MFEFNet: Multi-scale Feature Extraction and Fusion Network for Semantic Segmentation of Remote Sensing Images
2023 Asia Conference on Advanced Robotics, Automation, and Control Engineering (ARACE)(2023)
摘要
Achieving accurate semantic segmentation of complex scenes in remotely sensed images (RSIs) is of great significance for the interpretation of remote sensing big data. The inherent intra-class inconsistency and inter-class indistinguishability of RSIs make segmentation of different ground objects at various scales more challenging, while accurate mapping of edges for small-scale objects is also difficult. To address the above-mentioned problems, we propose a multi-scale feature extraction and fusion network, named MFEFNet, which uses adaptive spatial attention mechanism to capture spatial contextual information. The proposed network contains two modules, the single-level feature attention module (SFA-M) and multi-level feature fusion module (MFF-M). Specifically, SFA-M improves segmentation accuracy by obtaining multi-scale feature maps through the successive averaging pooling operation and adaptively aggregating multiscale information from coarse to fine, which can mine deeper spatial contextual information effectively. Besides, the MFF-M uses aligned convolution operations to make full use of the multi-level features, which have semantic gaps. The experiments and ablation studies have been conducted on the ISPRS Potsdam dataset. Quantitative analysis and visualizations show that MEF-Net can have good semantic segmentation performance. Numerically, compared to the mainstream model (SPANet,2022), MFEFNet (ResNet-50) improved by 1.68%, 0.9% and 2.02% in terms of mF1, OA and mIoU, respectively.
更多查看译文
关键词
attention mechanism,multi-level feature fusion,Convolutional neural network,remote sensing images,adaptive spatial attention
AI 理解论文
溯源树
样例
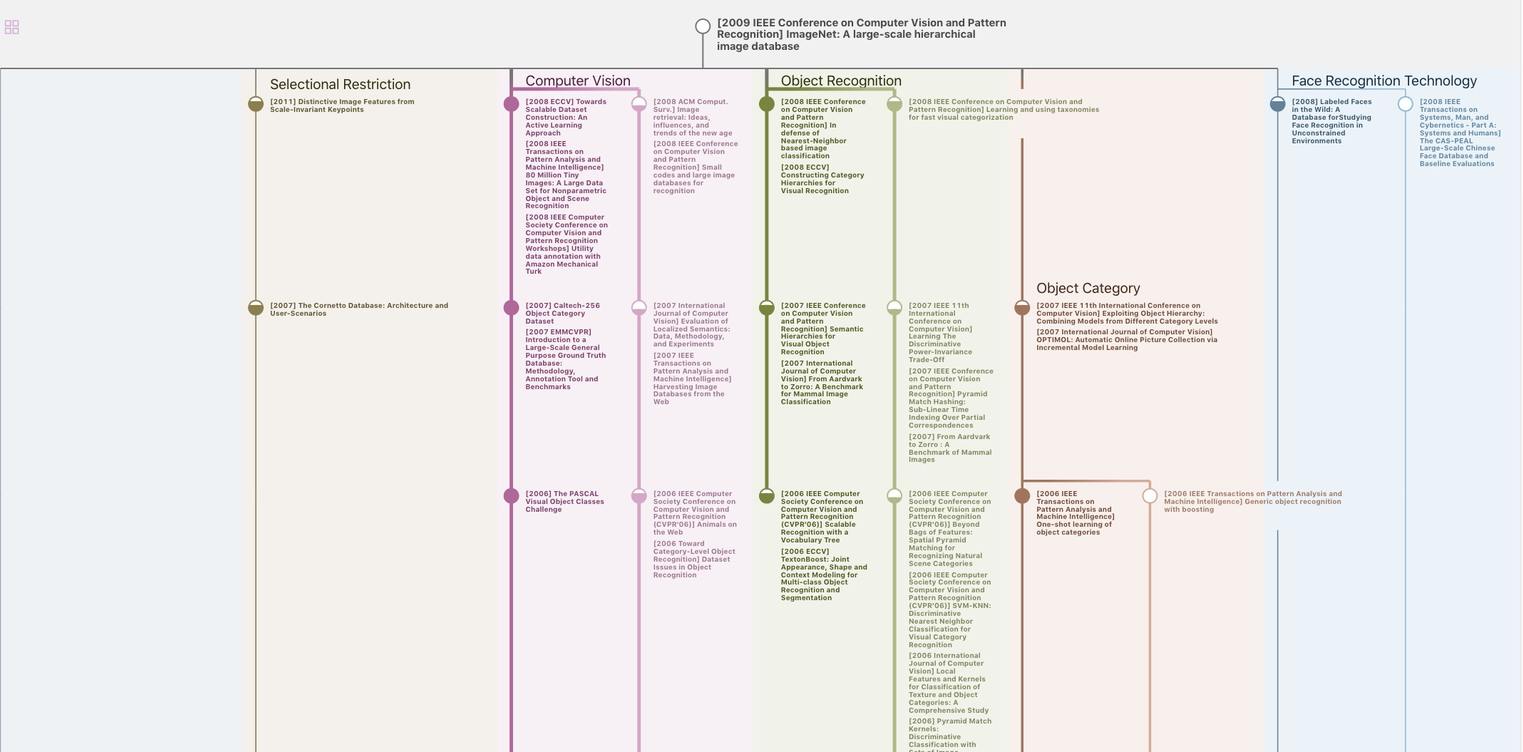
生成溯源树,研究论文发展脉络
Chat Paper
正在生成论文摘要