SAR Incremental Automatic Target Recognition Based on Mutual Information Maximization
IEEE GEOSCIENCE AND REMOTE SENSING LETTERS(2024)
摘要
To enable the synthetic aperture radar (SAR) automatic target recognition (ATR) system to continuously adapt to new recognition scenarios, it is necessary to equip the system with the ability to quickly update models. However, when these models learn new tasks, the knowledge of old tasks is quickly forgotten, a phenomenon known as catastrophic forgetting. The reason for catastrophic forgetting is that the model does not use the features of old tasks sufficiently. In this letter, an exemplar-free class incremental learning based on maximizing mutual information (CIL-MMI) is proposed to solve this problem. To effectively use the extracted features, CIL-MMI actively clusters features to maximize the mutual information (MI) between features and corresponding labels. The proposed method successfully avoids the distribution overlap caused by the small interclass differences and large intraclass variances inherent in SAR images. Experiments on the Moving and Stationary Target Acquisition and Recognition (MSTAR) dataset indicate that the proposed method outperforms state-of-the-art approaches, demonstrating improvements of 5.41%, 1.93%, and 2.47% at incremental steps 1, 2, and 3, respectively.
更多查看译文
关键词
Catastrophic forgetting,incremental learning,mutual information (MI)
AI 理解论文
溯源树
样例
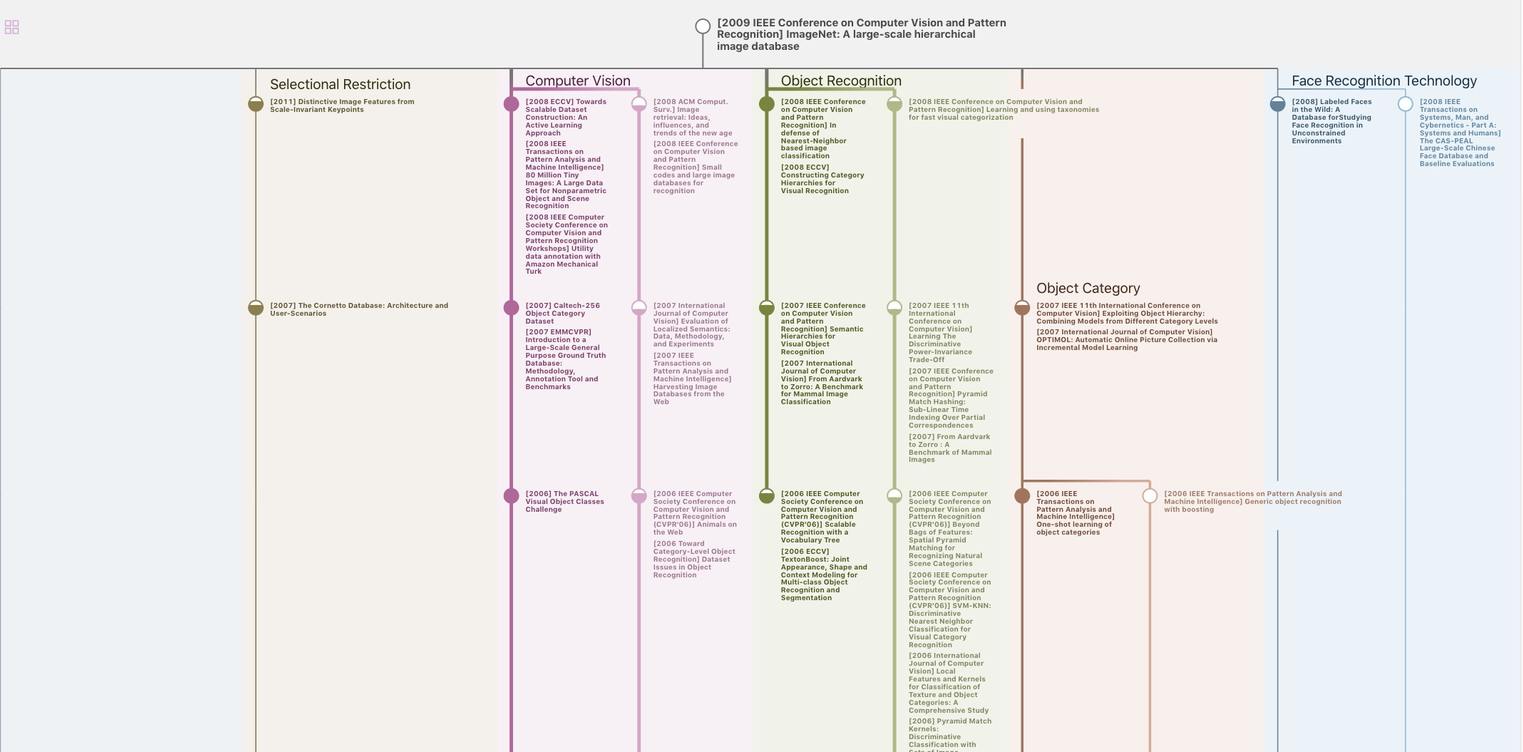
生成溯源树,研究论文发展脉络
Chat Paper
正在生成论文摘要