Functional Linear Partial Quantile Regression with Guaranteed Convergence for Neuroimaging Data Analysis
Statistics in Biosciences(2024)
Abstract
Functional data such as curves and surfaces have become more and more common with modern technological advancements. The use of functional predictors remains challenging due to its inherent infinite dimensionality. The common practice is to project functional data into a finite dimensional space. The popular partial least square method has been well studied for the functional linear model (Delaigle and Hall in Ann Stat 40(1):322–352, 2012). As an alternative, quantile regression provides a robust and more comprehensive picture of the conditional distribution of a response when it is non-normal, heavy-tailed, or contaminated by outliers. While partial quantile regression (PQR) was proposed in (Yu et al. in Neurocomputing 195:74–87, 2016)[ 2 ], no theoretical guarantees were provided due to the iterative nature of the algorithm and the non-smoothness of quantile loss function. To address these issues, we propose an alternative PQR formulation with guaranteed convergence. This novel formulation motivates new theories and allows us to establish asymptotic properties. Numerical studies on a benchmark dataset show the superiority of our new approach. We also apply our novel method to a functional magnetic resonance imaging data to predict attention deficit hyperactivity disorder and a diffusion tensor imaging dataset to predict Alzheimer’s disease.
MoreTranslated text
Key words
Functional data analysis,Functional partial least square,Functional partial quantile regression,Finite smoothing,Neuroimaging
AI Read Science
Must-Reading Tree
Example
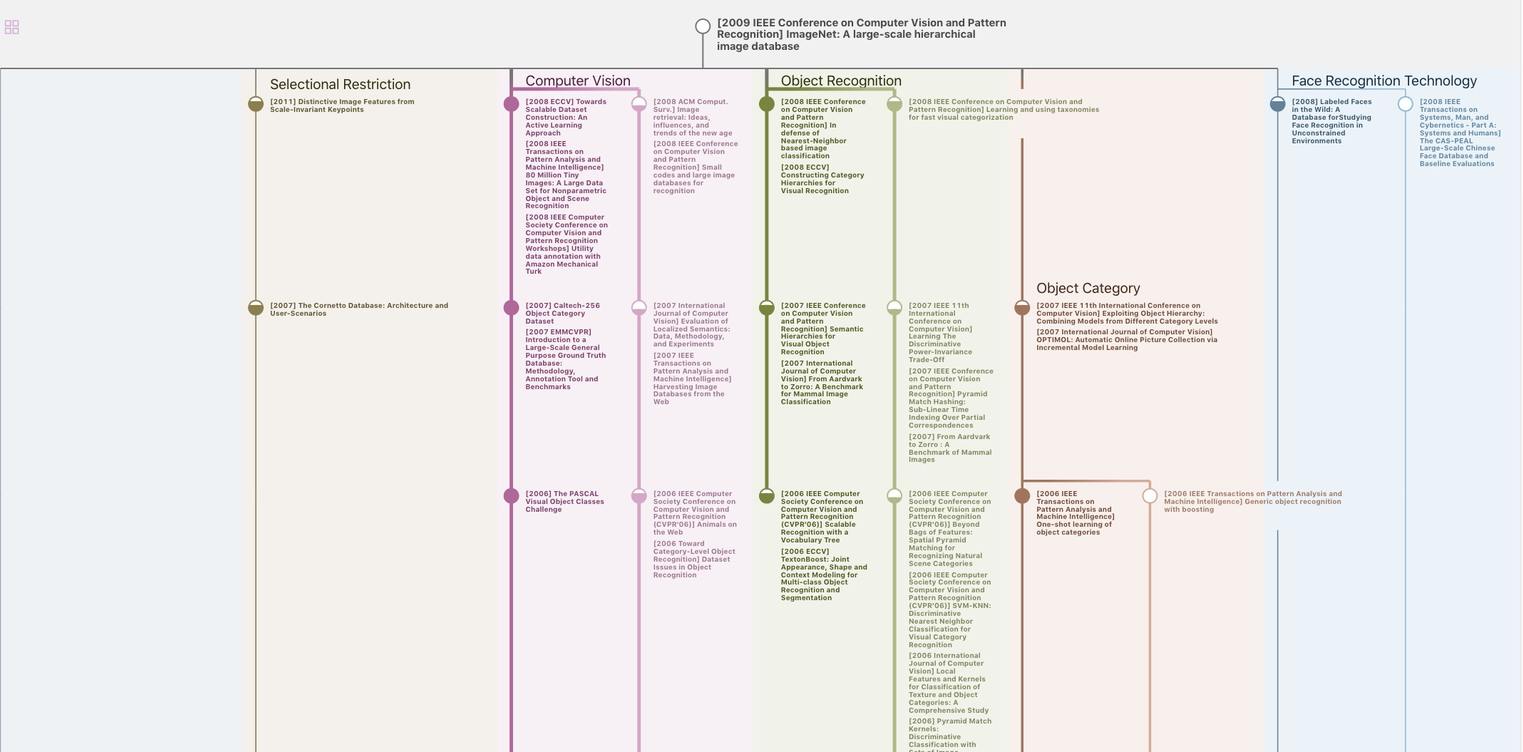
Generate MRT to find the research sequence of this paper
Chat Paper
Summary is being generated by the instructions you defined