FS-3DSSN: an efficient few-shot learning for single-stage 3D object detection on point clouds
The Visual Computer(2024)
摘要
The current 3D object detection methods have achieved promising results for conventional tasks to detect frequently occurring objects like cars, pedestrians and cyclists. However, they require many annotated boundary boxes and class labels for training, which is very expensive and hard to obtain. Nevertheless, detecting infrequent occurring objects, such as police vehicles, is also essential for autonomous driving to be successful. Therefore, we explore the potential of few-shot learning to handle this challenge of detecting infrequent categories. The current 3D object detectors do not have the necessary architecture to support this type of learning. Thus, this paper presents a new method termed few-shot single-stage network for 3D object detection (FS-3DSSN) to predict infrequent categories of objects. FS-3DSSN uses a class-incremental few-shot learning approach to detect infrequent categories without compromising the detection accuracy of frequent categories. It consists of two modules: (i) a single-stage network architecture for 3D object detection (3DSSN) using deformable convolutions to detect small objects and (ii) a class-incremental-based meta-learning module to learn and predict infrequent class categories. 3DSSN obtained 84.53 mAP_3D on the KITTI car category and 73.4 NDS on the nuScenes dataset, outperforming previous state of the art. Further, the result of FS-3DSSN on nuScenes is also encouraging for detecting infrequent categories while maintaining accuracy in frequent classes.
更多查看译文
关键词
Point cloud,3D object detection,Few-shot learning,Single-stage detector,Autonomous driving
AI 理解论文
溯源树
样例
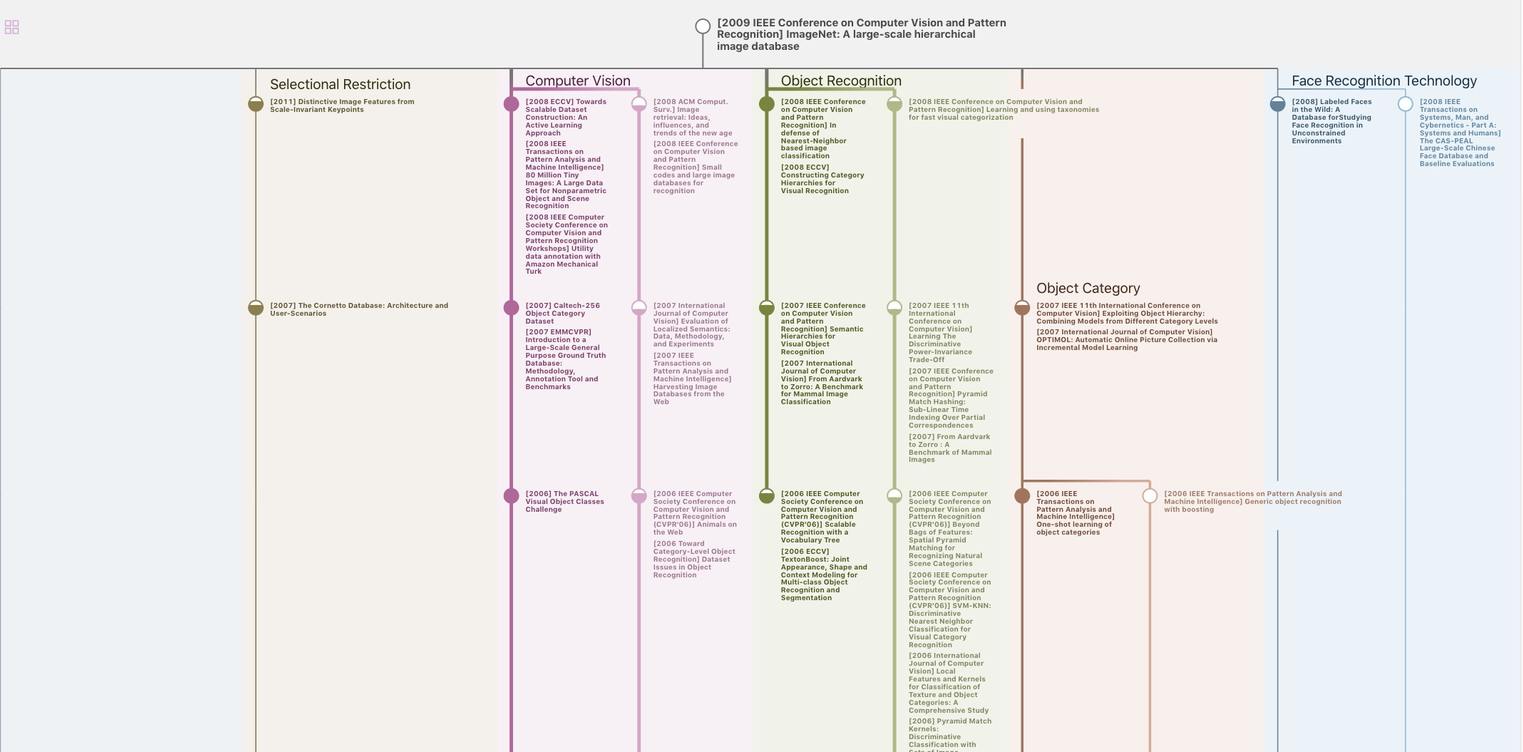
生成溯源树,研究论文发展脉络
Chat Paper
正在生成论文摘要