Probabilistic classification of surrounding rock mass of tunnels based on fully Bayesian Gaussian process classification (fB-GPC) with consideration of influencing factors selection
GEOLOGICAL JOURNAL(2024)
摘要
Rock mass classification is of great significance in guiding the design and construction of tunnels, which is often determined through single-factor or multi-factor classification methods based on some readily available rock indices, for example, dynamic elastic modulus, degree of tectonization, and so forth. Although these methods work reasonably well in some cases, they tend to lead to inaccurate classification results due to the pre-selected main factor or function forms, especially for tunnels with complex geological conditions. Alternatively, the classification may be achieved with the aid of some machine learning (ML) methods, which are data-driven, hence bypassing pre-determination of the mathematical function forms of the main factor(s). Note although these ML methods perform well in the classification of surrounding rock mass, they generally employ all measurements of readily available rock indices as input variables, which might lead to excessive model complexity and a potential decrease in generalization performance. In this paper, a fully Bayesian Gaussian process classification (fB-GPC) approach is proposed for probabilistic classification of surrounding rock mass, which is data-driven and can select the optimal combination of main factors that affect rock mass classification. A real-life example is used to illustrate and validate the proposed approach. Results show that the proposed fB-GPC method performs very well in determination of the most important factor for classification of surrounding rock mass, with an area under the receiver operating characteristic curve (AUC) of around 0.98. A sensitivity study is also performed to systematically examine the accuracy and robustness of the proposed approach in this study.
更多查看译文
关键词
Bayesian approach,data-driven method,feature selection,probabilistic geotechnical site characterization,tunnelling engineering
AI 理解论文
溯源树
样例
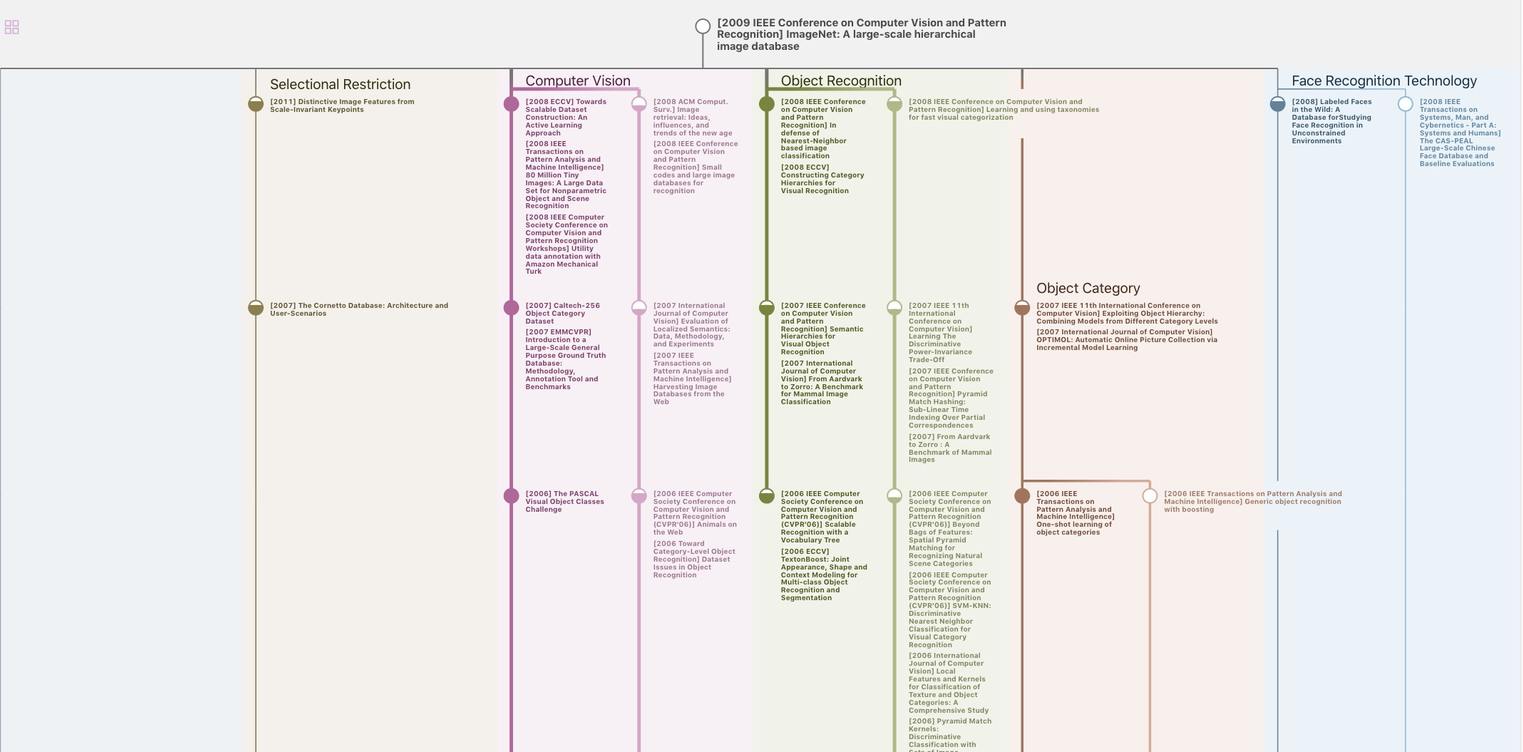
生成溯源树,研究论文发展脉络
Chat Paper
正在生成论文摘要