DockOpt: A Tool for Automatic Optimization of Docking Models.
Journal of Chemical Information and Modeling(2024)
摘要
Molecular docking is a widely used technique for leveraging protein structure for ligand discovery, but it remains difficult to utilize due to limitations that have not been adequately addressed. Despite some progress toward automation, docking still requires expert guidance, hindering its adoption by a broader range of investigators. To make docking more accessible, we developed a new utility called DockOpt, which automates the creation, evaluation, and optimization of docking models prior to their deployment in large-scale prospective screens. DockOpt outperforms our previous automated pipeline across all 43 targets in the DUDE-Z benchmark data set, and the generated models for 84% of targets demonstrate sufficient enrichment to warrant their use in prospective screens, with normalized LogAUC values of at least 15%. DockOpt is available as part of the Python package Pydock3 included in the UCSF DOCK 3.8 distribution, which is available for free to academic researchers at https://dock.compbio.ucsf.edu and free for everyone upon registration at https://tldr.docking.org.
更多查看译文
关键词
Molecular Docking
AI 理解论文
溯源树
样例
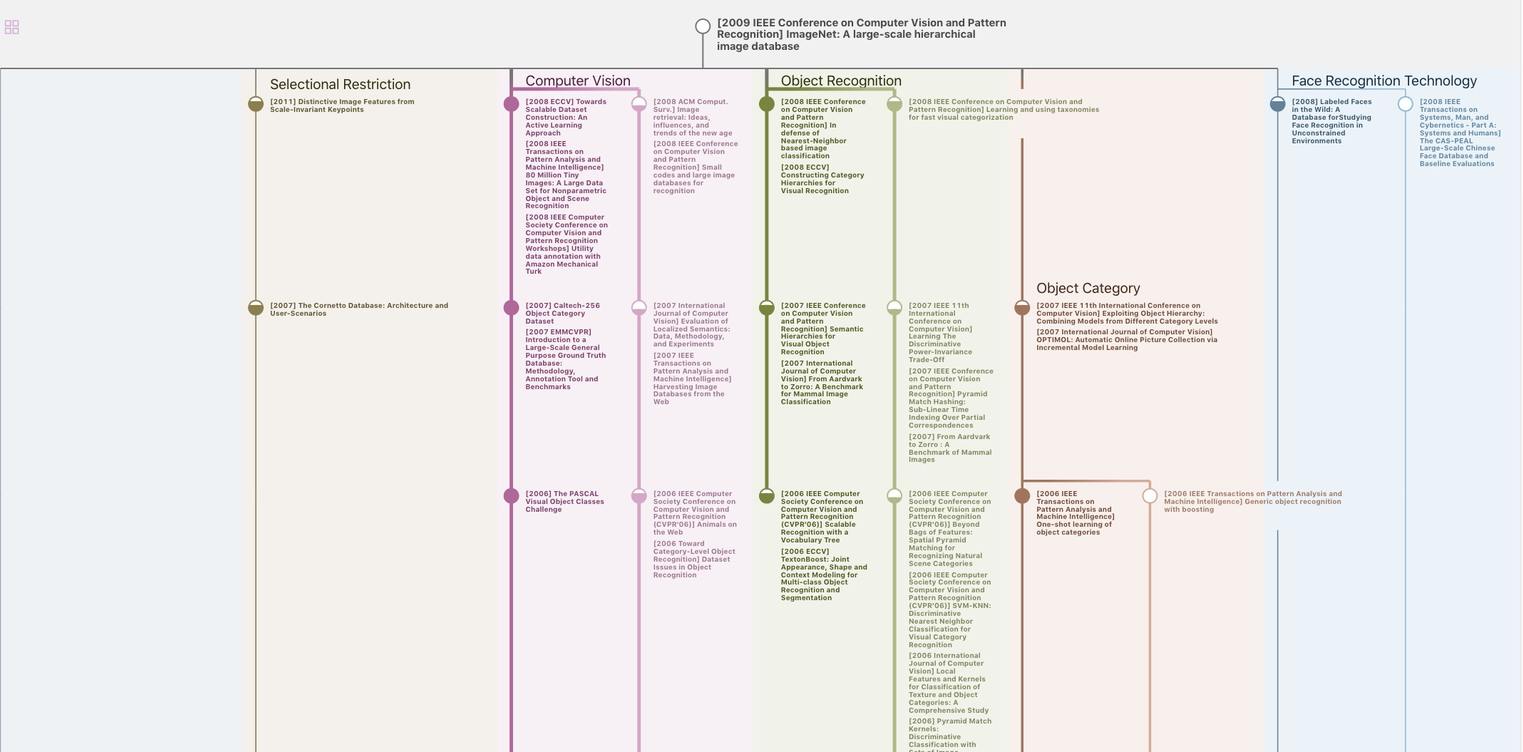
生成溯源树,研究论文发展脉络
Chat Paper
正在生成论文摘要