Machine Learning Prediction of Molecular Binding Profiles on Metal-Porphyrin Via Spectroscopic Descriptors.
The journal of physical chemistry letters(2024)
摘要
The study of molecular adsorption is crucial for understanding various chemical processes. Spectroscopy offers a convenient and non-invasive way of probing structures of adsorbed states and can be used for real-time observation of molecular binding profiles, including both structural and energetic information. However, deciphering atomic structures from spectral information using the first-principles approach is computationally expensive and time-consuming because of the sophistication of recording spectra, chemical structures, and their relationship. Here, we demonstrate the feasibility of a data-driven machine learning approach for predicting binding energy and structural information directly from vibrational spectra of the adsorbate by using CO adsorption on iron porphyrin as an example. Our trained machine learning model is not only interpretable but also readily transferred to similar metal-nitrogen-carbon systems with comparable accuracy. This work shows the potential of using structure-encoded spectroscopic descriptors in machine learning models for the study of adsorbed states of molecules on transition metal complexes.
更多查看译文
AI 理解论文
溯源树
样例
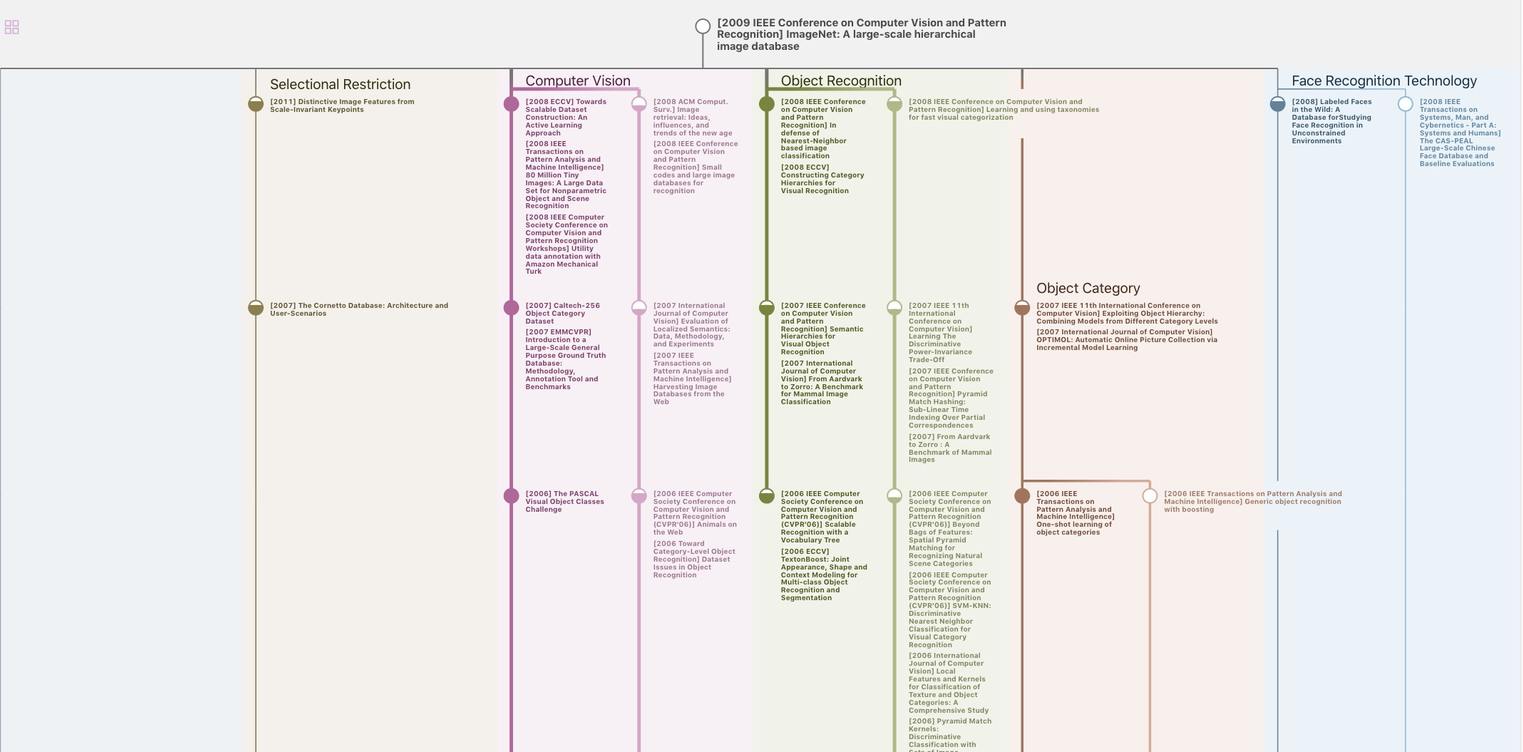
生成溯源树,研究论文发展脉络
Chat Paper
正在生成论文摘要