DOCTOR: Dynamic On-Chip Temporal Variation Remediation Toward Self-Corrected Photonic Tensor Accelerators
Journal of lightwave technology(2024)
摘要
Photonic computing has emerged as a promising solution for accelerating computation-intensive artificial intelligence (AI) workloads, offering unparalleled speed and energy efficiency, especially in resource-limited, latency-sensitive edge computing environments. However, the deployment of analog photonic tensor accelerators encounters reliability challenges due to hardware noise and environmental variations. While off-chip noise-aware training and on-chip training have been proposed to enhance the variation tolerance of optical neural accelerators with moderate , static noise, we observe a notable performance degradation over time due to temporally drifting variations, which requires a real-time, in-situ calibration mechanism. To tackle this challenging reliability issues, for the first time , we propose a lightweight dynamic on-chip remediation framework, dubbed DOCTOR , providing adaptive, in-situ accuracy recovery against temporally drifting noise. The DOCTOR framework intelligently monitors the chip status using adaptive probing and performs fast in-situ training-free calibration to restore accuracy when necessary. Recognizing nonuniform spatial variation distributions across devices and tensor cores, we also propose a variation-aware architectural remapping strategy to avoid executing critical tasks on noisy devices. Extensive experiments show that our proposed framework can guarantee sustained performance under drifting variations with 34% higher accuracy and 2-3 orders-of-magnitude lower overhead compared to state-of-the-art on-chip training methods. Our code is open-sourced at link.
更多查看译文
关键词
Photonic computing,optical neural networks,thermal variation,robustness,on-chip calibration
AI 理解论文
溯源树
样例
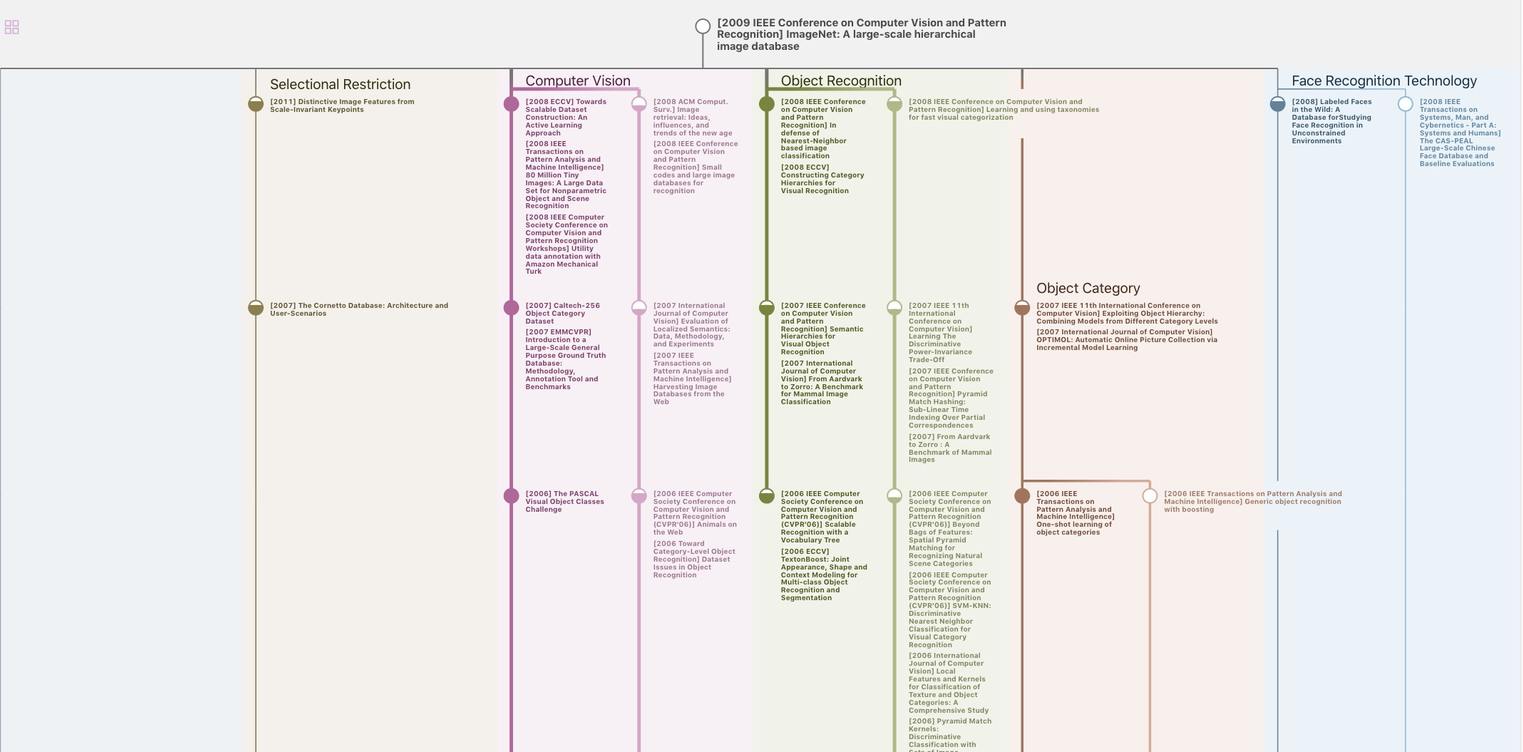
生成溯源树,研究论文发展脉络
Chat Paper
正在生成论文摘要