Alleviating Confounding Effects with Contrastive Learning in Recommendation
ADVANCES IN INFORMATION RETRIEVAL, ECIR 2024, PT II(2024)
Abstract
Recently, there has been a growing interest in mitigating the bias effects in recommendations using causal inference. However, Rubin's potential outcome framework may produce inaccurate estimates in real-world scenarios due to the presence of hidden confounders. In addition, existing works adopting the Pearl causal graph framework tend to focus on specific types of bias (e.g., selection bias, popularity bias, exposure bias) instead of directly mitigating the impact of hidden confounders. Motivated by the aforementioned limitations, in this paper, we formulate the recommendation task as a causal graph with unobserved/unmeasurable confounders. We present a novel causality-based architecture called Multi-behavior Debiased Contrastive Collaborative Filtering (MDCCL) and apply the front-door adjustment for intervention. We leverage a pre-like behavior such as clicking an item (i.e., a behavior occurred before the target behavior such as purchasing) to mitigate the bias effects. Additionally, we design a contrastive loss that also provides a debiasing effect benefiting the recommendation. An empirical study on three real-world datasets validates that our proposed method successfully outperforms nine state-of-the-art baselines. Code and the datasets will be available at https://github.com/queenjocey/MDCCL.
MoreTranslated text
Key words
Recommender system,causal inference,debiased recommendation,contrastive learning
AI Read Science
Must-Reading Tree
Example
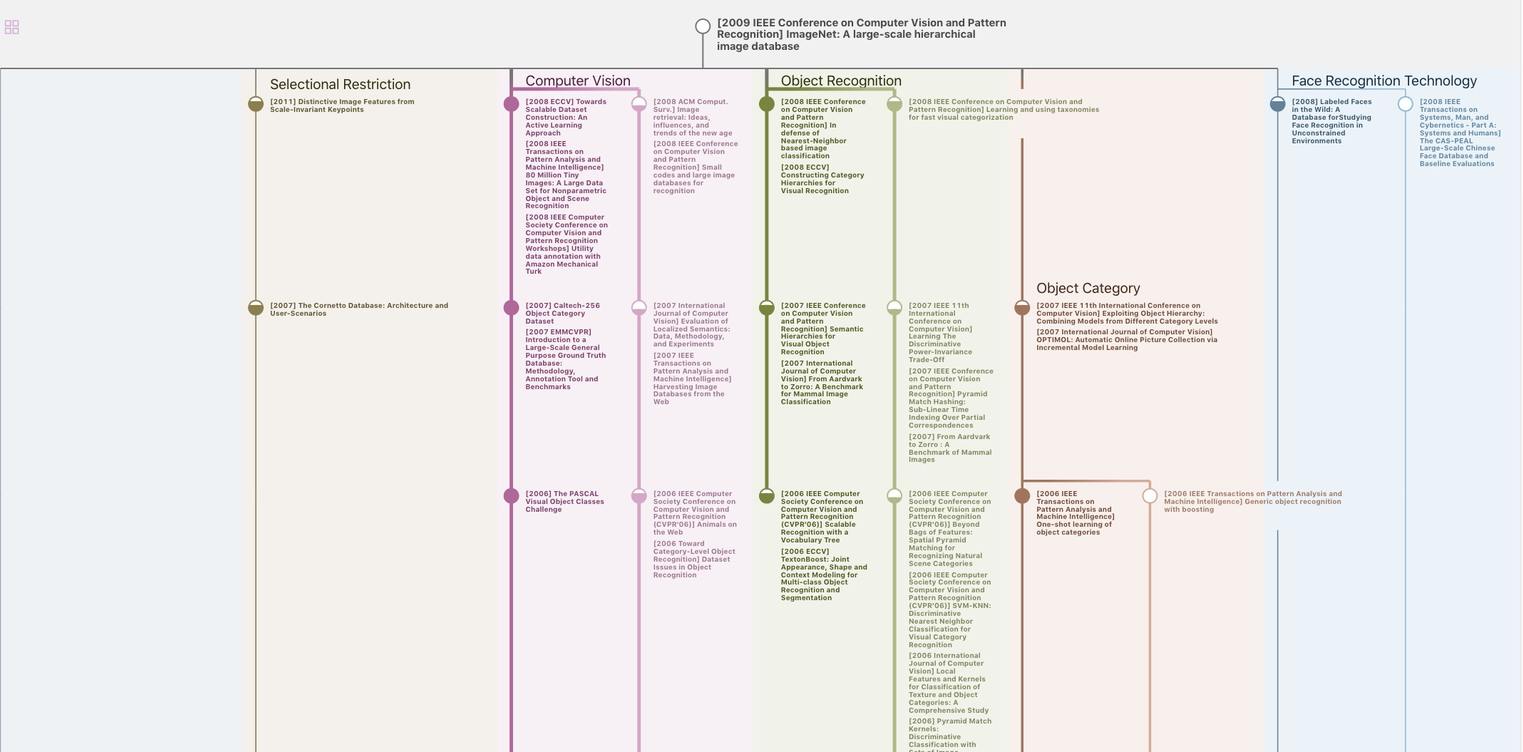
Generate MRT to find the research sequence of this paper
Chat Paper
Summary is being generated by the instructions you defined