Tandem SERS and MS/MS Profiling of Plasma Extracellular Vesicles for Early Ovarian Cancer Biomarker Discovery
ACS sensors(2024)
摘要
Extracellular vesicles (EVs) are vectors of biomolecular cargo that play essential roles in intercellular communication across a range of cells. Protein, lipid, and nucleic acid cargo harbored within EVs may serve as biomarkers at all stages of disease; however, the choice of methodology may challenge the specificity and reproducibility of discovery. To address these challenges, the integration of rigorous EV purification methods, cutting-edge spectroscopic technologies, and data analysis are critical to uncover diagnostic signatures of disease. Herein, we demonstrate an EV isolation and analysis pipeline using surface-enhanced Raman spectroscopy (SERS) and mass spectrometry (MS) techniques on plasma samples obtained from umbilical cord blood, healthy donor (HD) plasma, and plasma from women with early stage high-grade serous carcinoma (HGSC). Plasma EVs were purified by size exclusion chromatography and analyzed by surface-enhanced Raman spectroscopy (SERS), mass spectrometry (MS), and atomic force microscopy. After determining the fraction of highest EV purity, SERS and MS were used to characterize EVs from HDs, pooled donors with noncancerous gynecological ailments (n = 6), and donors with early stage [FIGO (I/II)] with HGSC. SERS spectra were subjected to different machine learning algorithms such as PCA, logistic regression, support vector machine, nai''ve Bayes, random forest, neural network, and k nearest neighbors to differentiate healthy, benign, and HGSC EVs. Collectively, we demonstrate a reproducible workflow with the potential to serve as a diagnostic platform for HGSC.
更多查看译文
关键词
extracellular vesicles,surface-enhanced Raman spectroscopy,nanohole arrays,plasmonics,nanomaterials,machine learning
AI 理解论文
溯源树
样例
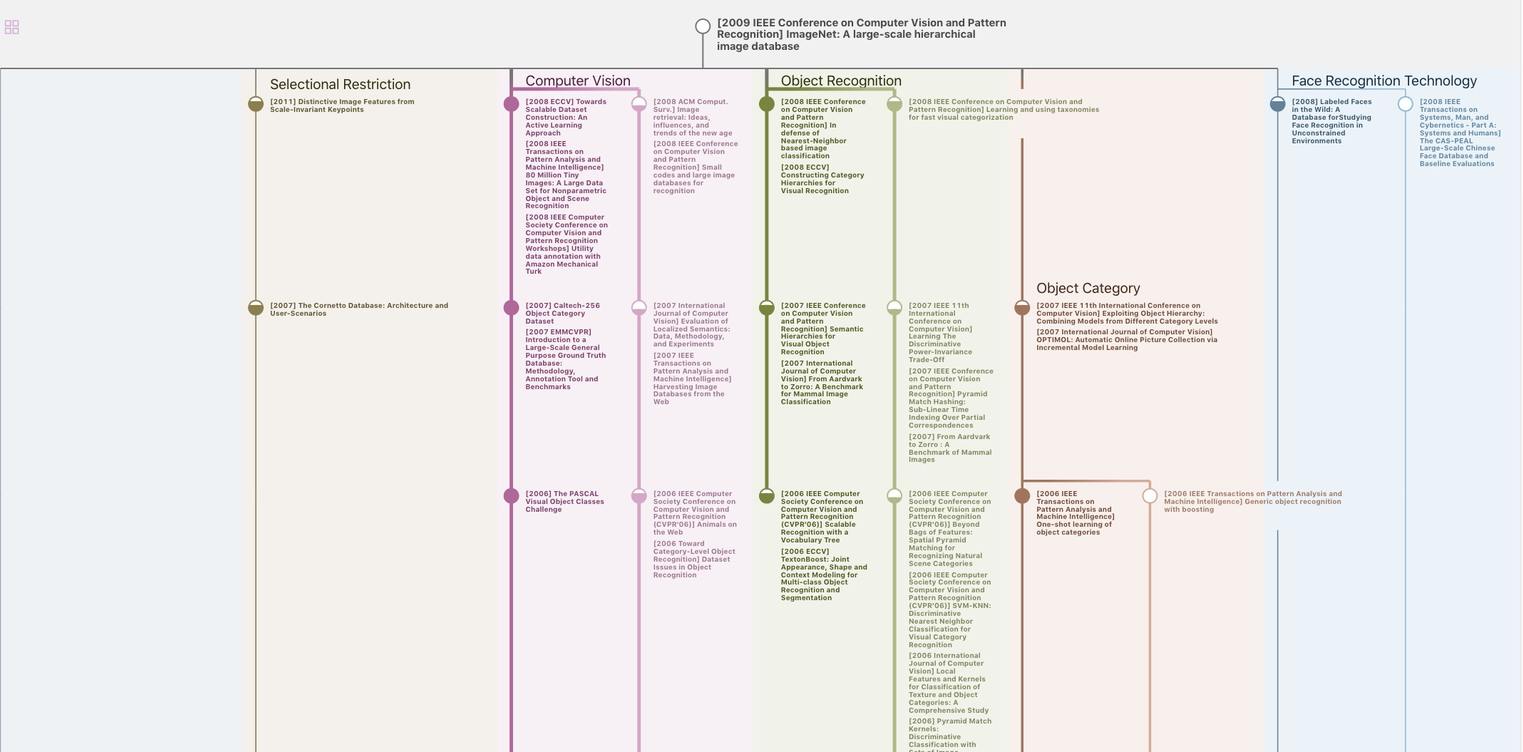
生成溯源树,研究论文发展脉络
Chat Paper
正在生成论文摘要