Probabilistic Contrastive Learning for Long-Tailed Visual Recognition.
IEEE TRANSACTIONS ON PATTERN ANALYSIS AND MACHINE INTELLIGENCE(2024)
Key words
Contrastive learning,long-tailed visual recognition,representation learning,semi-supervised learning,Contrastive learning,long-tailed visual recognition,representation learning,semi-supervised learning
AI Read Science
Must-Reading Tree
Example
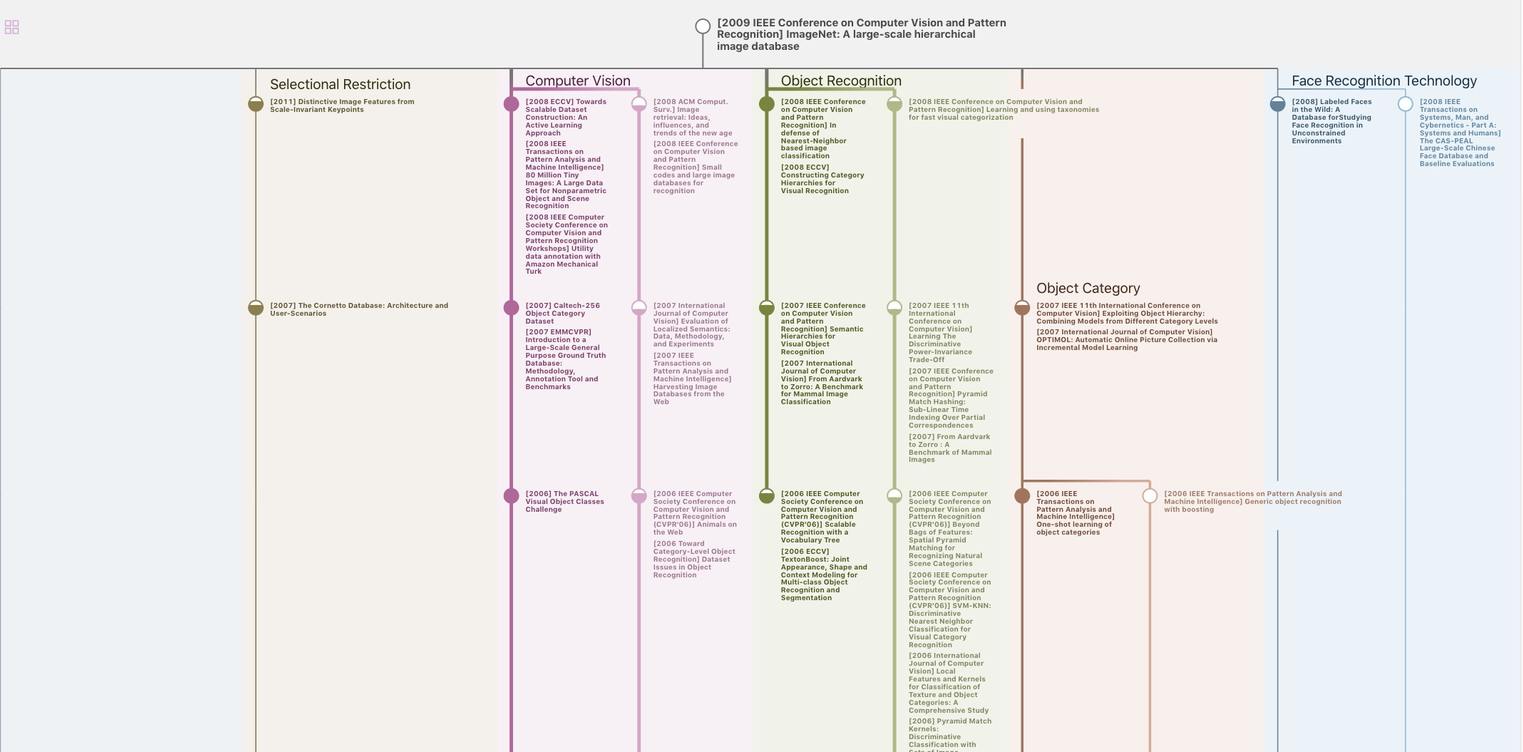
Generate MRT to find the research sequence of this paper
Chat Paper
Summary is being generated by the instructions you defined