Active Discovering New Slots for Task-Oriented Conversation
IEEE-ACM TRANSACTIONS ON AUDIO SPEECH AND LANGUAGE PROCESSING(2024)
摘要
Existing task-oriented conversational systems heavily rely on domain ontologies with pre-defined slots and candidate values. In practical settings, these prerequisites are hard to meet, due to the emerging new user requirements and ever-changing scenarios. To mitigate these issues for better interaction performance, there are efforts working towards detecting out-of-vocabulary values or discovering new slots under unsupervised or semi-supervised learning paradigms. However, overemphasizing on the conversation data patterns alone induces these methods to yield noisy and arbitrary slot results. To facilitate the pragmatic utility, real-world systems tend to provide a stringent amount of human labeling quota, which offers an authoritative way to obtain accurate and meaningful slot assignments. Nonetheless, it also brings forward the high requirement of utilizing such quota efficiently. Hence, we formulate a general new slot discovery task in an information extraction fashion and incorporate it into an active learning framework to realize human-in-the-loop learning. Specifically, we leverage existing language tools to extract value candidates where the corresponding labels are further leveraged as weak supervision signals. Based on these, we propose a bi-criteria selection scheme which incorporates two major strategies, namely, uncertainty-based and diversity-based sampling to efficiently identify terms of interest. We conduct extensive experiments on several public datasets and compare with a bunch of competitive baselines to demonstrate the effectiveness of our method.
更多查看译文
关键词
New slot discovery,task-oriented conversation,active learning,language processing
AI 理解论文
溯源树
样例
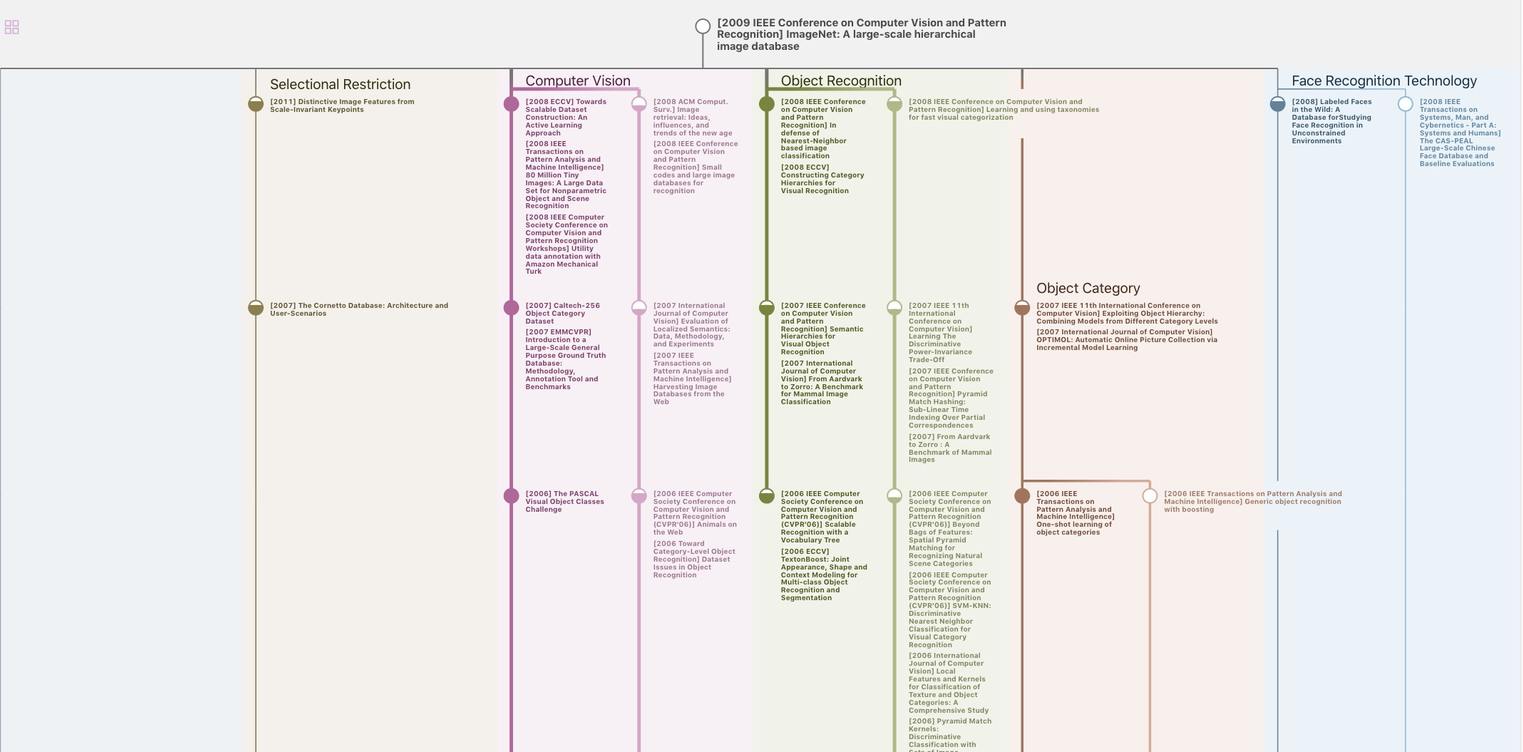
生成溯源树,研究论文发展脉络
Chat Paper
正在生成论文摘要