Learning cooperative strategies in StarCraft through role-based monotonic value function factorization
ELECTRONIC RESEARCH ARCHIVE(2024)
摘要
StarCraft is a popular real-time strategy game that has been widely used as a research platform for artificial intelligence. Micromanagement refers to the process of making each unit perform appropriate actions separately, depending on the current state in the the multi-agent system comprising all of the units, i.e., the fine-grained control of individual units for common benefit. Therefore, cooperation between different units is crucially important to improve the joint strategy. We have selected multi-agent deep reinforcement learning to tackle the problem of micromanagement. In this paper, we propose a method for learning cooperative strategies in StarCraft based on role-based montonic value function factorization (RoMIX). RoMIX learns roles based on the potential impact of each agent on the multi-agent task; it then represents the action value of a role in a mixed way based on monotonic value function factorization. The final value is calculated by accumulating the action value of all roles. The role-based learning improves the cooperation between agents on the team, allowing them to learn the joint strategy more quickly and efficiently. In addition, RoMIX can also reduce storage resources to a certain extent. Experiments show that RoMIX can not only solve easy tasks, but it can also learn better cooperation strategies for more complex and difficult tasks.
更多查看译文
关键词
Q-learning,multi-agent reinforcement learning,machine learning,artificial intelligence,StarCraft multi-agent challenge
AI 理解论文
溯源树
样例
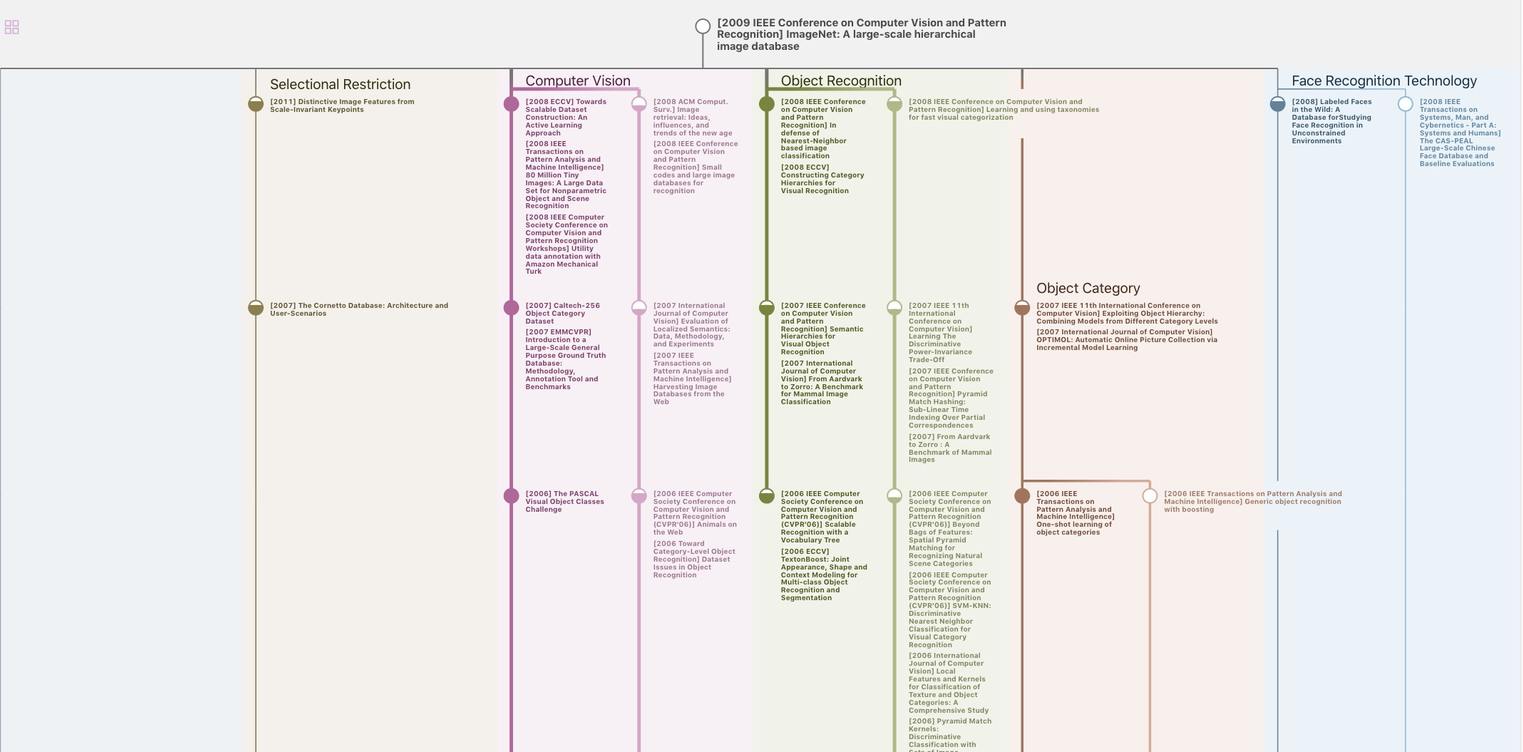
生成溯源树,研究论文发展脉络
Chat Paper
正在生成论文摘要