paper Discriminating quartz host rock based on its trace element chemistry using machine learning-a new tool for sedimentary provenance studies
Chemical Geology(2024)
Abstract
Quartz is one of the most abundant mineral in the continental crust, occurring in a wide variety of igneous, metamorphic, and sedimentary rocks. It incorporates several trace elements such as Ti, Al, Li, Ge, B, and P in its structure during crystallization/precipitation from magma/fluid, which can potentially be used as petrogenetic indicators. Being physically and chemically resilient during sedimentary recycling, detrital quartz can retain the signatures of the physicochemical environment of their formation. Earlier studies have mostly attempted to discriminate quartz from magmatic and magmatic-hydrothermal systems using bivariate or ternary discrimination diagrams. In this study, we developed three robust machine learning (ML) methods (XGBoost, LightGBM, and CatBoost models) to discriminate quartz from a wide variety of magmatic/magmatic-hydrothermal host rocks based on their trace element chemistry. For this purpose, we compiled published 11,267 trace element analyses of quartz grains considering 50 trace elements from 14 different host rocks, namely A-, S-, I- type granitoids and pegmatites, A-, I- type volcanic rocks, A-, S- type- granite greisen, hydrothermal veins associated with A-, S-, I- type intrusions, and metamorphic rocks. The three ML classifiers can successfully discriminate quartz from the aforementioned groups with an average accuracy of similar to 95%. Quartz hosted in each system can be correctly predicted with >75% classification accuracy, except for I -type pegmatite. The Shapley Additive exPlanations (SHAP) algorithm, which measures the contribution of each element to the output predicted by the ML models, indicates that Ti, Li, Ge, Al, B, and P exert the highest control on the ML classifiers. We propose our ML models as a new tool for constraining sedimentary provenance and demonstrate the usefulness of our approach using the trace element data of detrital quartz from sediments in the catchment area of the Bega River in the Lachlan Fold Belt, Australia.
MoreTranslated text
Key words
Quartz,Trace elements,Machine learning models,Host rock discrimination,Sedimentary provenance
AI Read Science
Must-Reading Tree
Example
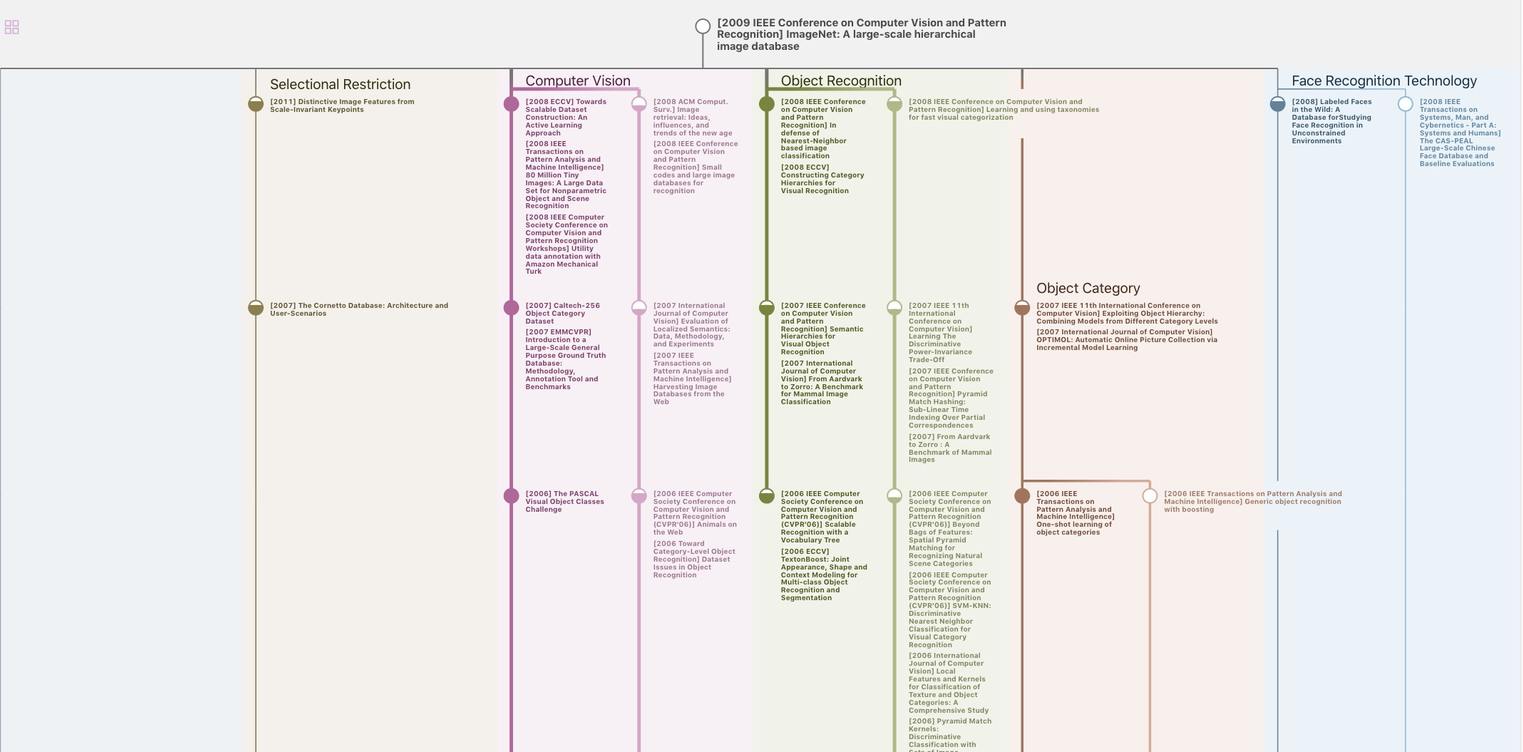
Generate MRT to find the research sequence of this paper
Chat Paper
Summary is being generated by the instructions you defined