Interactive spatiotemporal LSTM approach for enhanced industrial fault diagnosis
INDUSTRIAL LUBRICATION AND TRIBOLOGY(2024)
摘要
PurposeRotating machinery is a crucial component of large equipment, and detecting faults in it accurately is critical for reliable operation. Although fault diagnosis methods based on deep learning have been significantly developed, the existing methods model spatial and temporal features separately and then weigh them, resulting in the decoupling of spatiotemporal features.Design/methodology/approachThe authors propose a spatiotemporal long short-term memory (ST-LSTM) method for fault diagnosis of rotating machinery. The authors collected vibration signals from real rolling bearing and gearing test rigs for verification.FindingsThrough these two experiments, the authors demonstrate that machine learning methods still have advantages on small-scale data sets, but our proposed method exhibits a significant advantage due to the simultaneous modeling of the time domain and space domain. These results indicate the potential of the interactive spatiotemporal modeling method for fault diagnosis of rotating machinery.Originality/valueThe authors propose a ST-LSTM method for fault diagnosis of rotating machinery. The authors collected vibration signals from real rolling bearing and gearing test rigs for verification.
更多查看译文
关键词
Fault diagnosis,Deep predictive learning,Artificial intelligence,Rotating machinery
AI 理解论文
溯源树
样例
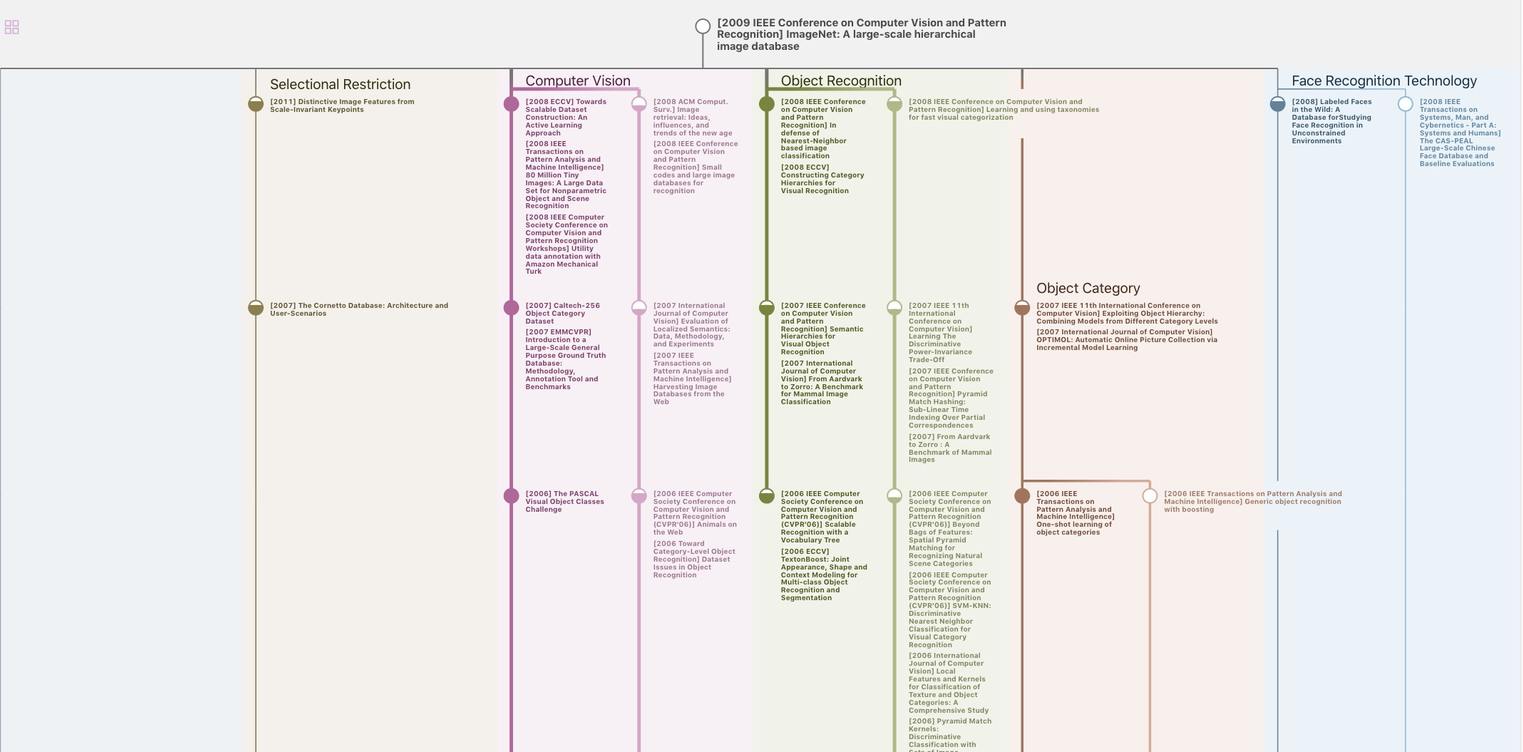
生成溯源树,研究论文发展脉络
Chat Paper
正在生成论文摘要