leibler divergence as a function ofthe privacy parameter ε, the impact of the adversary's attack andglobal sensitivity for the problem of adversarial classification in Laplacemechanisms.
更多查看译文
关键词
Adversarial Examples
AI 理解论文
溯源树
样例
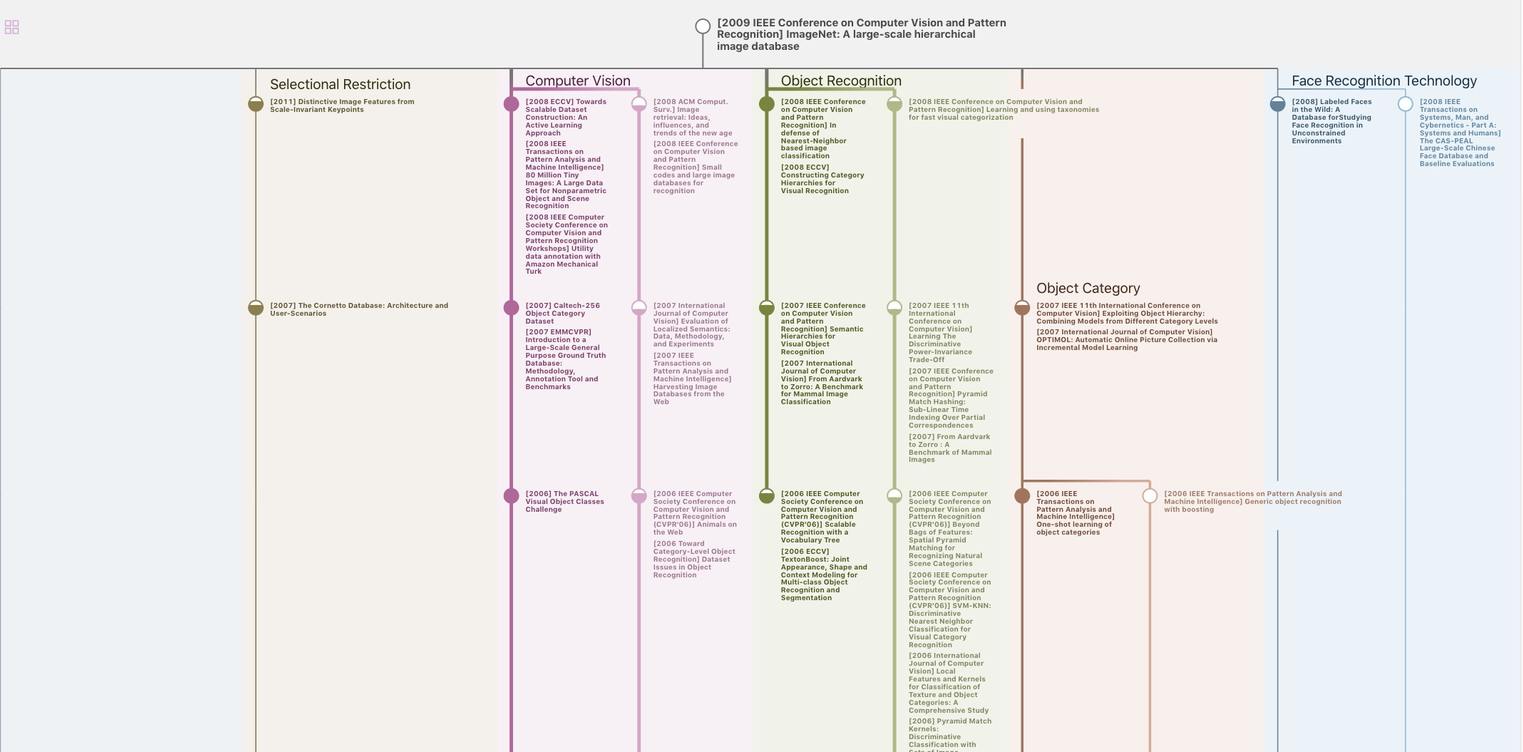
生成溯源树,研究论文发展脉络
Chat Paper
正在生成论文摘要