Feature Collusion Attack on PMU Data-driven Event Classification.
IEEE PES Innovative Smart Grid Technologies Conference(2024)
Abstract
Event classification is a critical task to ensure the reliability of the power system. Recent developments in event classification methods leverage data-driven techniques with fine-grained Phasor Measurement Units (PMU) data. However, the existing event classification methods are vulnerable to different types of adversarial attacks that can significantly degrade the event classification performance. In this paper, we evaluate the vulnerability of the classification models against feature collision attacks on PMU data. Feature collusion attack leverages a surrogate model to learn the victim’s classification model which in turn makes it a plausible attack strategy for both black-box and white-box settings. Specifically, this attack strategy undermines the accuracy of the classification models by crafting poisonous samples that share common features with benign samples which in turn changes the decision boundaries of the classification models. The experimental results on real-world PMU data in a black-box setting show that generating and adding poisonous samples into the model training dataset can significantly degrade the accuracy of current event classification methods.
MoreTranslated text
Key words
Phasor Measurement Units (PMUs),Adversarial Attacks,Data Poisoning Attacks,and Feature Collusion Attack
AI Read Science
Must-Reading Tree
Example
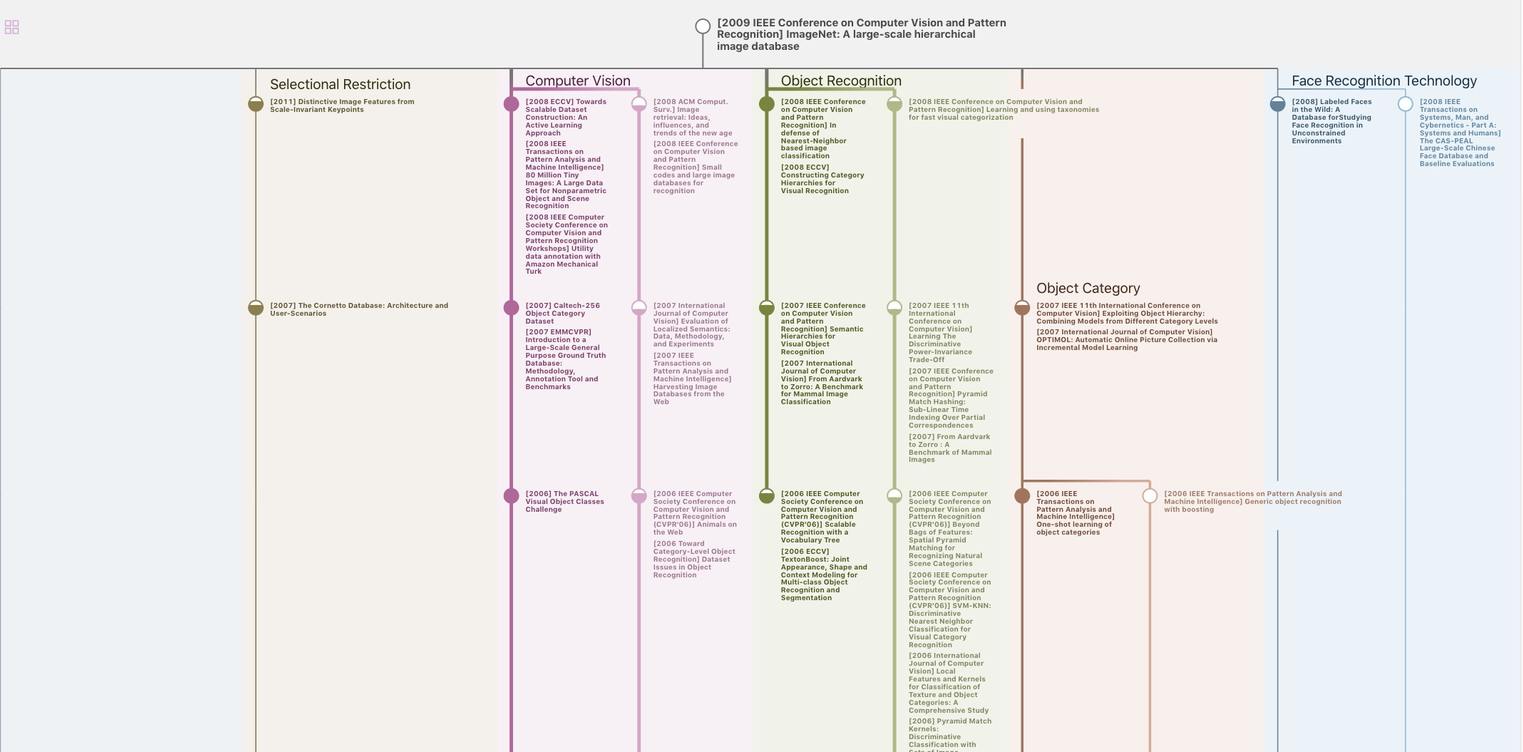
Generate MRT to find the research sequence of this paper
Chat Paper
Summary is being generated by the instructions you defined