Adaptive Stochastic Gradient Algorithm for Black-box Multi-Objective Learning
ICLR 2024(2024)
Abstract
Multi-objective optimization (MOO) has become an influential framework for various machine learning problems, including reinforcement learning and multi-task learning. In this paper, we study the black-box multi-objective optimization problem, where we aim to optimize multiple potentially conflicting objectives with function queries only. To address this challenging problem and find a Pareto optimal solution or the Pareto stationary solution,
we propose a novel adaptive stochastic gradient algorithm for black-box MOO, called ASMG.
Specifically, we use the stochastic gradient approximation method to obtain the gradient for the distribution parameters of the Gaussian smoothed MOO with function queries only. Subsequently, an adaptive weight is employed to aggregate all stochastic gradients to optimize all objective functions effectively.
Theoretically, we explicitly provide the connection between the original MOO problem and the corresponding Gaussian smoothed MOO problem and prove the convergence rate for the proposed ASMG algorithm in both convex and non-convex scenarios.
Empirically, the proposed ASMG method achieves competitive performance on multiple numerical benchmark problems. Additionally, the state-of-the-art performance on the black-box multi-task learning problem demonstrates the effectiveness of the proposed ASMG method.
MoreTranslated text
Key words
Multi-Objective Optimization,Black-Box Optimization,Black-Box Multi-Objective Optimization
AI Read Science
Must-Reading Tree
Example
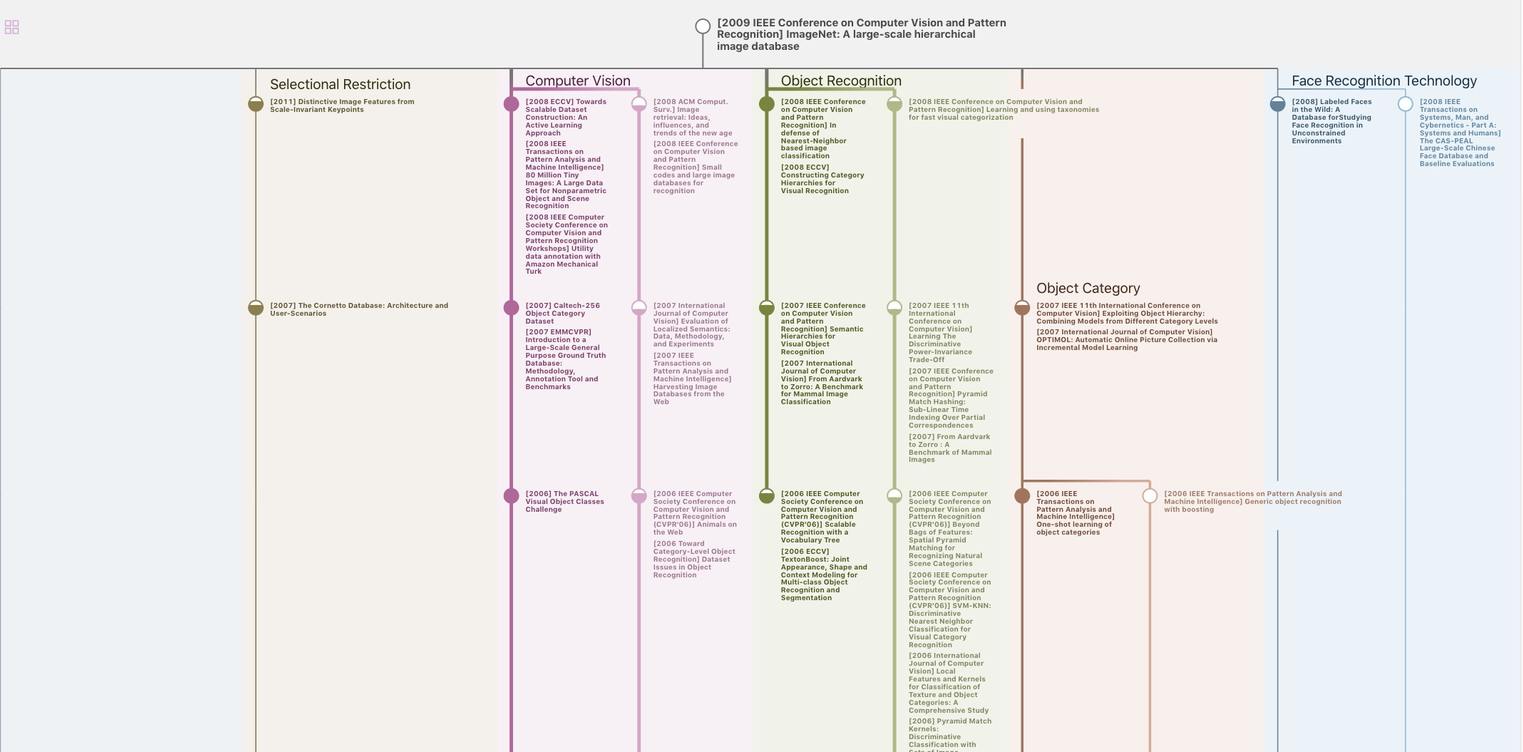
Generate MRT to find the research sequence of this paper
Chat Paper
Summary is being generated by the instructions you defined