Sources of High-Energy Astrophysical Neutrinos
arXiv · High Energy Astrophysical Phenomena(2024)
Deutsches Elektronen-Synchrotron DESY
Abstract
We discuss recent results in neutrino astronomy and their implications forthe cosmic-ray acceleration in relativistic outflows, such as in ActiveGalactic Nuclei (AGN) jets, Gamma-Ray Bursts (GRBs), and Tidal DisruptionEvents (TDEs). We especially focus on challenges at the interface to particleacceleration which can be inferred from the multi-messenger context, such asthe paradigm that the sources power the Ultra-High-Energy Cosmic Rays (UHECRs).We demonstrate that both AGN blazars (in the context of neutrino observations)and GRBs (as UHECR sources in the context of neutrino-non-observations) pointtowards acceleration spectra harder than E^-2, or relatively high minimalcosmic-ray injection energies, to meet the respective energy budgetrequirements. We furthermore speculate that neutrino flares in AGN blazars maybe related to super-Eddington accretion flares, or that GRBs are powered bysignificantly higher kinetic energies than typically assumed in electromagneticmodels. For internal shock models, the UHECR paradigm for GRBs can only bemaintained in the light of neutrino stacking limits in multi-zone models. Whilerelativistic outflows in TDEs have become recently interesting per se andmodels for the neutrino emission from jetted TDEs exist, a direct connectionbetween TDE jets pointing in our direction and astrophysical neutrinos has notbeen identified yet.
MoreTranslated text
PDF
View via Publisher
AI Read Science
AI Summary
AI Summary is the key point extracted automatically understanding the full text of the paper, including the background, methods, results, conclusions, icons and other key content, so that you can get the outline of the paper at a glance.
Example
Background
Key content
Introduction
Methods
Results
Related work
Fund
Key content
- Pretraining has recently greatly promoted the development of natural language processing (NLP)
- We show that M6 outperforms the baselines in multimodal downstream tasks, and the large M6 with 10 parameters can reach a better performance
- We propose a method called M6 that is able to process information of multiple modalities and perform both single-modal and cross-modal understanding and generation
- The model is scaled to large model with 10 billion parameters with sophisticated deployment, and the 10 -parameter M6-large is the largest pretrained model in Chinese
- Experimental results show that our proposed M6 outperforms the baseline in a number of downstream tasks concerning both single modality and multiple modalities We will continue the pretraining of extremely large models by increasing data to explore the limit of its performance
Try using models to generate summary,it takes about 60s
Must-Reading Tree
Example
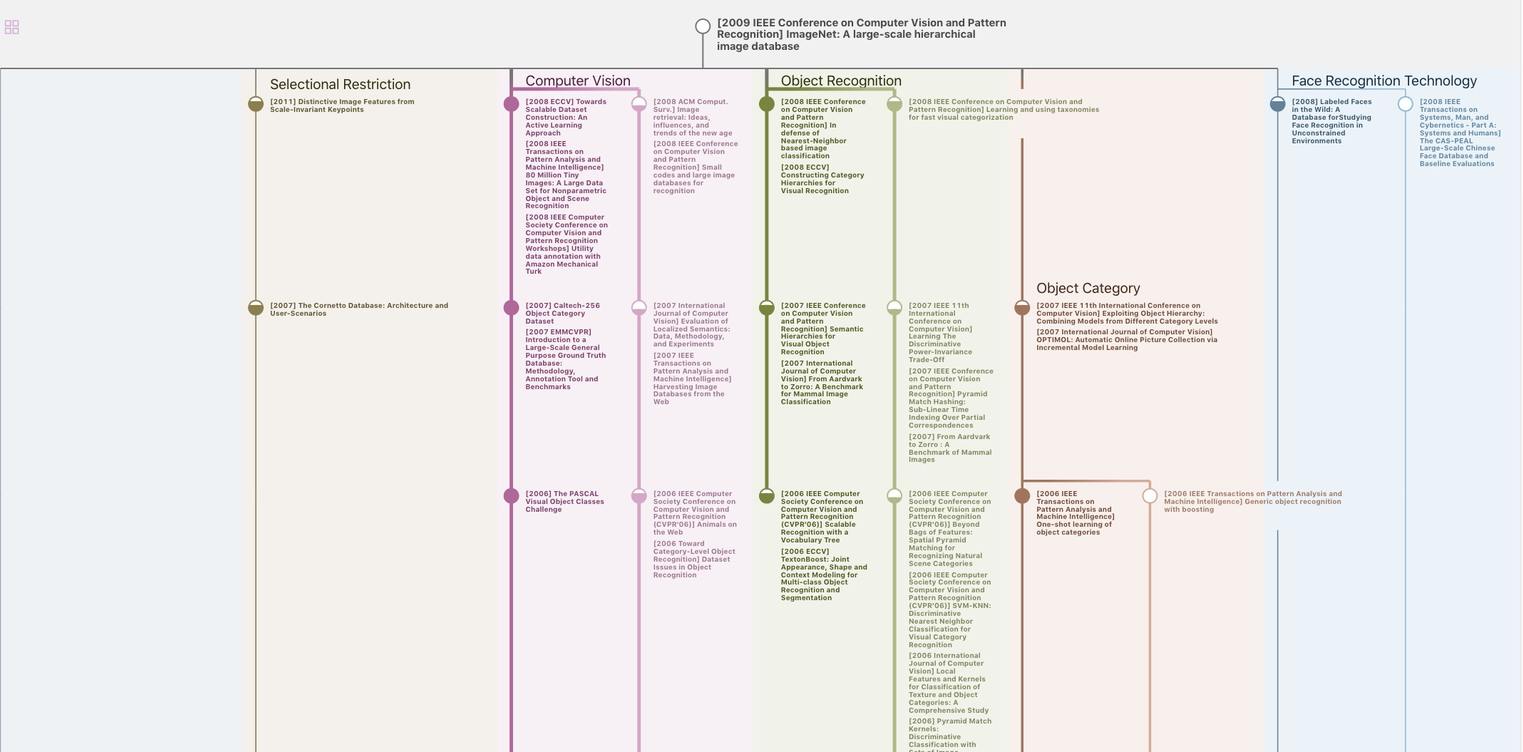
Generate MRT to find the research sequence of this paper
Data Disclaimer
The page data are from open Internet sources, cooperative publishers and automatic analysis results through AI technology. We do not make any commitments and guarantees for the validity, accuracy, correctness, reliability, completeness and timeliness of the page data. If you have any questions, please contact us by email: report@aminer.cn
Chat Paper
去 AI 文献库 对话