GATFormer: A Graph-based Transformer for Long-Term Forecasting of Traffic Overcrowding
2023 IEEE 26TH INTERNATIONAL CONFERENCE ON INTELLIGENT TRANSPORTATION SYSTEMS, ITSC(2023)
Abstract
Urban traffic forecasting is a critical issue in modern cities. In recent years, there has been a growing interest in using data from automated fare collection (AFC) systems to analyze passenger movement patterns and identify and predict travel behaviors. Urban transportation networks can be optimised using this analysis and by implementing machine learning algorithms. Accurately forecasting traffic flows (e.g., for identifying congested stations) is important for enhancing passenger satisfaction and safety. However, most existing methods analyze only station-level data for short-term flow forecasting, failing to consider the complex interconnected relations across the transportation network and the impact of long-term trends. In this paper, we propose a novel approach, GATFormer, that combines Graph Attention Networks (GAT) with a sequence-to-sequence attention mechanism to predict long-term overcrowding at traffic nodes (e.g., congestion at stations) and providing information to both transport network managers for policy decision making and to passengers for traffic guidance. Our method includes two parts: anticipation of both where and when overcrowding will take place. The proposed method is applied to real subway AFC data from both Suzhou and Hangzhou, China. The experimental results show that the model outperforms other baselines in long-term overcrowded station prediction.
MoreTranslated text
Key words
Transformer,Overcrowding,Long-term Forecasting,Attention Mechanism,Transportation Network,Traffic Flow,Urban Transport,Modern City,Graph Attention Network,Traffic Forecasting,Implementation Of Machine Learning Algorithms,Neural Network,Prediction Accuracy,Deep Neural Network,Big Data,Spatial Information,Short-term Memory,Long Short-term Memory,Recurrent Neural Network,Spatial Dependence,Traffic Prediction,Multi-head Attention Mechanism,Temporal Dependencies,Passenger Flow,Flow Prediction,Graph Neural Networks,Graph Convolutional Network,Gated Recurrent Unit,Urban Transportation Systems,LSTM-based Model
AI Read Science
Must-Reading Tree
Example
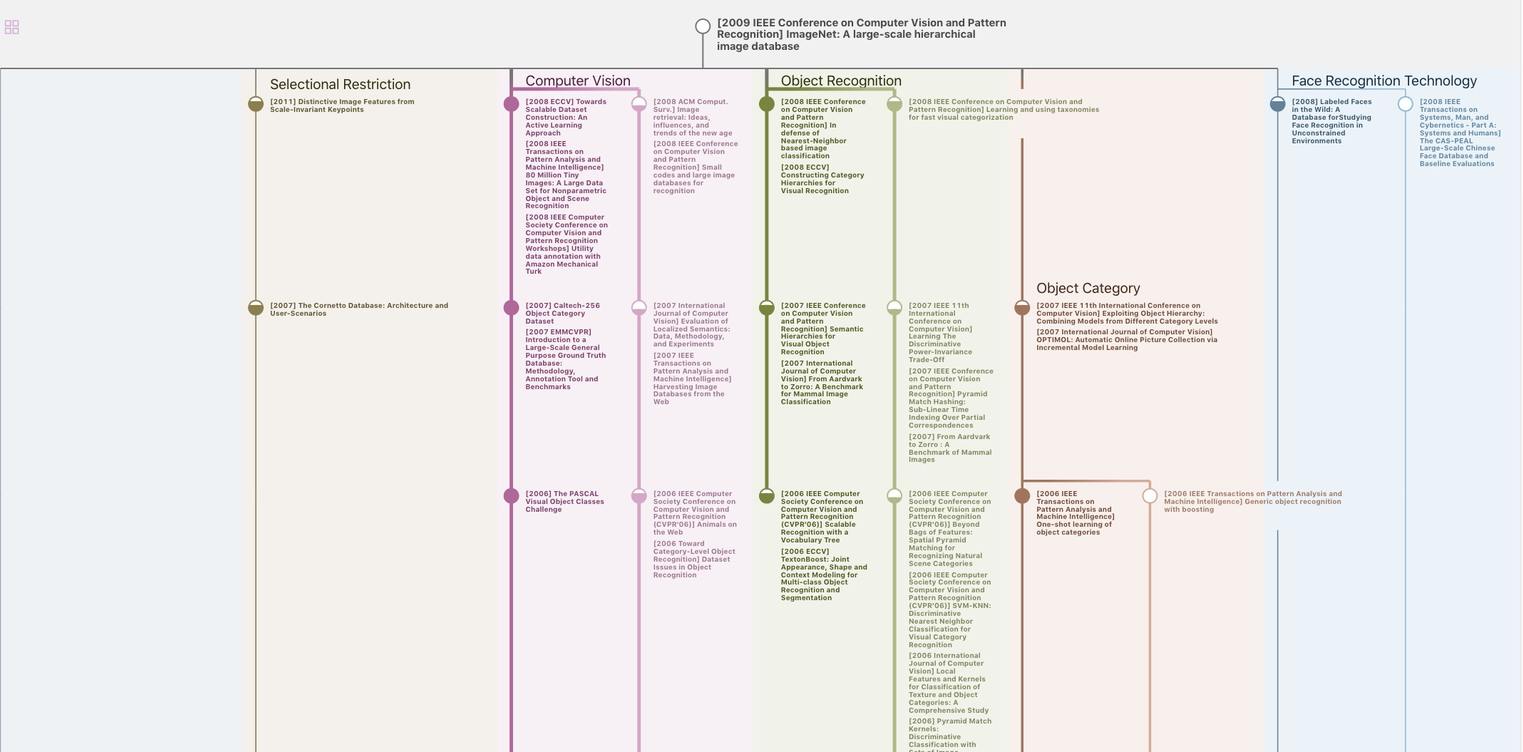
Generate MRT to find the research sequence of this paper
Chat Paper
Summary is being generated by the instructions you defined