Estimation of Concrete Compressive Strength: A Neural Ordinary Differential Equation Approach
2023 International Conference on New Trends in Computational Intelligence (NTCI)(2023)
摘要
The 28-day concrete compressive strength(CCS) is one of the most significant indicators required by the construction industry. The existing methods require making concrete samples and conducting compressive strength tests through physical experiments in the sample age of 28 days. Waiting time of the experiments increases the cost in time and financial, which can not meet the actual demand. The advent of computational intelligence makes it possible to estimate the CCS. Many scholars use regression models to estimate the CCS. However, the formation of concrete strength is a complex dynamic system. The use of regression models can place limitations on estimates, limiting the potential for effects. It is inappropriate to simplify a complex dynamic system as a regression problem. Differential equations are widely used to describe dynamical systems. Neural ordinary differential equations draw on the idea of differential equations. But unlike ordinary differential equations in mathematics, the derivative functions of ordinary differential equations are modeled by neural networks. We proposed a novel approach to model the dynamical system of concrete. Furthermore, it provides a novel approach to estimating the 28-day CCS by modeling concrete dynamic systems based on Neural ODE. The experimental results show our approach achieves acceptable accuracy.
更多查看译文
关键词
Neural ODE,concrete,estimate strength,dynamic modeling
AI 理解论文
溯源树
样例
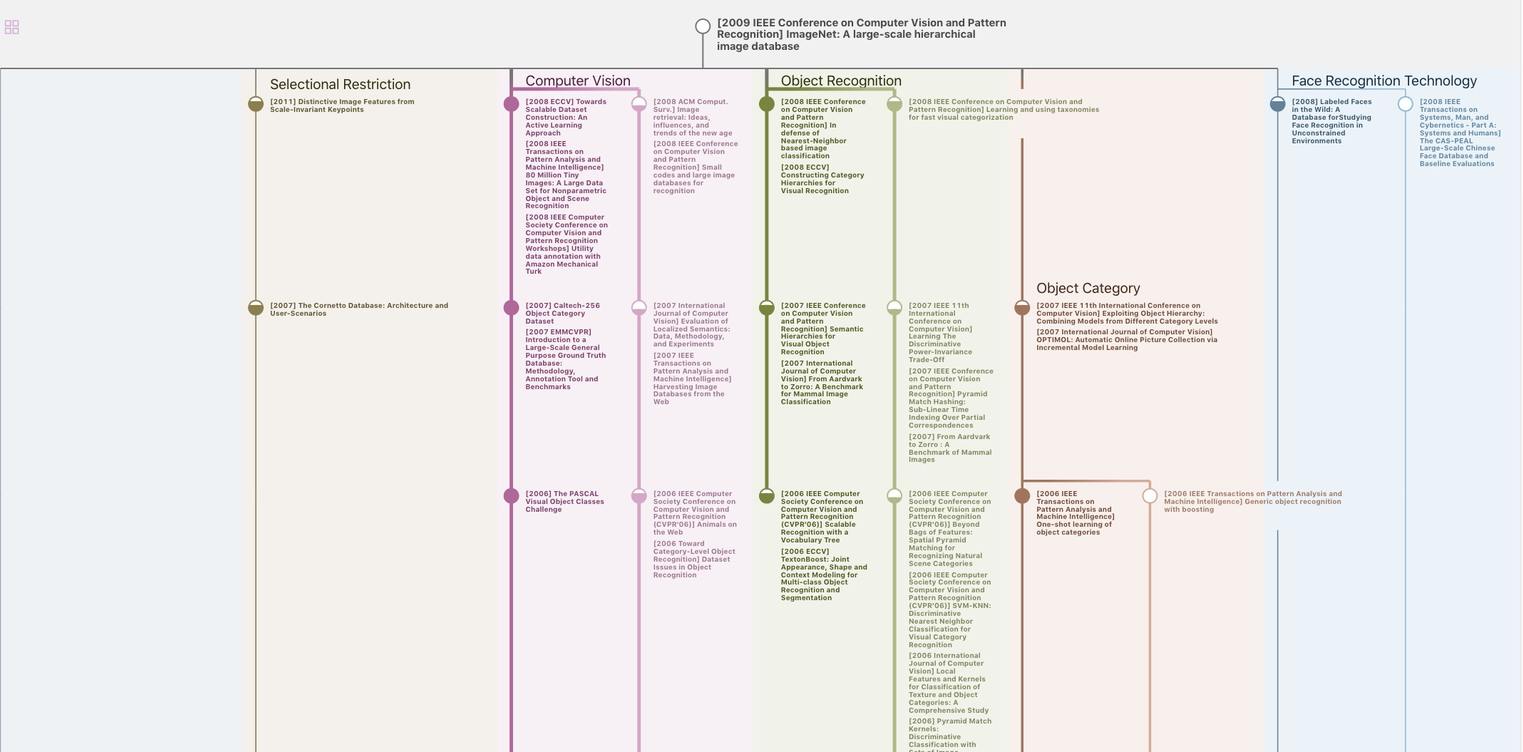
生成溯源树,研究论文发展脉络
Chat Paper
正在生成论文摘要