Preference Contrastive Learning for Personalized Recommendation
PATTERN RECOGNITION AND COMPUTER VISION, PRCV 2023, PT IX(2024)
摘要
Recommender systems play a crucial role in providing personalized services but face significant challenges from data sparsity and long-tail bias. Researchers have sought to address these issues using self-supervised contrastive learning. Current contrastive learning primarily relies on self-supervised signals to enhance embedding quality. Despite performance improvement, task-independent contrastive learning contributes limited to the recommendation task. In an effort to adapt contrastive learning to the task, we propose a preference contrastive learning (PCL) model by contrasting preferences of user-items pairs to model users' interests, instead of the self-supervised user-user/item-item discrimination. The supervised contrastive manner works in a single view of the interaction graph and does not require additional data augmentation and multi-view contrasting anymore. Performance on public datasets shows that the proposed PCL outperforms the state-of-the-art models, demonstrating that preference contrast betters self-supervised contrast for personalized recommendation.
更多查看译文
关键词
Recommender System,Contrastive Learning,Interest Propagation,Graph Convolution
AI 理解论文
溯源树
样例
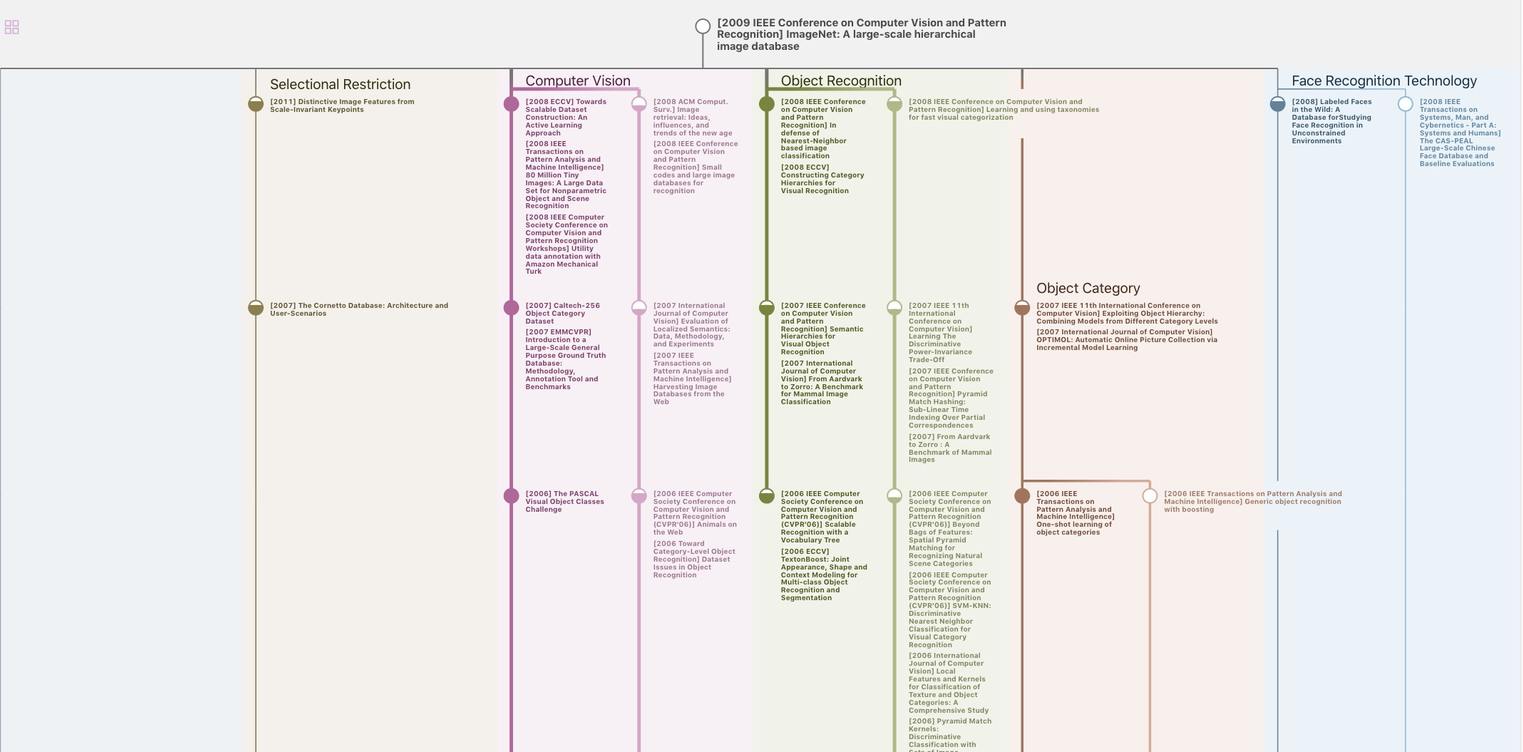
生成溯源树,研究论文发展脉络
Chat Paper
正在生成论文摘要